Adversarial Benchmark Evaluation Rectified by Controlling for Difficulty
ECAI 2023(2023)
摘要
Adversarial benchmark construction, where harder instances challenge new generations of AI systems, is becoming the norm. While this approach may lead to better machine learning models —on average and for the new benchmark—, it is unclear how these models behave on the original distribution. Two opposing effects are intertwined here. On the one hand, the adversarial benchmark has a higher proportion of difficult instances, with lower expected performance. On the other hand, models trained on the adversarial benchmark may improve on these difficult instances (but may also neglect some easy ones). To disentangle these two effects we can control for difficulty, showing that we can recover the performance on the original distribution, provided the harder instances were obtained from this distribution in the first place. We show this difficulty-aware rectification works in practice, through a series of experiments with several benchmark construction schemas and the use of a populational difficulty metric. As a take-away message, instead of distributional averages we recommend using difficulty-conditioned characteristic curves when evaluating models built with adversarial benchmarks.
更多查看译文
关键词
difficulty,evaluation
AI 理解论文
溯源树
样例
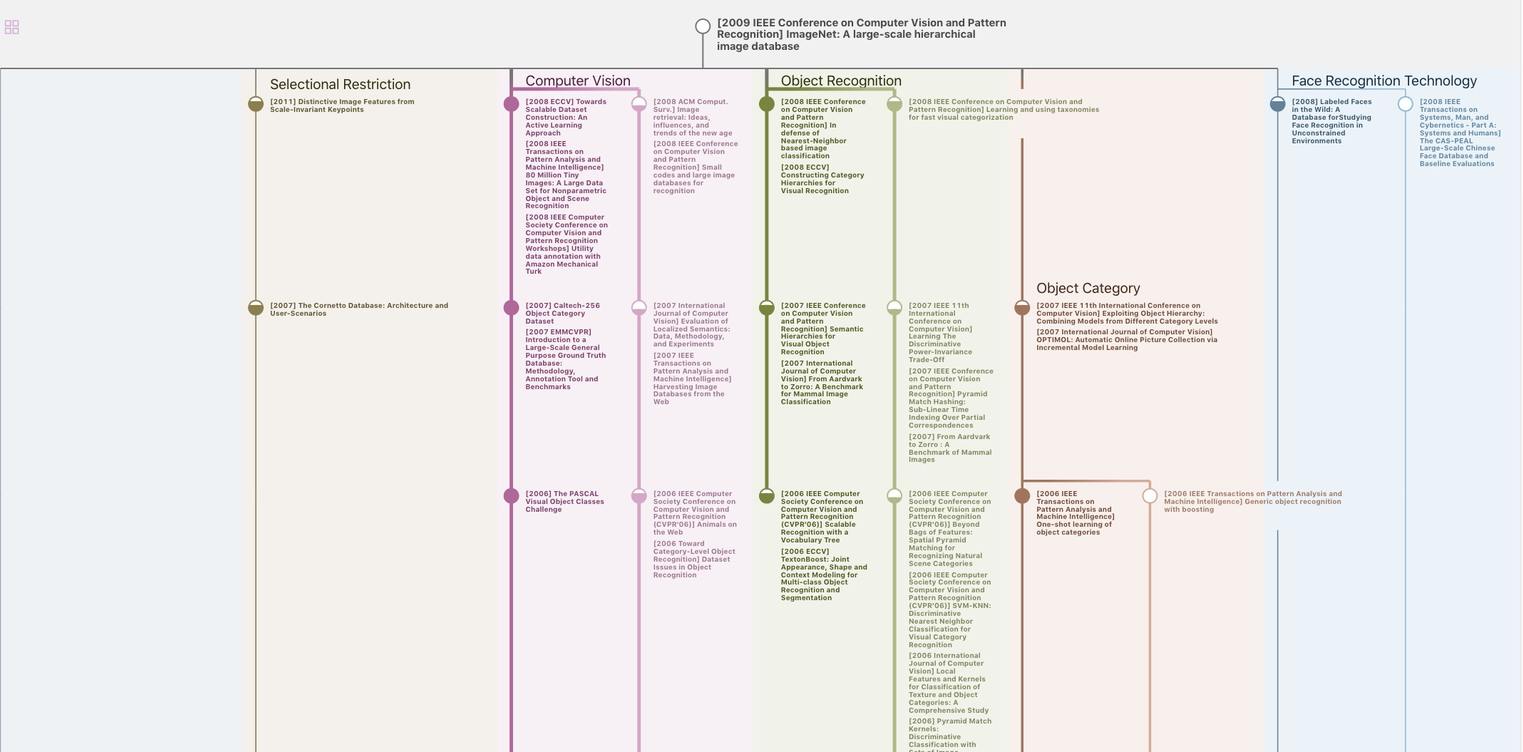
生成溯源树,研究论文发展脉络
Chat Paper
正在生成论文摘要