Neural network approach to quasiparticle dispersions in doped antiferromagnets
arXiv (Cornell University)(2023)
摘要
Numerically simulating spinful, fermionic systems is of great interest from the perspective of condensed matter physics. However, the exponential growth of the Hilbert space dimension with system size renders an exact parameterization of large quantum systems prohibitively demanding. This is a perfect playground for neural networks, owing to their immense representative power that often allows to use only a fraction of the parameters that are needed in exact methods. Here, we investigate the ability of neural quantum states (NQS) to represent the bosonic and fermionic $t-J$ model - the high interaction limit of the Fermi-Hubbard model - on different 1D and 2D lattices. Using autoregressive recurrent neural networks (RNNs) with 2D tensorized gated recurrent units, we study the ground state representations upon doping the half-filled system with holes. Moreover, we present a method to calculate dispersion relations from the neural network state representation, applicable to any neural network architecture and any lattice geometry, that allows to infer the low-energy physics from the NQS. To demonstrate our approach, we calculate the dispersion of a single hole in the $t-J$ model on different 1D and 2D square and triangular lattices. Furthermore, we analyze the strengths and weaknesses of the RNN approach for fermionic systems, pointing the way for an accurate and efficient parameterization of fermionic quantum systems using neural quantum states.
更多查看译文
关键词
antiferromagnets,dispersions,neural network approach
AI 理解论文
溯源树
样例
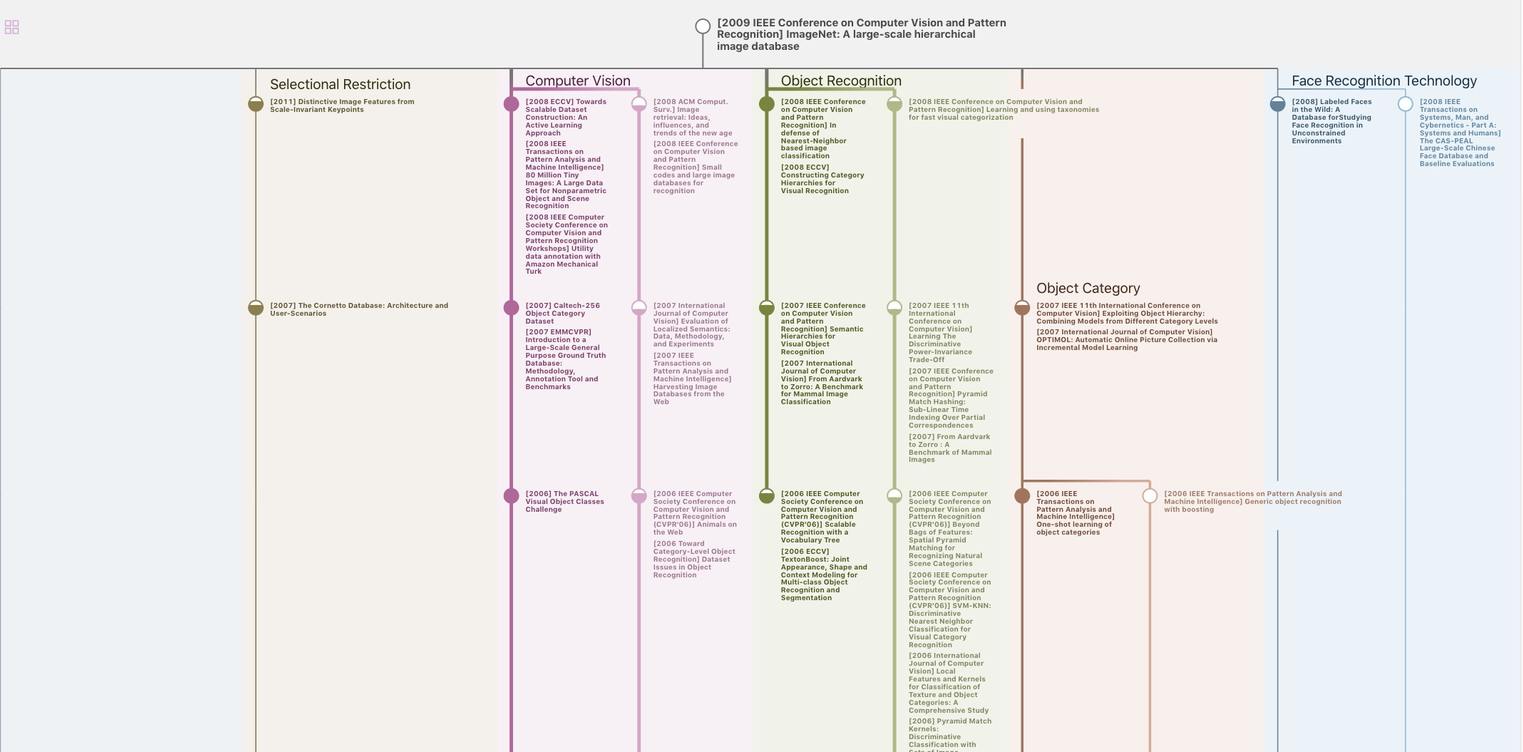
生成溯源树,研究论文发展脉络
Chat Paper
正在生成论文摘要