Adaptive Self-Supervised Continual Learning
ECAI 2023(2023)
摘要
Continual Learning (CL) studies the problem of developing a robust model that can learn new tasks while retaining previously learned knowledge. However, the current CL methods exclusively focus on data with annotations, disregarding that unlabelled data is the mainstream in real-world applications. To close this research gap, this study concentrates on continual self-supervised learning, which is plagued by challenges of memory over-fitting and class imbalance. Besides, these challenges are exacerbated throughout incremental training. Aimed at addressing these challenges from both loss and data perspectives, we introduce a framework, Adaptive Self-supervised Continual Learning (ASCL). Specifically, we devise an Adaptive Sharpness-Aware Minimization (ASAM) module responsible for identifying flatter local minima in the loss landscape with a smaller memory over-fitting risk. Additionally, we design an Adaptive Memory Enhancement (AME) module responsible for rebalancing self-supervised loss with new and old tasks from a data perspective. Finally, the adaptive mechanisms in AME and ASAM modules dynamically adjust the loss landscape sharpness and memory enhancement strength with the feedback of intermediate training results. The results of our extensive experiments demonstrate the state-of-the-art performance of our methods in continual self-supervised learning scenarios across multiple datasets.
更多查看译文
关键词
learning,self-supervised
AI 理解论文
溯源树
样例
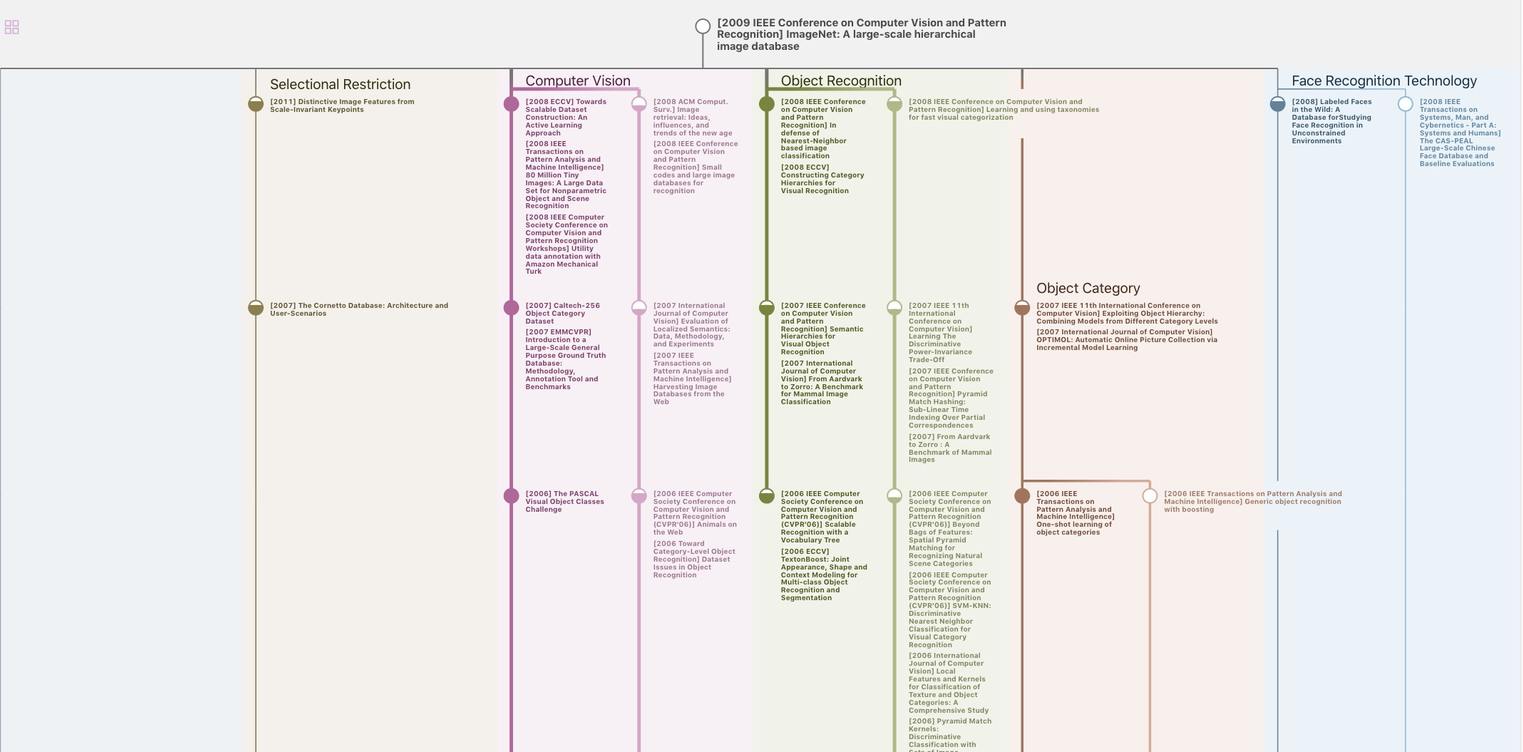
生成溯源树,研究论文发展脉络
Chat Paper
正在生成论文摘要