Efficient Information Modulation Network for Image Super-Resolution
ECAI 2023(2023)
摘要
Recent researches have shown that the success of Transformers comes from their macro-level framework and advanced components, not just their self-attention (SA) mechanism. Comparable results can be obtained by replacing SA with spatial pooling, shifting, MLP, fourier transform and constant matrix, all of which have spatial information encoding capability like SA. In light of these findings, this work focuses on combining efficient spatial information encoding technology with superior macro architectures in Transformers. We rethink spatial convolution to achieve more efficient encoding of spatial features and dynamic modulation value representations by convolutional modulation techniques. The large-kernel convolution and Hadamard product are utilizated in the proposed Multi-orders Long-range convolutional modulation (MOLRCM) layer to imitate the implementation of SA. Moreover, MOLRCM layer also achieve long-range correlations and self-adaptation behavior, similar to SA, with linear complexity. On the other hand, we also address the sub-optimality of vanilla feed-forward networks (FFN) by introducing spatial awareness and locality, improving feature diversity, and regulating information flow between layers in the proposed Spatial Awareness Dynamic Feature Flow Modulation (SADFFM) layer. Experiment results show that our proposed efficient information modulation network (EIMN) performs better both quantitatively and qualitatively. Codes and supplementary materials link: https://github.com/liux520/EIMN.
更多查看译文
关键词
network,super-resolution
AI 理解论文
溯源树
样例
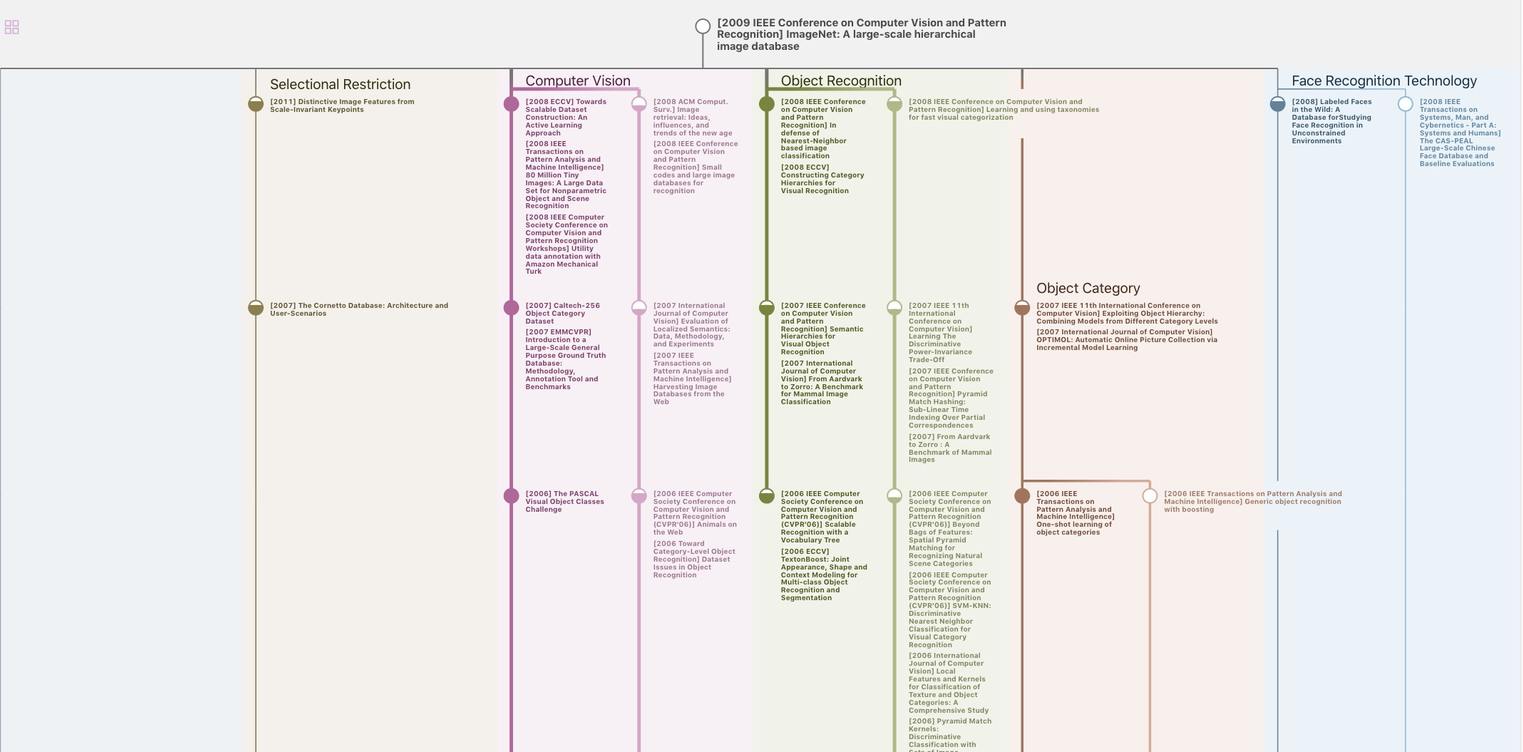
生成溯源树,研究论文发展脉络
Chat Paper
正在生成论文摘要