A machine learning model for diagnosing acute pulmonary embolism and comparison with Wells score, revised Geneva score, and Years algorithm
CHINESE MEDICAL JOURNAL(2024)
摘要
Background:Acute pulmonary embolism (APE) is a fatal cardiovascular disease, yet missed diagnosis and misdiagnosis often occur due to non-specific symptoms and signs. A simple, objective technique will help clinicians make a quick and precise diagnosis. In population studies, machine learning (ML) plays a critical role in characterizing cardiovascular risks, predicting outcomes, and identifying biomarkers. This work sought to develop an ML model for helping APE diagnosis and compare it against current clinical probability assessment models.Methods:This is a single-center retrospective study. Patients with suspected APE were continuously enrolled and randomly divided into two groups including training and testing sets. A total of 8 ML models, including random forest (RF), Naive Bayes, decision tree, K-nearest neighbors, logistic regression, multi-layer perceptron, support vector machine, and gradient boosting decision tree were developed based on the training set to diagnose APE. Thereafter, the model with the best diagnostic performance was selected and evaluated against the current clinical assessment strategies, including the Wells score, revised Geneva score, and Years algorithm. Eventually, the ML model was internally validated to assess the diagnostic performance using receiver operating characteristic (ROC) analysis.Results:The ML models were constructed using eight clinical features, including D-dimer, cardiac troponin T (cTNT), arterial oxygen saturation, heart rate, chest pain, lower limb pain, hemoptysis, and chronic heart failure. Among eight ML models, the RF model achieved the best performance with the highest area under the curve (AUC) (AUC = 0.774). Compared to the current clinical assessment strategies, the RF model outperformed the Wells score (P = 0.030) and was not inferior to any other clinical probability assessment strategy. The AUC of the RF model for diagnosing APE onset in internal validation set was 0.726.Conclusions:Based on RF algorithm, a novel prediction model was finally constructed for APE diagnosis. When compared to the current clinical assessment strategies, the RF model achieved better diagnostic efficacy and accuracy. Therefore, the ML algorithm can be a useful tool in assisting with the diagnosis of APE.
更多查看译文
关键词
Acute pulmonary embolism,Machine learning,Wells score,Revised Geneva score,Years algorithm
AI 理解论文
溯源树
样例
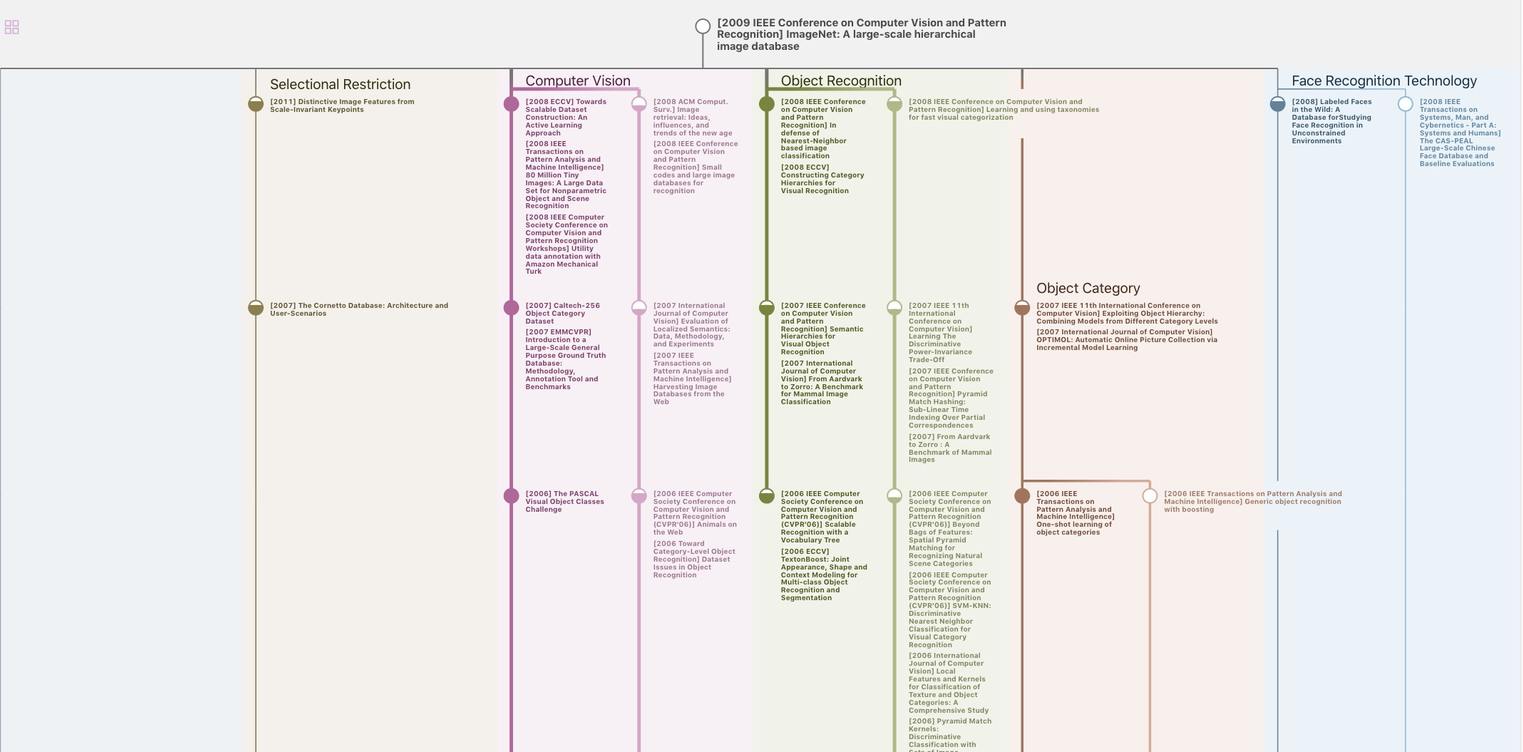
生成溯源树,研究论文发展脉络
Chat Paper
正在生成论文摘要