S2M: Converting Single-Turn to Multi-Turn Datasets for Conversational Question Answering
ECAI 2023(2023)
摘要
Supplying data augmentation to conversational question answering (CQA) can
effectively improve model performance. However, there is less improvement from
single-turn datasets in CQA due to the distribution gap between single-turn and
multi-turn datasets. On the other hand, while numerous single-turn datasets are
available, we have not utilized them effectively. To solve this problem, we
propose a novel method to convert single-turn datasets to multi-turn datasets.
The proposed method consists of three parts, namely, a QA pair Generator, a QA
pair Reassembler, and a question Rewriter. Given a sample consisting of context
and single-turn QA pairs, the Generator obtains candidate QA pairs and a
knowledge graph based on the context. The Reassembler utilizes the knowledge
graph to get sequential QA pairs, and the Rewriter rewrites questions from a
conversational perspective to obtain a multi-turn dataset S2M. Our experiments
show that our method can synthesize effective training resources for CQA.
Notably, S2M ranks 1st place on the QuAC leaderboard at the time of submission
(Aug 24th, 2022).
更多查看译文
关键词
conversational question answering,single-turn,multi-turn
AI 理解论文
溯源树
样例
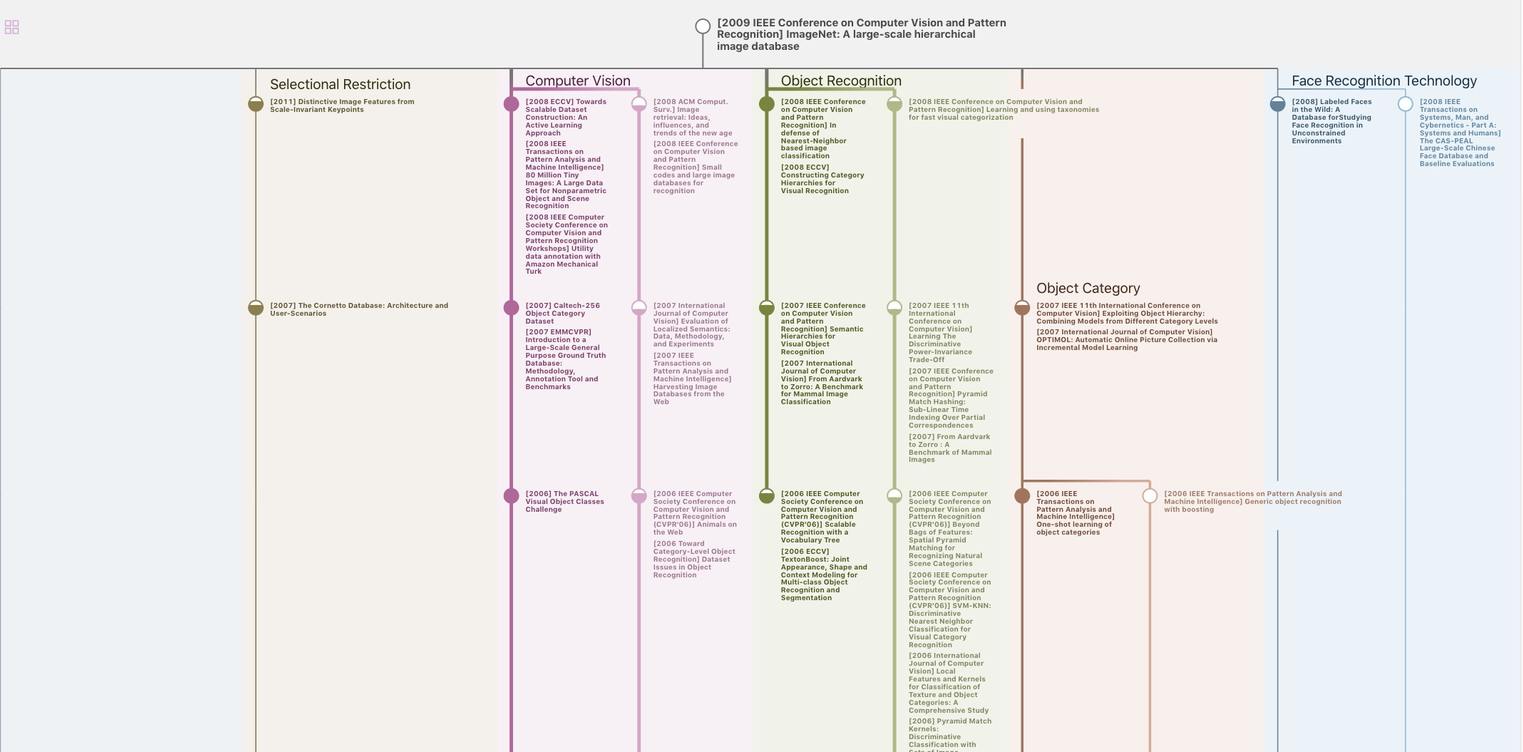
生成溯源树,研究论文发展脉络
Chat Paper
正在生成论文摘要