Land Cover and Crop Types Mapping Using Different Spatial Resolution Imagery in a Mediterranean Irrigated Area
Environmental monitoring and assessment(2023)
摘要
Crop type identification is critical for agricultural sustainability policy development and environmental assessments. Therefore, it is important to obtain their spatial distribution via different approaches. Medium-, high- and very high-resolution optical satellite sensors are efficient tools for acquiring this information, particularly for challenging studies such as those conducted in heterogeneous agricultural fields. This research examined the ability of four multitemporal datasets (Sentinel-1-SAR (S1), Sentinel-2-MSI (S2), RapidEye (RE), and PlanetScope (PS)) to identify land cover and crop types (LCCT) in a Mediterranean irrigated area. To map LCCT distribution, a supervised pixel-based classification is adopted using Support Vector Machine with a radial basis function kernel (SVMRB) and Random Forest (RF). Thus, LCCT maps were generated into three levels, including six (Level I), ten (Level II), and fourteen (Level III) classes. Overall, the findings revealed high overall accuracies of >92%, >83%, and > 81% for Level I, Level II, and Level III, respectively, except for Sentinel-1. It was found that accuracy improves considerably when the number of classes decreases, especially when cropland or non-cropland classes are grouped into one. Furthermore, there was a similarity in performance between S2 alone and S1S2. PlanetScope LCCT classifications outperform other sensors. In addition, the present study demonstrated that SVM achieved better performances against RF and can thereby effectively extract LCCT information from high-resolution imagery as PlanetScope.
更多查看译文
关键词
Crop type identification,Optical remote sensing,Sentinel-1,Machine learning
AI 理解论文
溯源树
样例
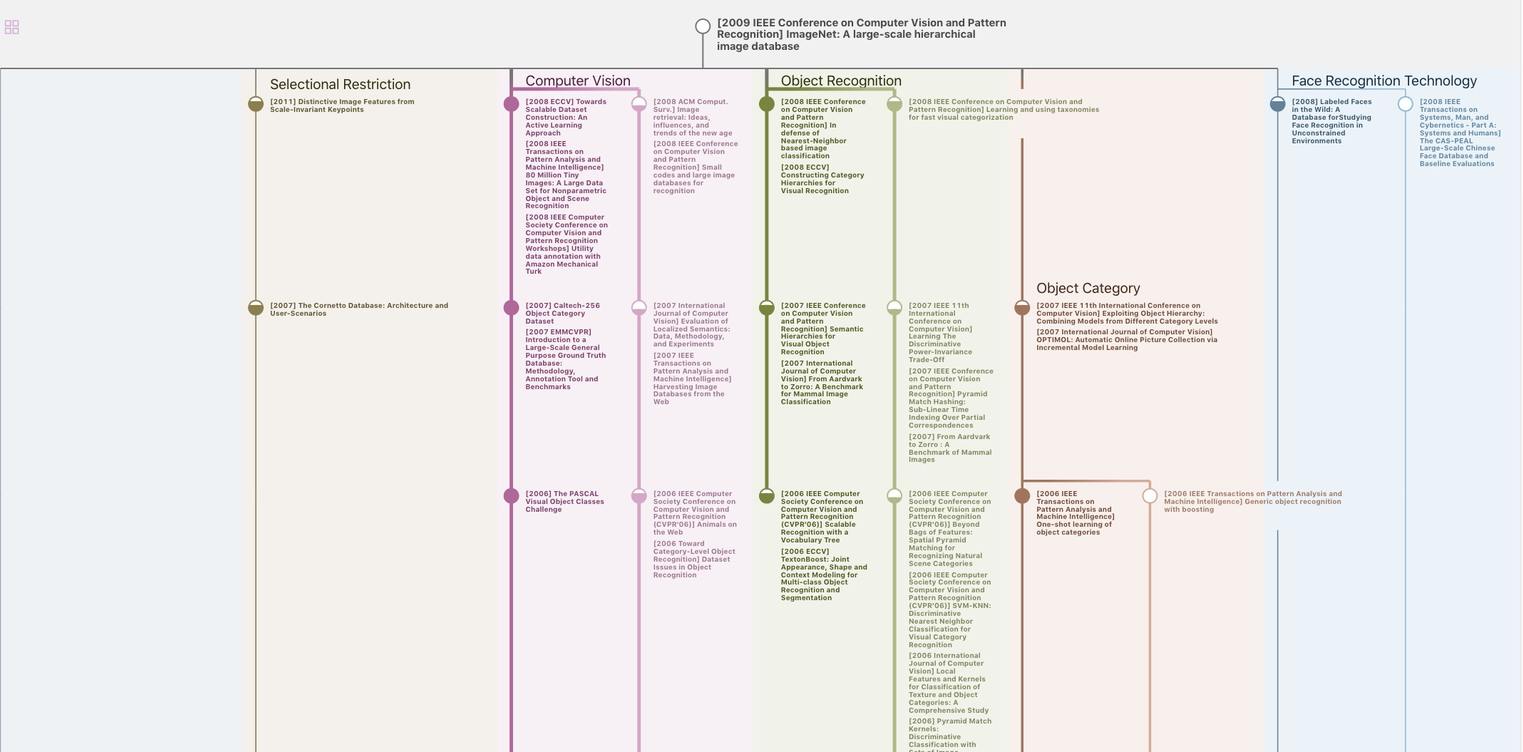
生成溯源树,研究论文发展脉络
Chat Paper
正在生成论文摘要