Selective Learning for Sample-Efficient Training in Multi-Agent Sparse Reward Tasks (extended Abstract)
IJCAI 2024(2024)
摘要
Learning effective strategies in sparse reward tasks is one of the fundamental challenges in reinforcement learning. This becomes extremely difficult in multi-agent environments, as the concurrent learning of multiple agents induces the non-stationarity problem and a sharply increased joint state space. Existing works have attempted to promote multi-agent cooperation through experience sharing. However, learning from a large collection of shared experiences is inefficient as there are only a few high-value states in sparse reward tasks, which may instead lead to the curse of dimensionality in large-scale multi-agent systems. This paper focuses on sparse-reward multi-agent cooperative tasks and proposes an effective experience-sharing method, Multi-Agent Selective Learning (MASL), to boost sample-efficient training by reusing valuable experiences from other agents. MASL adopts a retrogression-based selection method to identify high-value traces of agents from the team rewards, based on which some recall traces are generated and shared among agents to motivate effective exploration. Moreover, MASL selectively considers information from other agents to cope with the non-stationarity issue while enabling efficient training for large-scale agents. Experimental results show that MASL significantly improves sample efficiency compared with state-of-the-art MARL algorithms in cooperative tasks with sparse rewards.
更多查看译文
关键词
Machine Learning: ML: Multiagent Reinforcement Learning,Agent-based and Multi-agent Systems: MAS: Coordination and cooperation
AI 理解论文
溯源树
样例
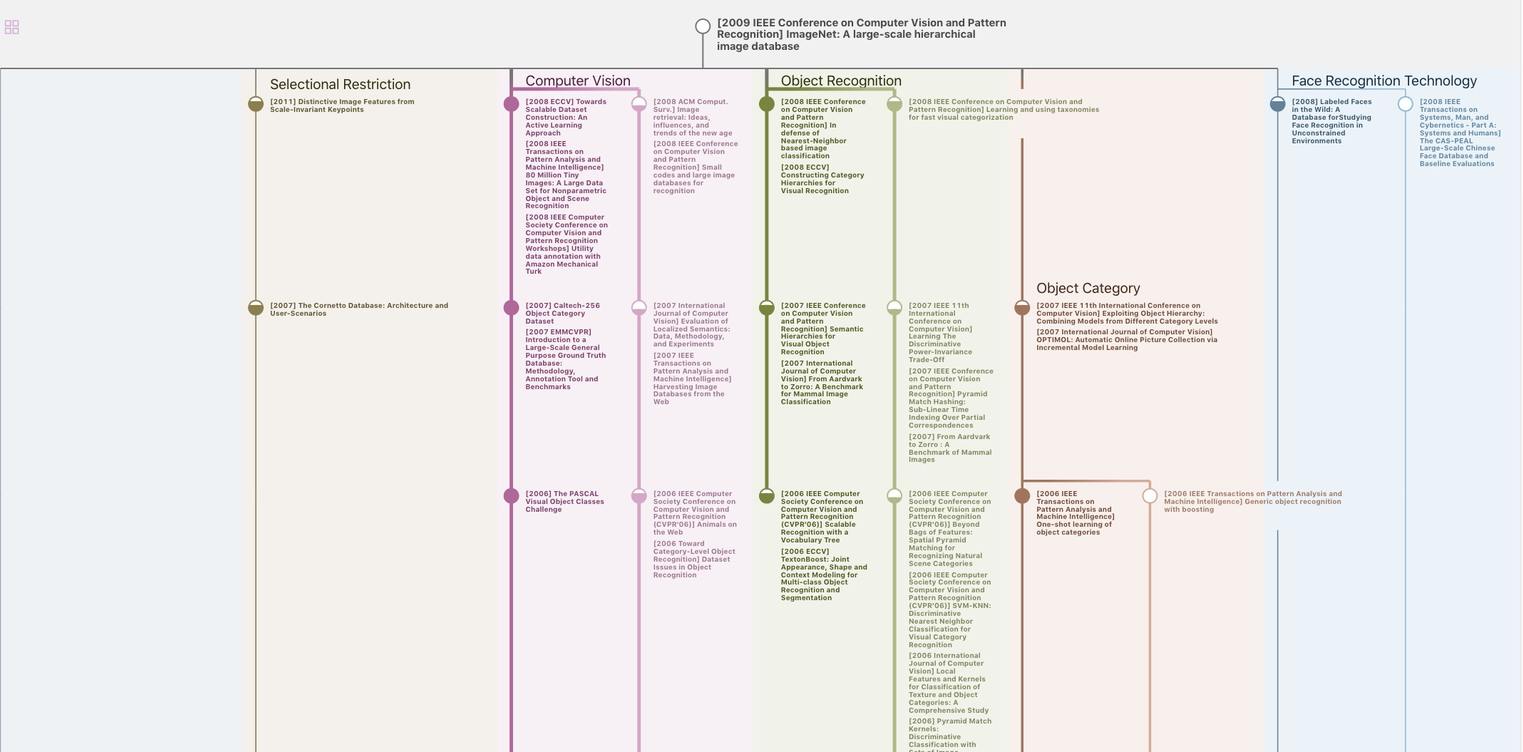
生成溯源树,研究论文发展脉络
Chat Paper
正在生成论文摘要