Bayesian Optimization-guided Discovery of High-performance Methane Combustion Catalysts based on Multi-component PtPd@CeZrOx Core-Shell Nanospheres
ANGEWANDTE CHEMIE-INTERNATIONAL EDITION(2023)
摘要
Formula regulation of multi-component catalysts by manual search is undoubtedly a time-consuming task, which has severely impeded the development efficiency of high-performance catalysts. In this work, PtPd@CeZrOx core-shell nanospheres, as a successful case study, is explicitly demonstrated how Bayesian optimization (BO) accelerates the discovery of methane combustion catalysts with the optimal formula ratio (the Pt/Pd mole ratio ranges from 1/2.33-1/9.09, and Ce/Zr from 1/0.22-1/0.35), which directly results in a lower conversion temperature (T50 approaching to 330 degrees C) than ones reported hitherto. Consequently, the best sample obtained could be efficiently developed after two rounds of iterations, containing only 18 experiments in all that is far less than the common human workload via the traditional trial-and-error search for optimal compositions. Further, this BO-based machine learning strategy can be straightforward extended to serve the autonomous discovery in multi-component material systems, for other desired properties, showing promising opportunities to practical applications in future. Bayesian optimization has been successfully employed to accelerate discovery of the high-performance multi-component PtPd@CeZrOx core-shell nanospheres for catalytic CH4 combustion. The target T50 could reach a minimum after only two iterations, and the workload can be greatly reduced from thousands to as less as 18 experiments.+image
更多查看译文
关键词
bayesian optimization‐guided,catalysts
AI 理解论文
溯源树
样例
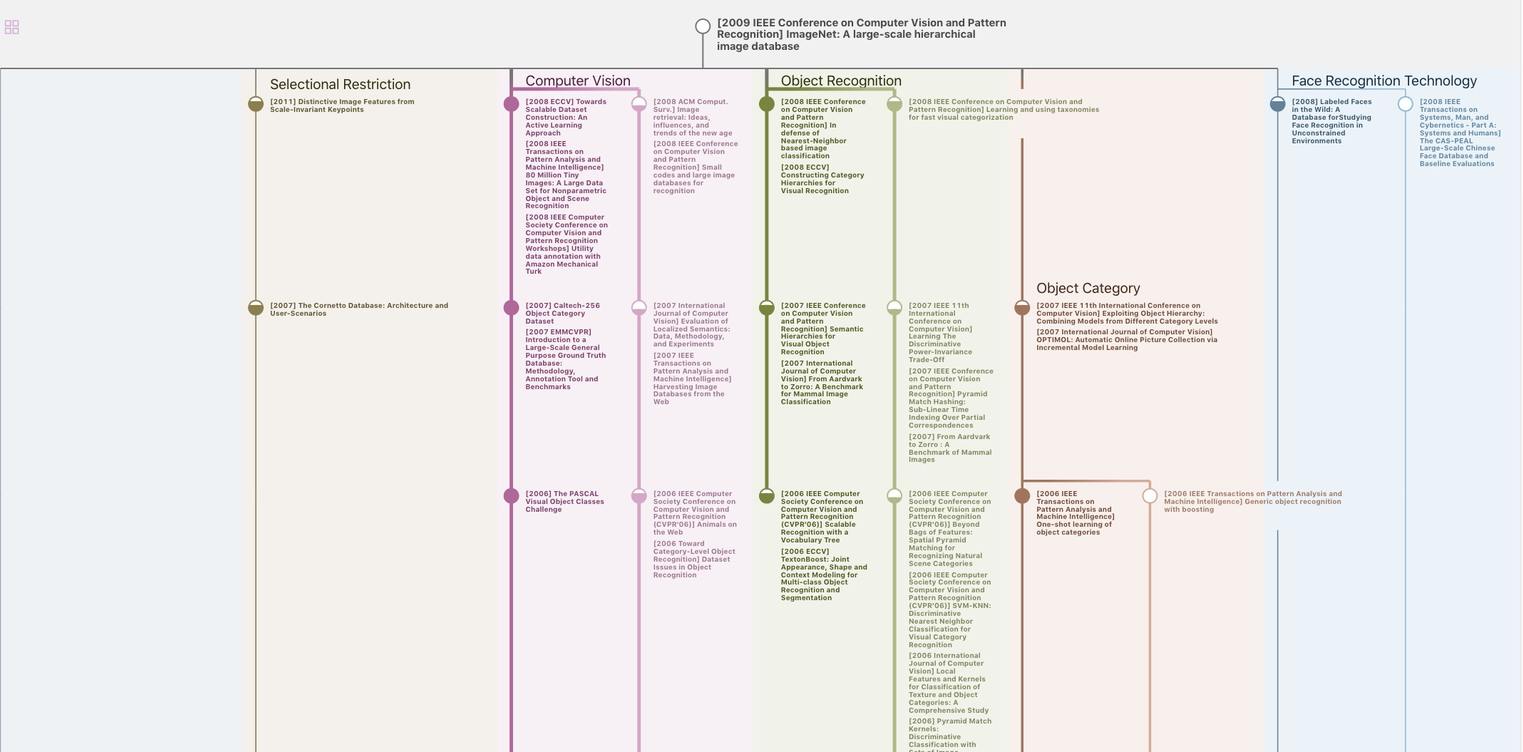
生成溯源树,研究论文发展脉络
Chat Paper
正在生成论文摘要