LEA2: A Lightweight Ensemble Adversarial Attack via Non-overlapping Vulnerable Frequency Regions
2023 IEEE/CVF International Conference on Computer Vision (ICCV)(2023)
摘要
Recent work shows that well-designed adversarial examples can fool deep neural networks (DNNs). Due to their transferability, adversarial examples can also attack target models without extra information, called black-box attacks. However, most existing ensemble attacks depend on numerous substitute models to cover the vulnerable subspace of a target model. In this work, we find three types of models with non-overlapping vulnerable frequency regions, which can cover a large enough vulnerable subspace. Based on this finding, we propose a lightweight ensemble adversarial attack named LEA
2
, integrated by standard, weakly robust, and robust models. Moreover, we analyze Gaussian noise from the perspective of frequency and find that Gaussian noise is located in the vulnerable frequency regions of standard models. Therefore, we substitute standard models with Gaussian noise to ensure the use of high-frequency vulnerable regions while reducing attack time consumption. Experiments on several image datasets indicate that LEA
2
achieves better transferability under different defended models compared with extensive baselines and state-of-the-art attacks.
更多查看译文
AI 理解论文
溯源树
样例
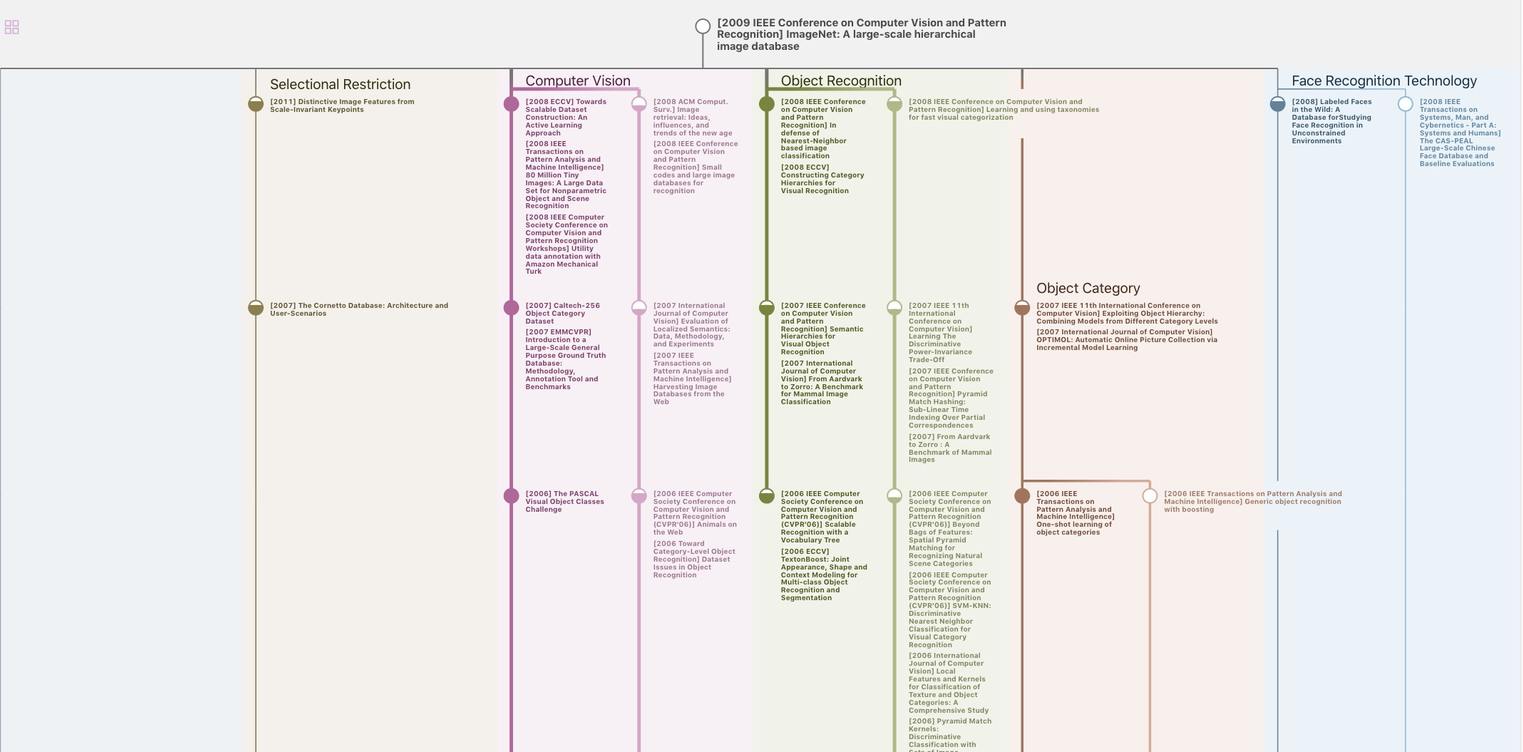
生成溯源树,研究论文发展脉络
Chat Paper
正在生成论文摘要