Towards Inadequately Pre-trained Models in Transfer Learning.
ICCV(2023)
摘要
Transfer learning has been a popular learning paradigm in the deep learning era, especially in annotation-insufficient scenarios. Better ImageNet pre-trained models have been demonstrated, from the perspective of architecture, by previous research to have better transferability to downstream tasks[26]. However, in this paper, we find that during the same pre-training process, models at middle epochs, which are inadequately pre-trained, can outperform fully trained models when used as feature extractors (FE), while the fine-tuning (FT) performance still grows with the source performance. This reveals that there is not a solid positive correlation between top-1 accuracy on ImageNet and the transferring result on target data. Based on the contradictory phenomenon between FE and FT that a better feature extractor fails to be fine-tuned better accordingly, we conduct comprehensive analyses on features before the softmax layer to provide insightful explanations. Our discoveries suggest that, during pre-training, models tend to first learn spectral components corresponding to large singular values and the residual components contribute more when fine-tuning.
更多查看译文
AI 理解论文
溯源树
样例
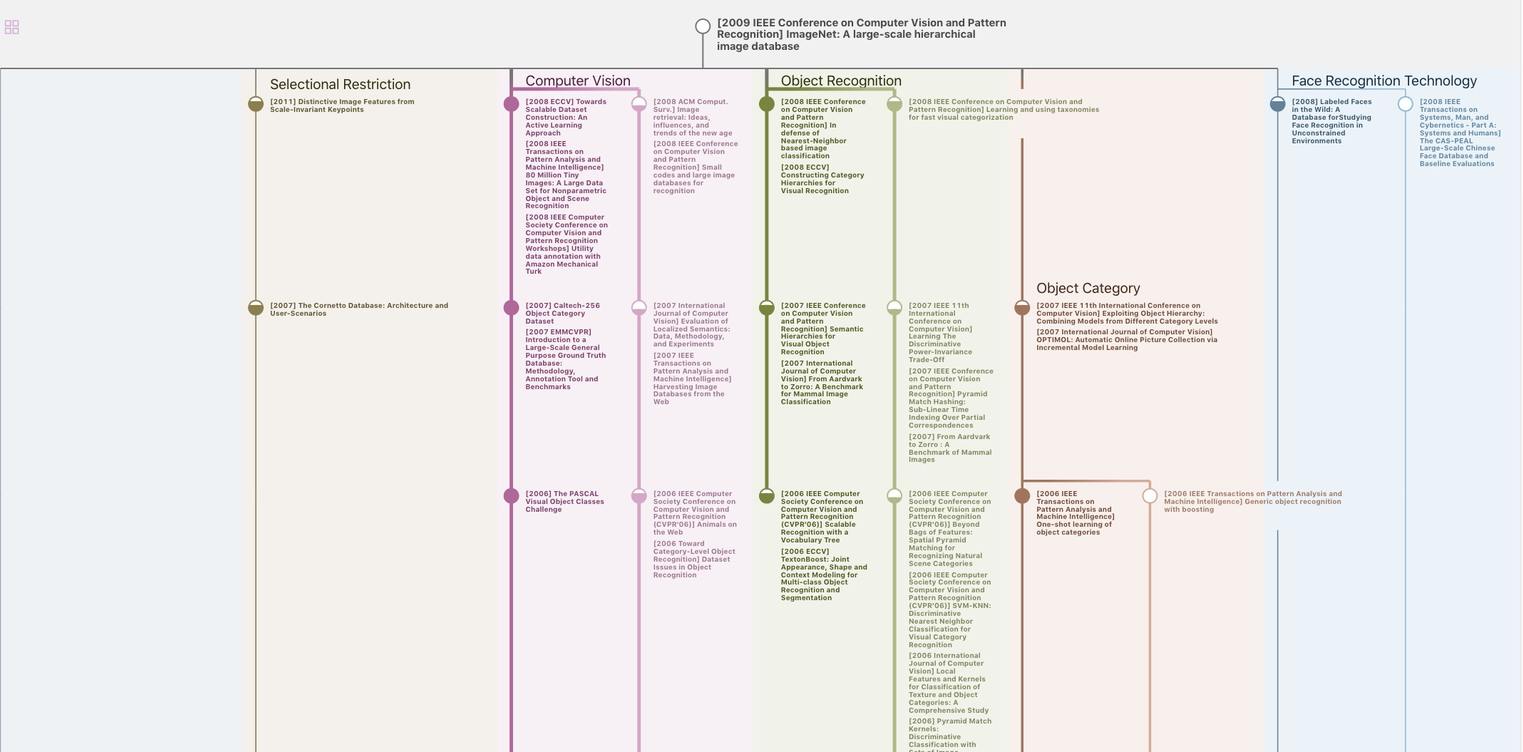
生成溯源树,研究论文发展脉络
Chat Paper
正在生成论文摘要