Two-Stage Deep Learning Framework for Quality Assessment of Left Atrial\n Late Gadolinium Enhanced MRI Images
International Workshop on Statistical Atlases and Computational Models of the Heart(2023)
摘要
Accurate assessment of left atrial fibrosis in patients with atrial fibrillation relies on high-quality 3D late gadolinium enhancement (LGE) MRI images. However, obtaining such images is challenging due to patient motion, changing breathing patterns, or sub-optimal choice of pulse sequence parameters. Automated assessment of LGE-MRI image diagnostic quality is clinically significant as it would enhance diagnostic accuracy, improve efficiency, ensure standardization, and contributes to better patient outcomes by providing reliable and high-quality LGE-MRI scans for fibrosis quantification and treatment planning. To address this, we propose a two-stage deep-learning approach for automated LGE-MRI image diagnostic quality assessment. The method includes a left atrium detector to focus on relevant regions and a deep network to evaluate diagnostic quality. We explore two training strategies, multi-task learning, and pretraining using contrastive learning, to overcome limited annotated data in medical imaging. Contrastive Learning result shows about $4\\%$, and $9\\%$ improvement in F1-Score and Specificity compared to Multi-Task learning when there's limited data.
更多查看译文
关键词
left atrial
AI 理解论文
溯源树
样例
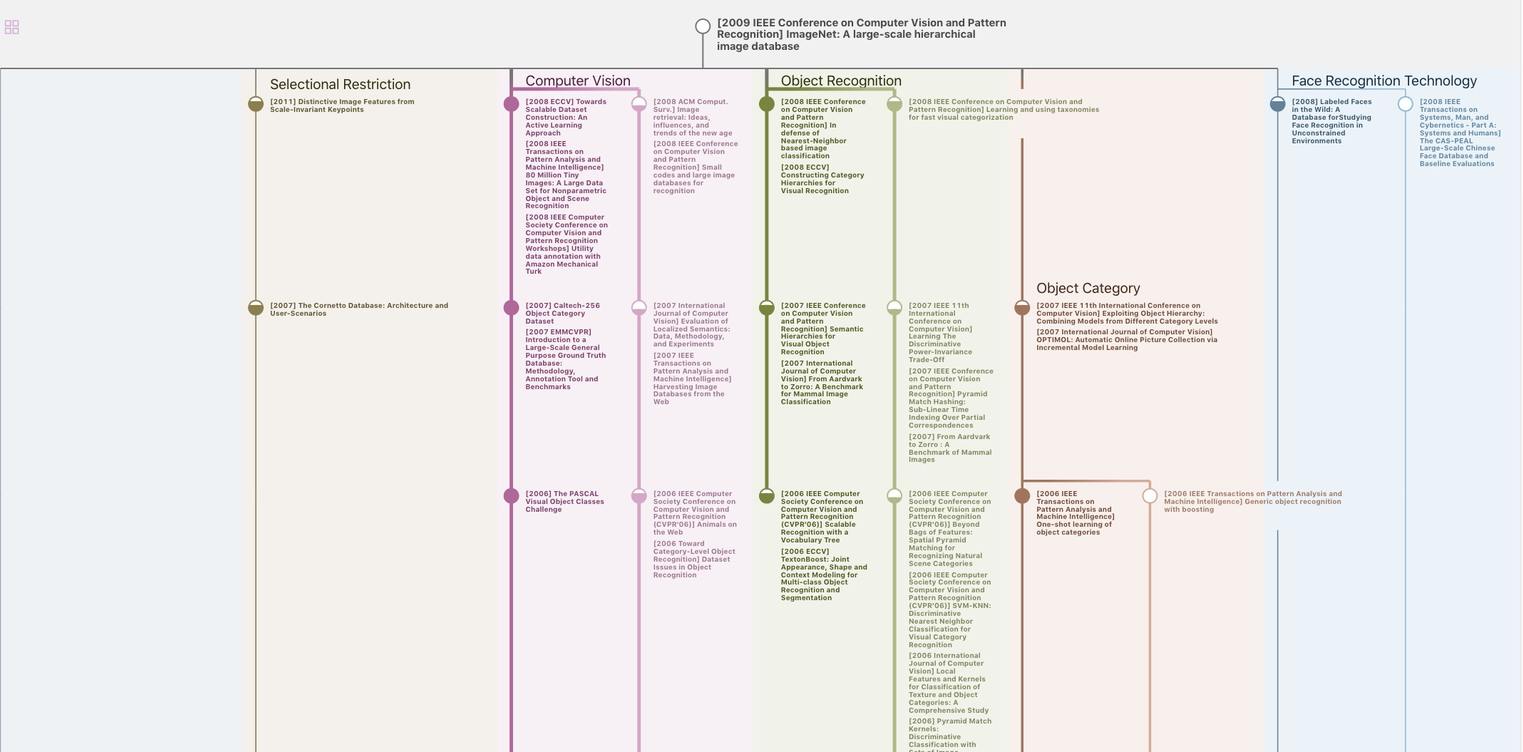
生成溯源树,研究论文发展脉络
Chat Paper
正在生成论文摘要