Scene-Aware Label Graph Learning for Multi-Label Image Classification.
ICCV(2023)
摘要
Multi-label image classification refers to assigning a set of labels for an image. One of the main challenges of this task is how to effectively capture the correlation among labels. Existing studies on this issue mostly rely on the statistical label co-occurrence or semantic similarity of labels. However, an important fact is ignored that the co-occurrence of labels is closely related with image scenes (indoor, outdoor, etc.), which is a vital characteristic in multi-label image classification. In this paper, a novel scene-aware label graph learning framework is proposed, which is capable of learning visual representations for labels while fully perceiving their co-occurrence relationships under variable scenes. Specifically, our framework is able to detect scene categories of images without relying on manual annotations, and keeps track of the co-occurring labels by maintaining a global co-occurrence matrix for each scene category throughout the whole training phase. These scene-independent co-occurrence matrices are further employed to guide the interactions among label representations in a graph propagation manner towards accurate label prediction. Extensive experiments on public benchmarks demonstrate the superiority of our framework.
更多查看译文
AI 理解论文
溯源树
样例
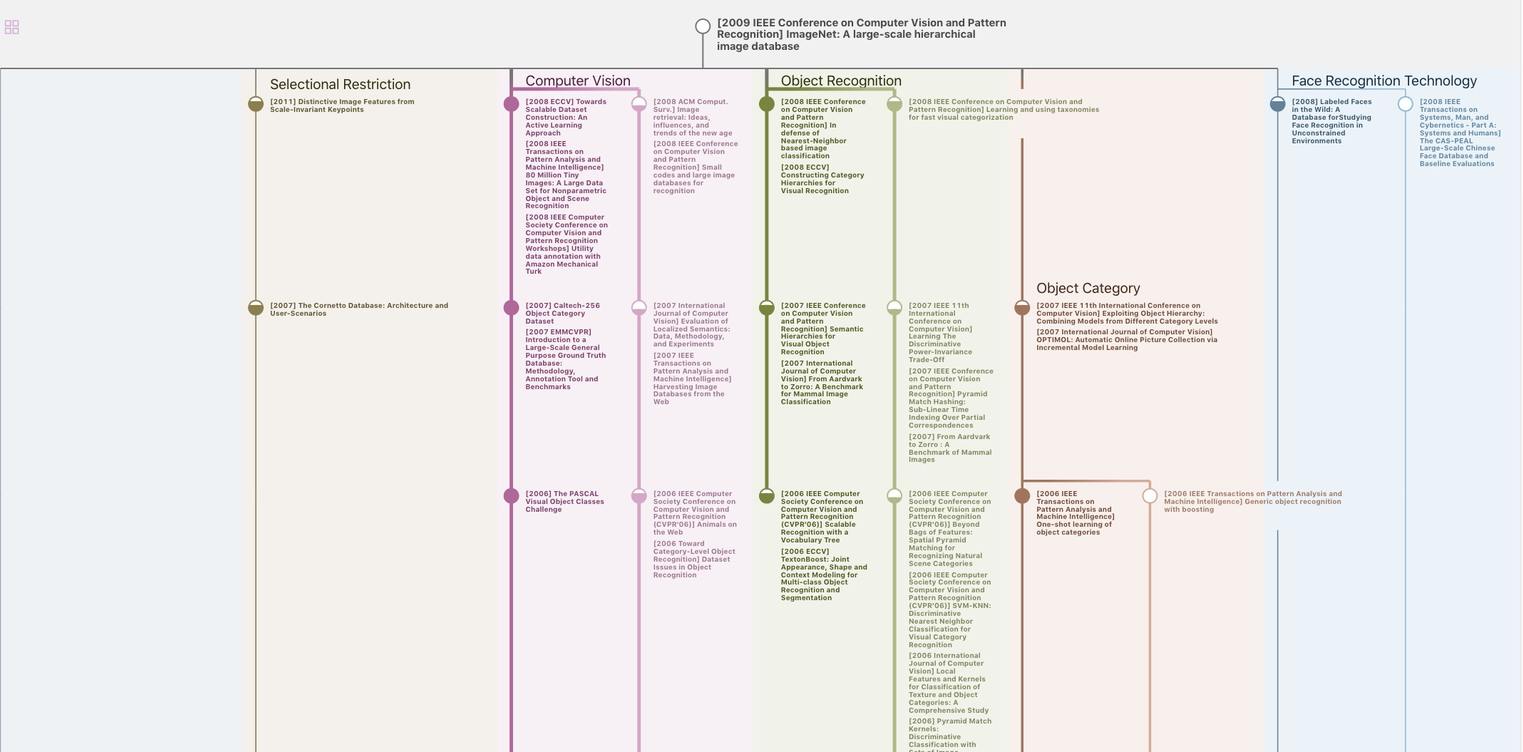
生成溯源树,研究论文发展脉络
Chat Paper
正在生成论文摘要