Enhancing Link Prediction with Self-Discriminating Augmentation for Structure-Aware Contrastive Learning
ECAI 2023(2023)
摘要
Link prediction is a crucial research area for both data mining and machine learning. Despite the success of contrastive learning in node classification tasks, applying it directly to link prediction tasks has revealed two major weaknesses, i.e., single positive sample contrasting and random augmentation, resulting in inferior performance. To overcome these issues, we propose a new contrastive learning approach for link prediction, called Structure-aware Contrastive Representation Learning with Self-discriminating Augmentation (SECRET). Our approach includes a novel data augmentation scheme based on the prediction model itself and takes into account both the contrastive objective and the reconstruction loss, which jointly improve the performance of link prediction. Our experiments on 11 benchmark datasets demonstrate that SECRET significantly outperforms the other state-of-the-art baselines.
更多查看译文
关键词
link prediction,learning,self-discriminating,structure-aware
AI 理解论文
溯源树
样例
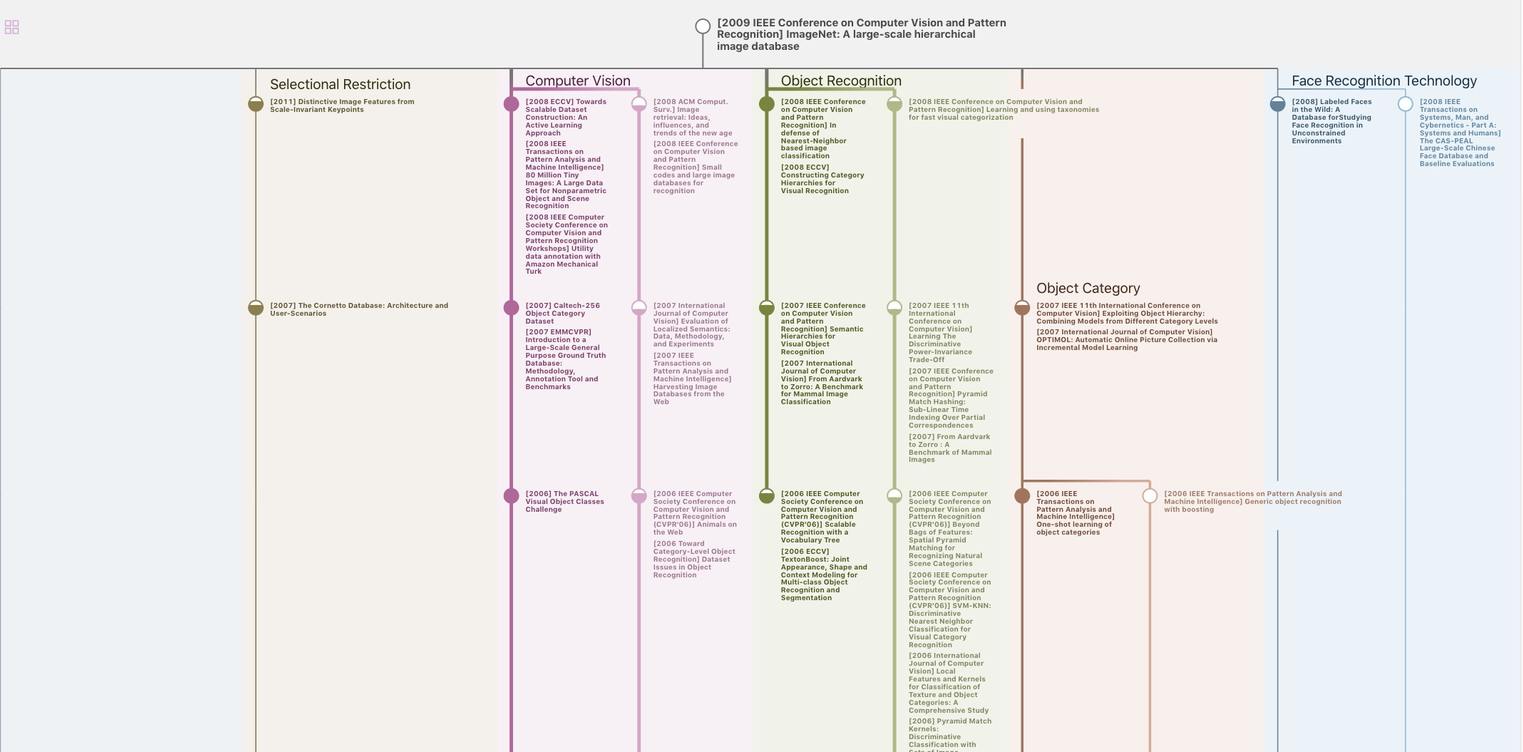
生成溯源树,研究论文发展脉络
Chat Paper
正在生成论文摘要