PARSE: A personalized clinical time-series representation learning framework via abnormal offsets analysis.
Computer methods and programs in biomedicine(2023)
摘要
BACKGROUND AND OBJECTIVE:Clinical risk prediction of patients is an important research issue in the field of healthcare, which is of great significance for the diagnosis, treatment and prevention of diseases. In recent years, a large number of deep learning-based methods have been proposed for clinical prediction by mining relevant features of patients' health condition from historical Electronic Health Records (EHRs) data. However, most of these existing methods only focus on discovering the time series characteristics of physiological indexes such as laboratory tests and physical examinations, and fail to comprehensively consider the deviation degree of these physiological indexes from the normal range and their stability, thus greatly limiting the prediction performance.
METHODS:We propose a personalized clinical time-series representation learning framework via abnormal offsets analysis named PARSE for clinical risk prediction. In PARSE, while extracting relevant temporal features from the original EHR data, we further capture relevant features of abnormal condition as complementary information from the absolute offset of each physiological index's observed values from its normal value and the relative offset between each physiological index's observed values in two adjacent time steps. Finally, an adaptive fusion module is introduced to effectively integrate the above features to obtain the personalized patient's representations for clinical risk prediction.
RESULTS:We conduct an in-hospital mortality prediction task on two public real-world datasets. PARSE achieves the highest F1 scores of 48.1% and 40.3%, outperforming the state-of-the-art methods with a boost of 2.4% and 6.2% on two datasets respectively. Furthermore, the results of ablation experiments demonstrate that the two abnormal offsets and the proposed adaptive fusion method are contributing.
CONCLUSIONS:PARSE can better extract the risk-related information from the EHRs data and improve the personalization of the patients' representations. Each part of PARSE improves the final prediction performance independently.
更多查看译文
AI 理解论文
溯源树
样例
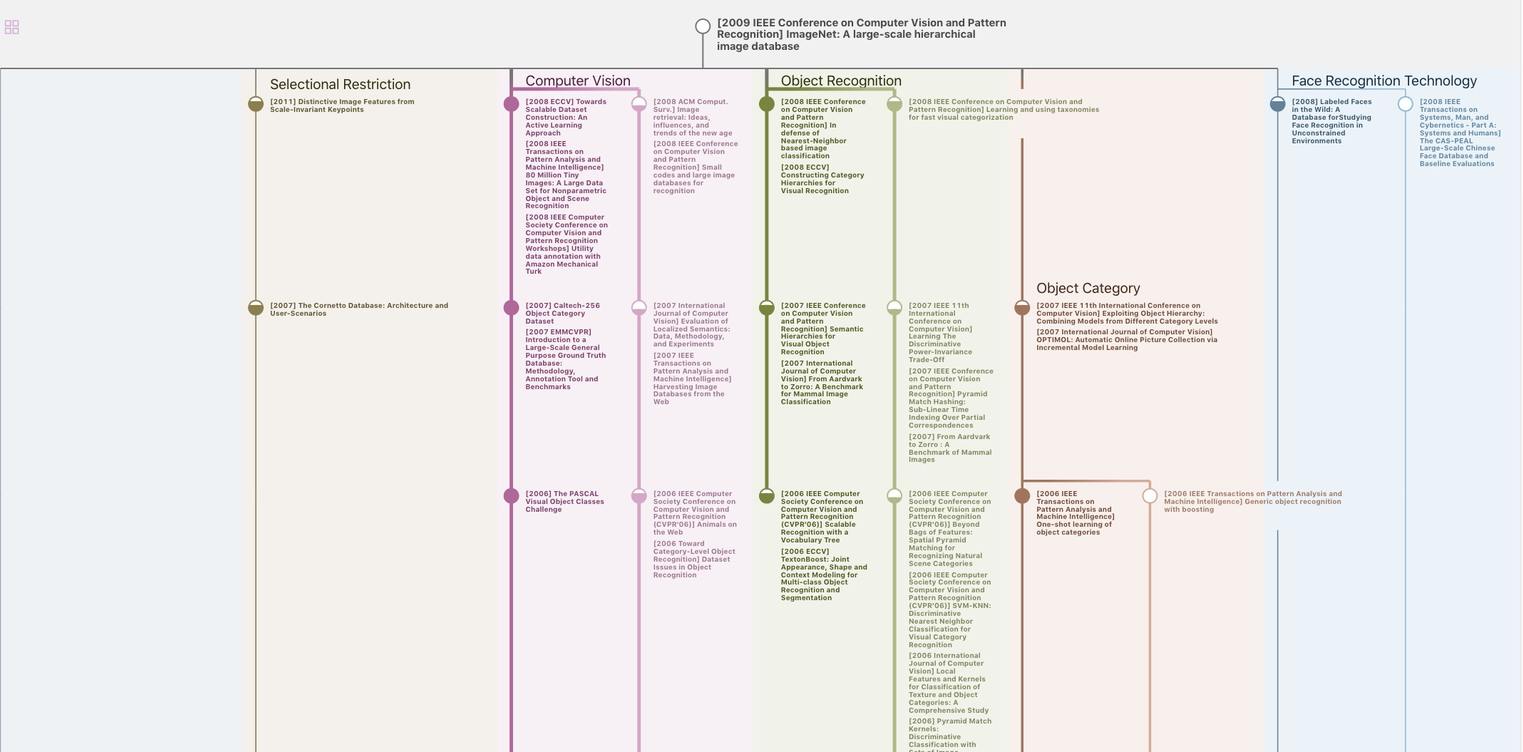
生成溯源树,研究论文发展脉络
Chat Paper
正在生成论文摘要