Interpreting Reward Models in RLHF-Tuned Language Models Using Sparse Autoencoders
CoRR(2023)
摘要
Large language models (LLMs) aligned to human preferences via reinforcement learning from human feedback (RLHF) underpin many commercial applications. However, how RLHF impacts LLM internals remains opaque. We propose a novel method to interpret learned reward functions in RLHF-tuned LLMs using sparse autoencoders. Our approach trains autoencoder sets on activations from a base LLM and its RLHF-tuned version. By comparing autoencoder hidden spaces, we identify unique features that reflect the accuracy of the learned reward model. To quantify this, we construct a scenario where the tuned LLM learns token-reward mappings to maximize reward. This is the first application of sparse autoencoders for interpreting learned rewards and broadly inspecting reward learning in LLMs. Our method provides an abstract approximation of reward integrity. This presents a promising technique for ensuring alignment between specified objectives and model behaviors.
更多查看译文
关键词
interpreting reward models,language models,autoencoders,rlhf-tuned
AI 理解论文
溯源树
样例
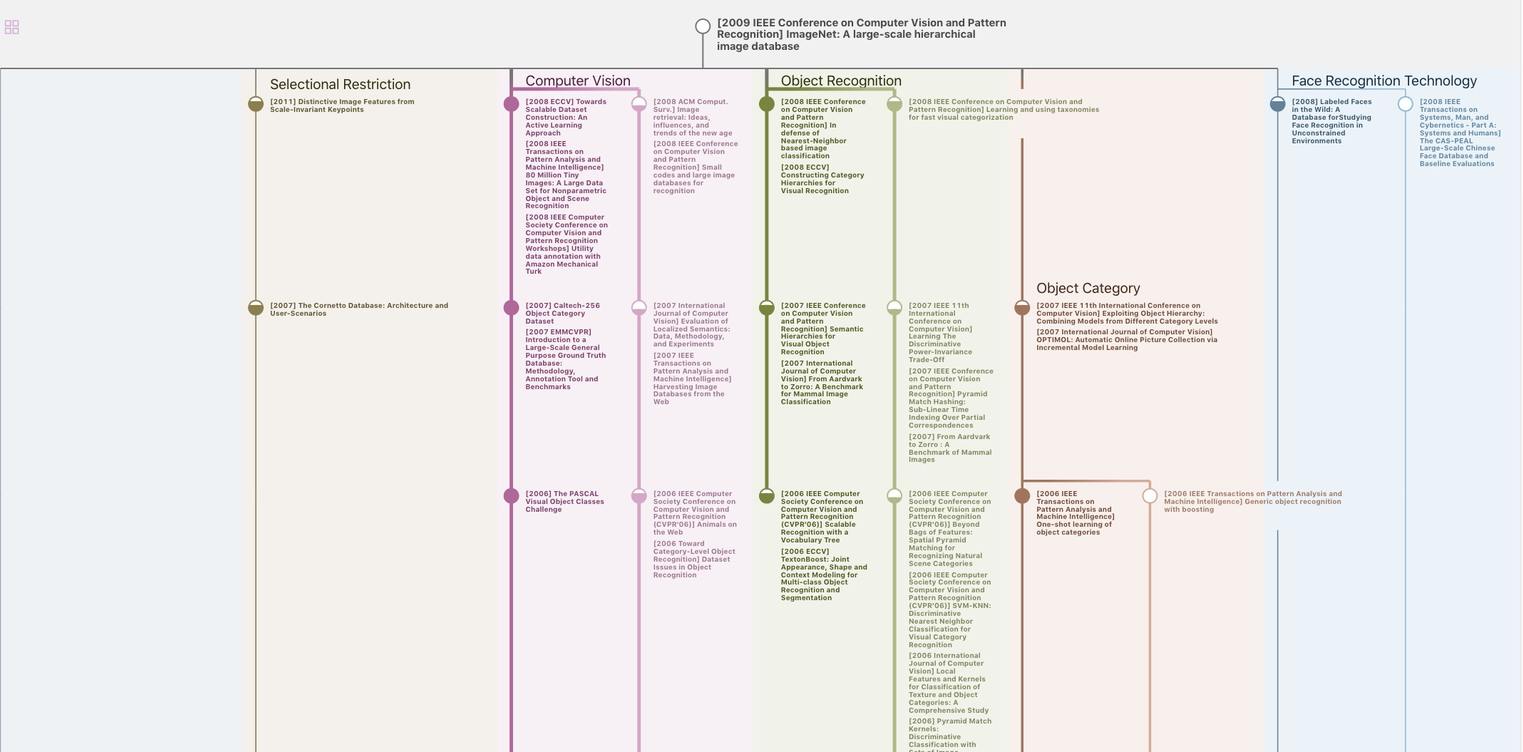
生成溯源树,研究论文发展脉络
Chat Paper
正在生成论文摘要