A Novel Architecture for Robust and Adaptive Machine Learning Using Heterogeneous Data in Condition Monitoring of Automation Systems
2023 IEEE 28th International Conference on Emerging Technologies and Factory Automation (ETFA)(2023)
摘要
Machine learning implementations in an industrial setting poses various challenges due to the heterogeneous nature of the data sources. A classical machine learning algorithm cannot adapt to dynamic changes in the environment, such as the addition, removal, or failure of a data source. However, to handle heterogeneous data and the challenges coming with this, it is a mandatory capability to build robust and adaptive machine learning models for industrial applications. In this work, a novel architecture for robust and adaptive machine learning is proposed to address these challenges. For this, an architecture consisting of different modular layers is developed, where different models can be easily plugged in. The architecture can handle heterogeneous data with different fusion techniques, which are discussed and evaluated in this paper. The proposed architecture is then evaluated on two public datasets for condition monitoring of automation systems to prove its robustness and adaptiveness. The architecture is compared with baseline models and shows more robust performance in case of failing/removed data sources. In addition, new data sources can easily be added without the need to retrain the whole model. Furthermore, the architecture can detect and locate faulty data sources.
更多查看译文
关键词
Machine Learning,Robust and Dynamic Machine Learning,Automation Systems,Data Fusion
AI 理解论文
溯源树
样例
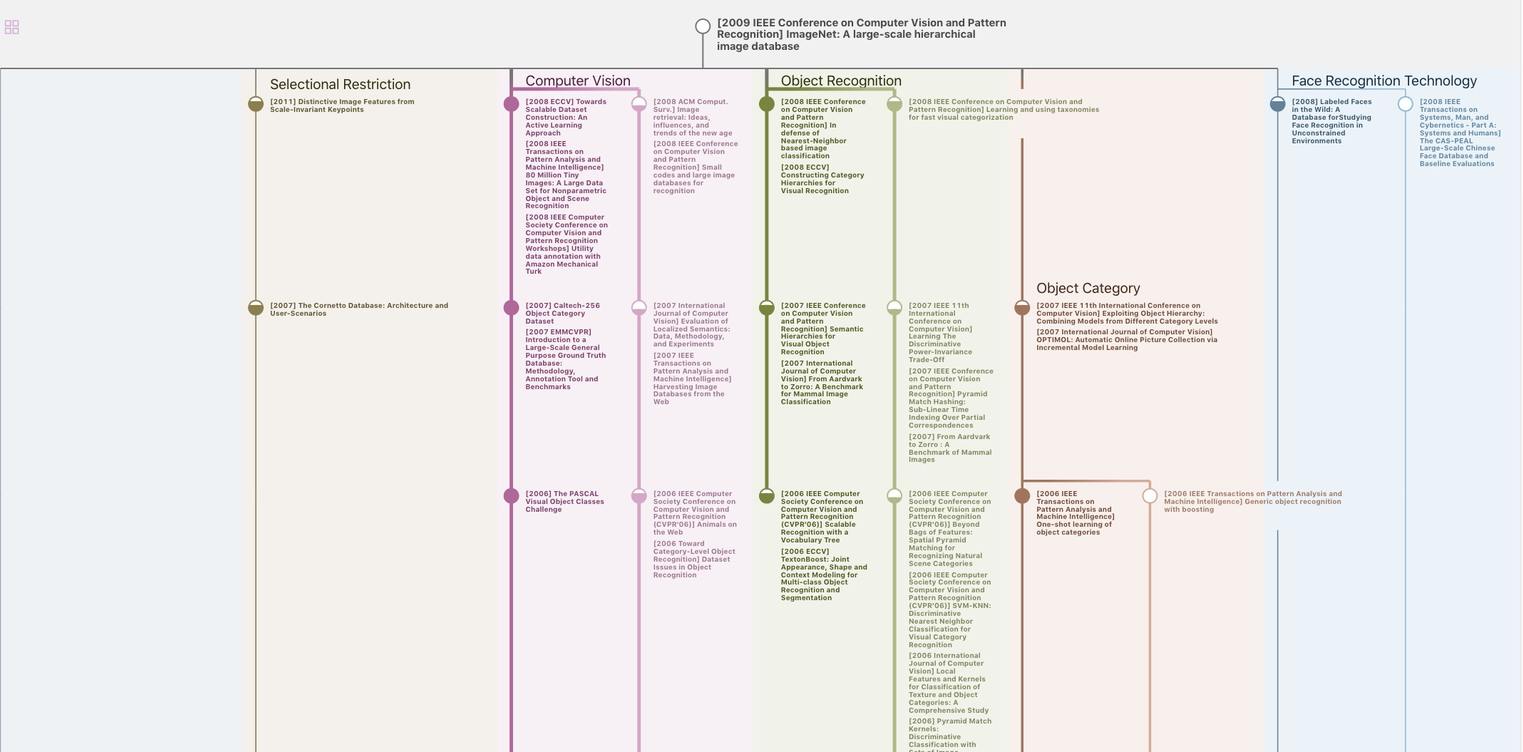
生成溯源树,研究论文发展脉络
Chat Paper
正在生成论文摘要