Disentangling Interaction Using Maximum Entropy Reinforcement Learning in Multi-Agent Systems
ECAI 2023(2023)
摘要
Research on multi-agent interaction involving both multiple artificial agents and humans is still in its infancy. Most recent approaches have focused on environments with collaboration-focused human behavior, or providing only a small, defined set of situations. When deploying robots in human-inhabited environments in the future, it will be unlikely that all interactions fit a predefined model of collaboration, where collaborative behavior is still expected from the robot. Existing approaches are unlikely to effectively create such behaviors in such “coexistence” environments. To tackle this issue, we introduce a novel framework that decomposes interaction and task-solving into separate learning problems and blends the resulting policies at inference time. Policies are learned with maximum entropy reinforcement learning, allowing us to create interaction-impact-aware agents and scale the cost of training agents linearly with the number of agents and available tasks. We propose a weighting function covering the alignment of interaction distributions with the original task. We demonstrate that our framework addresses the scaling problem while solving a given task and considering collaboration opportunities in a co-existence particle environment and a new cooking environment. Our work introduces a new learning paradigm that opens the path to more complex multi-robot, multi-human interactions.
更多查看译文
关键词
maximum entropy reinforcement learning,reinforcement learning,interaction,multi-agent
AI 理解论文
溯源树
样例
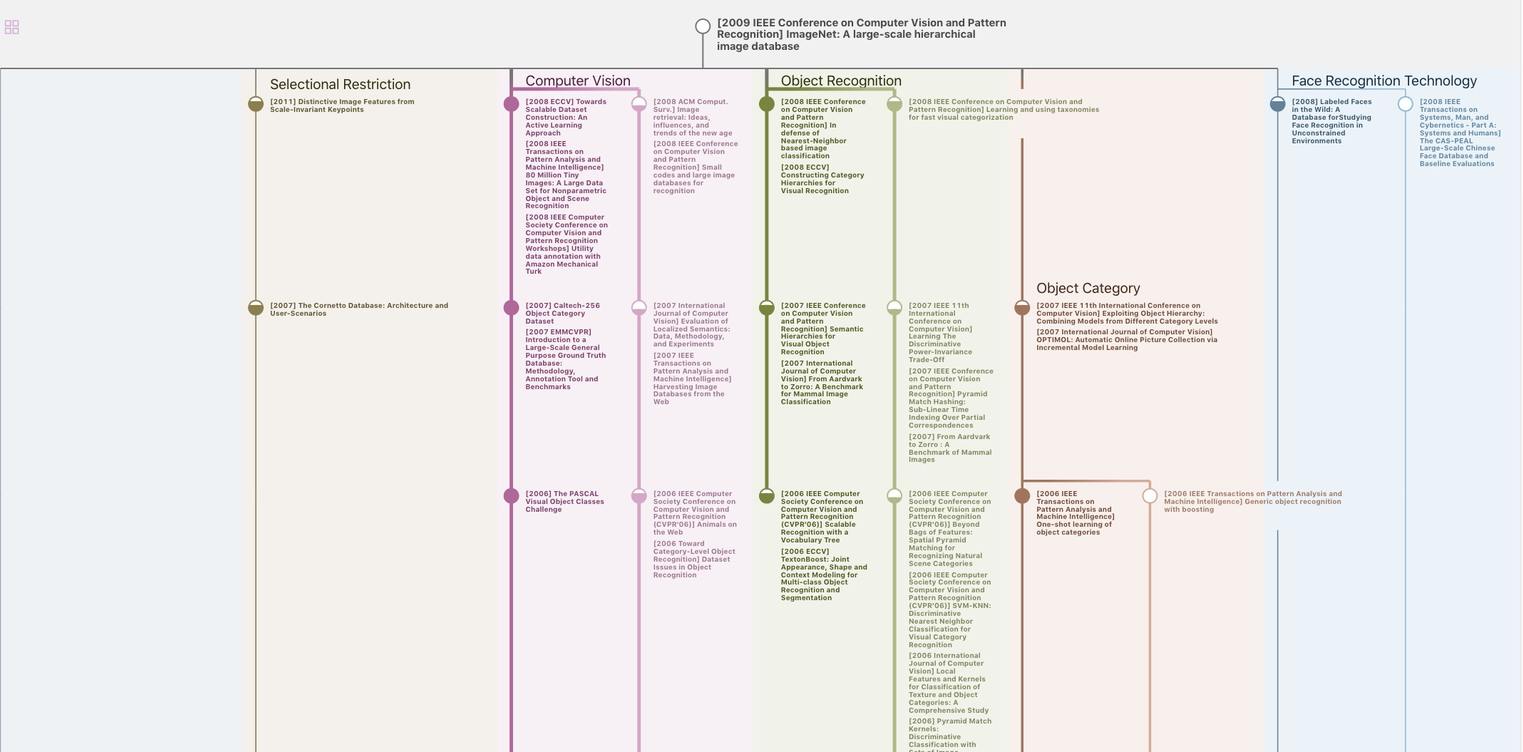
生成溯源树,研究论文发展脉络
Chat Paper
正在生成论文摘要