PiercingEye: Identifying Both Faint and Distinct Clues for Explainable Fake News Detection with Progressive Dynamic Graph Mining
ECAI 2023(2023)
摘要
Explainability is crucial for the successful use of AI for fake news detection (FND). Researchers aim to improve the explainability of FND by highlighting important descriptions in crowd-contributed comments as clues. From the perspective of law and sociology, there are distinct clues that are easy to discover and understand, and faint clues that require careful observation and analysis. For example, in fake new related to COVID-Omicron showing increased pathogenicity and transmissibility, distinct clues might involve virologists’ opinions regarding the inverse correlation between pathogenicity and transmissibility. Meanwhile, faint clues might be reflected in an infected person’s claim that the symptoms are milder than a cold (indirectly indicating reduced pathogenicity). Occasionally, the statements of some ordinary eyewitness can decisively reveal the truth of news, leading to the judgment of fake news. Existing methods generally use static networks to model the entire news life-cycle, which makes it fail to capture the subtle dynamic interactions between individual clues and news. Thereby faint clues, whose relations to the truth of news are challenging to be characterized and extracted directly, are more likely to be overshadowed by distinct clues. To address this issue, we propose an explainable FND method, dubbed as PiercingEye, which leverages dynamic interaction information to progressively mine valuable clues. PiercingEye models the news propagation topology as a dynamic graph, with interactive comments serving as nodes, and employs the time-semantic encoding mechanism to refine the modeling of temporal interaction information between comments and news to preserve faint clues. Subsequently, it utilizes the self-attention mechanism to aggregate distinct and faint clues for FND. Experimental results demonstrate that PiercingEye outperforms state-of-the-art methods and is capable of identifying both faint and distinct clues for humans to debunk fake news.
更多查看译文
关键词
explainable fake news detection,progressive dynamic graph mining
AI 理解论文
溯源树
样例
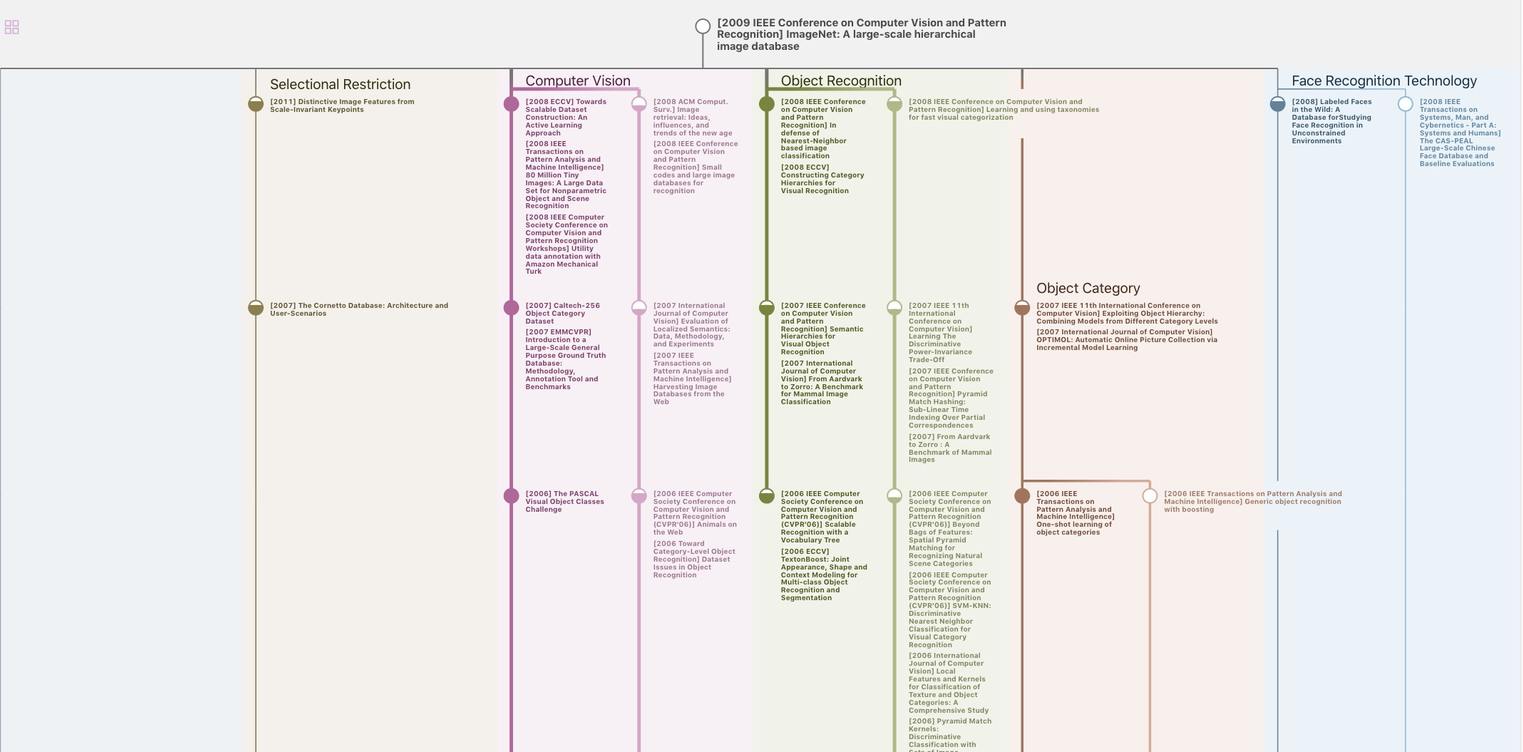
生成溯源树,研究论文发展脉络
Chat Paper
正在生成论文摘要