Global Balanced Experts for Federated Long-Tailed Learning.
ICCV(2023)
摘要
Federated learning (FL) is a prevalent distributed machine learning approach that enables collaborative training of a global model across multiple devices without sharing local data. However, the presence of long-tailed data can negatively deteriorate the model’s performance in real-world FL applications. Moreover, existing re-balance strategies are less effective for the federated long-tailed issue when directly utilizing local label distribution as the class prior at the clients’ side. To this end, we propose a novel Global Balanced Multi-Expert (GBME) framework to optimize a balanced global objective, which does not require additional information beyond the standard FL pipeline. In particular, a proxy is derived from the accumulated gradients uploaded by the clients after local training, and is shared by all clients as the class prior for re-balance training. Such a proxy can also guide the client grouping to train a multi-expert model, where the knowledge from different clients can be aggregated via the ensemble of different experts corresponding to different client groups. To further strengthen the privacy-preserving ability, we present a GBME-p algorithm with a theoretical guarantee to prevent privacy leakage from the proxy. Extensive experiments on long-tailed decentralized datasets demonstrate the effectiveness of GBME and GBME-p, both of which show superior performance to state-of-the-art methods. The code is available at here.
更多查看译文
AI 理解论文
溯源树
样例
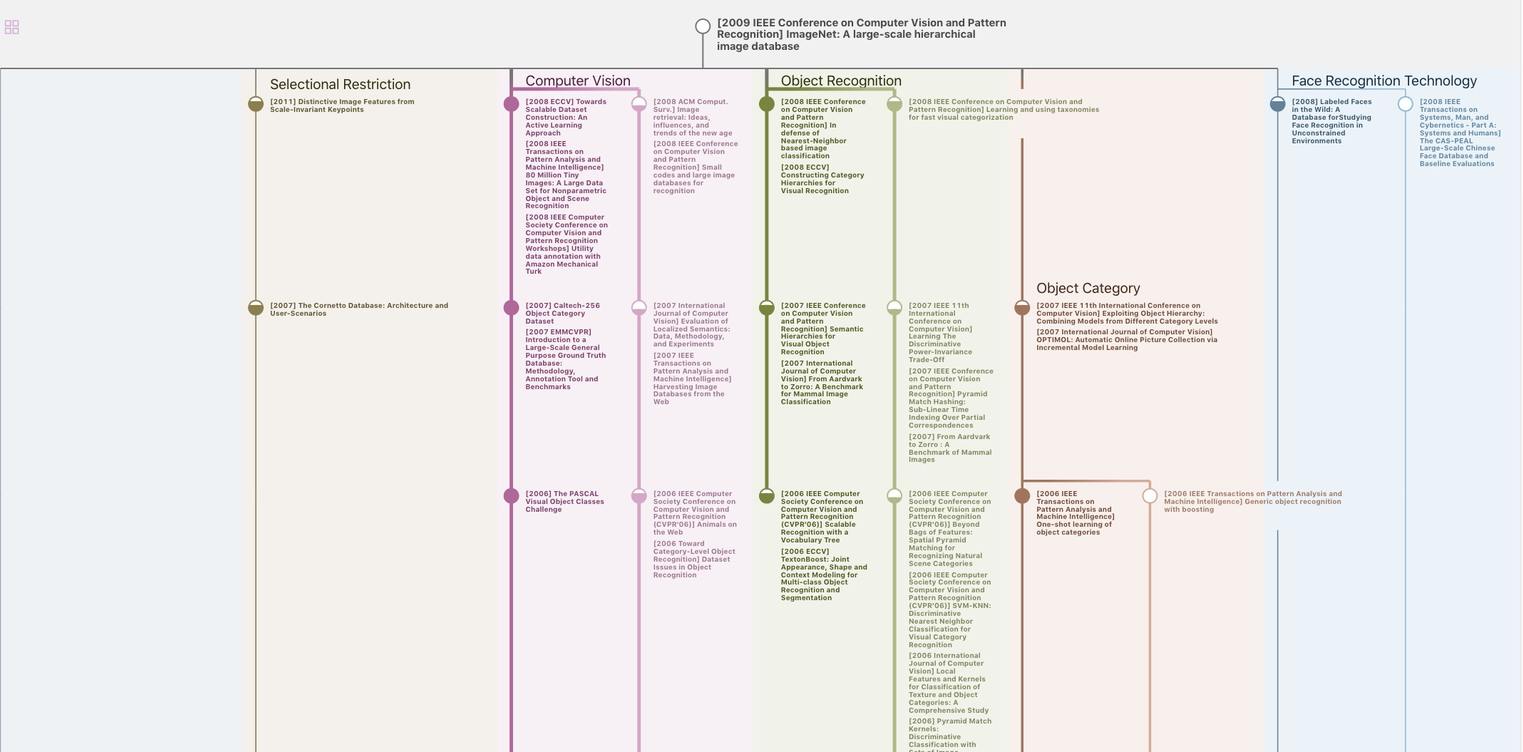
生成溯源树,研究论文发展脉络
Chat Paper
正在生成论文摘要