SIRA-PCR: Sim-to-Real Adaptation for 3D Point Cloud Registration.
ICCV(2023)
摘要
Point cloud registration is essential for many applications. However, existing real datasets require extremely tedious and costly annotations, yet may not provide accurate camera poses. For the synthetic datasets, they are mainly object-level, so the trained models may not generalize well to real scenes. We design SIRA-PCR, a new approach to 3D point cloud registration. First, we build a synthetic scene-level 3D registration dataset, specifically designed with physically-based and random strategies to arrange diverse objects. Second, we account for variations in different sensing mechanisms and layout placements, then formulate a sim-to-real adaptation framework with an adaptive re-sample module to simulate patterns in real point clouds. To our best knowledge, this is the first work that explores sim-to-real adaptation for point cloud registration. Extensive experiments show the SOTA performance of SIRA-PCR on widely-used indoor and out-door datasets. The code and dataset will be released on https://github.com/Chen-Suyi/SIRA_Pytorc.h
更多查看译文
AI 理解论文
溯源树
样例
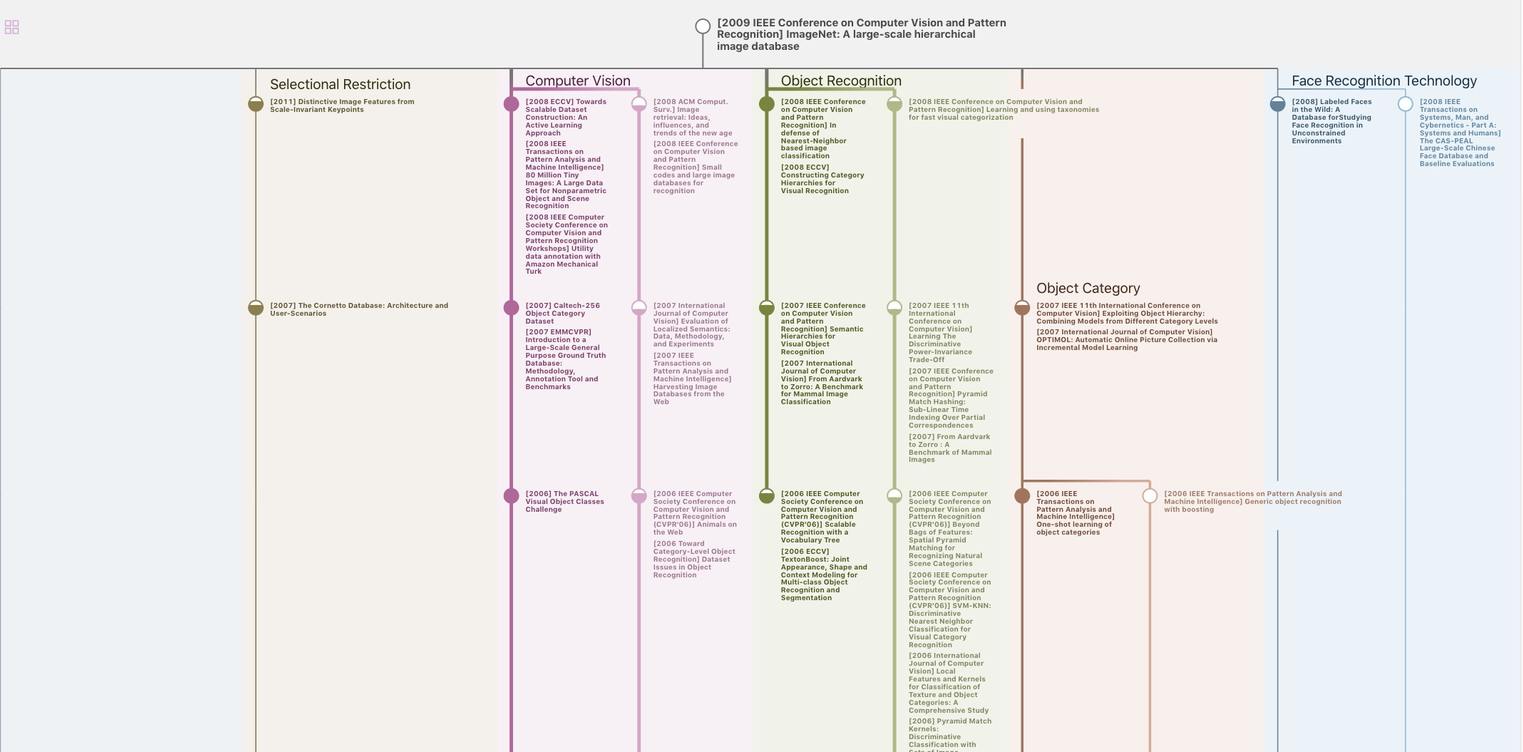
生成溯源树,研究论文发展脉络
Chat Paper
正在生成论文摘要