Domain Adaptation Using Class-Balanced Self-Paced Learning for Soil Classification With LIBS
IEEE Transactions on Plasma Science(2023)
摘要
Laser-induced breakdown spectroscopy (LIBS) is a promising technology for soil analysis due to its simple setup, cost-effectiveness, and rapid (few seconds) analysis time per sample. The recent rise in machine learning (ML) techniques for processing LIBS spectra has made LIBS more attractive. However, because of the soil samples’ varied physical properties and chemical composition, the emission lines’ distribution can be highly variable. It may cause spectra distribution change and make the training spectra not representative of the test spectra. Hence, applying an ML model trained with only samples from the training distribution to the test distribution will likely experience performance degradation. To solve the spectra distribution problem, we propose using self-learning, a domain adaptation (DA) method to self-adapt to the domain shift. It involves an iterative process of predicting on the target domain with the model trained by the source domain and then taking the confident predictions as pseudolabels for co-training the model. On top of self-learning, we also propose a novel class-balanced self-paced learning method. It balances the classes in the co-training process by ignoring the easy classes, which has a large predictive proportion to avoid the gradual dominance of these classes in pseudolabel generation. Instead of using universal selection proportion and in addition to achieve various confidence thresholds for classes, the proposed method balances and self-paces the other classes by customizing the class selection proportion and increment to avoid model bias in the self-training process. The class selection proportion and addition are tuned by validation, in which the validation set is generated by decision fusion of convolutional neural networks and partial least-squares discriminant analysis. Our method is tested with the Euro-Mediterranean symposium on LIBS (EMSLIBS) dataset, which shows the proposed method can effectively handle the spectra distribution change and achieves 90.2% test accuracy. It is comparable to the EMSLIBS contest winners with the same dataset, which uses test data calibration.
更多查看译文
关键词
Distribution change,domain adaptation (DA),laser-induced breakdown spectroscopy (LIBS),Self-learning
AI 理解论文
溯源树
样例
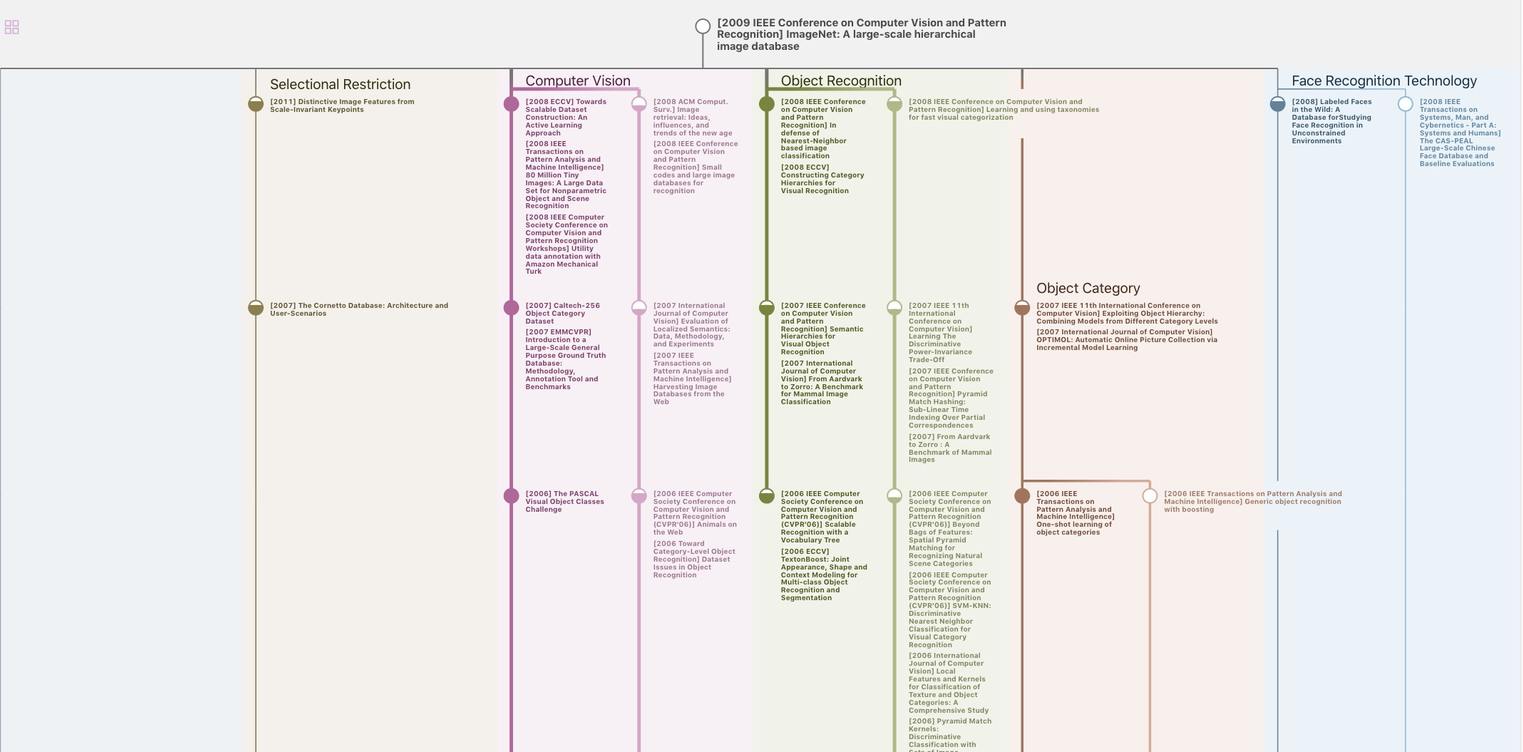
生成溯源树,研究论文发展脉络
Chat Paper
正在生成论文摘要