Constrained Recalibration of Reynolds-Averaged Navier-Stokes Models
arXiv (Cornell University)(2023)
摘要
The constants and functions in Reynolds-averaged Navier-Stokes (RANS) turbulence models are coupled. Consequently, modifications of a RANS model often negatively impact its basic calibrations, which is why machine-learned augmentations are often detrimental outside the training dataset. A solution to this is to identify the degrees of freedom that do not affect the basic calibrations and only modify these identified degrees of freedom when recalibrating the baseline model to accommodate a specific application. This approach is colloquially known as the "rubber-band" approach, which we formally call "constrained model recalibration" in this paper. To illustrate the efficacy of the approach, we identify the degrees of freedom in the Spalart-Allmaras model that do not affect the log law calibration. By subsequently interfacing data-based methods with these degrees of freedom, we train models to solve historically challenging flow scenarios, including the round-jet/plane-jet anomaly, airfoil stall, secondary flow separation, and recovery after separation. In addition to good performance inside the training dataset, the trained models yield similar performance as the baseline model outside the training dataset.
更多查看译文
关键词
Reynolds Averaged Navier Stokes,Bayesian Optimization,Turbulence Models
AI 理解论文
溯源树
样例
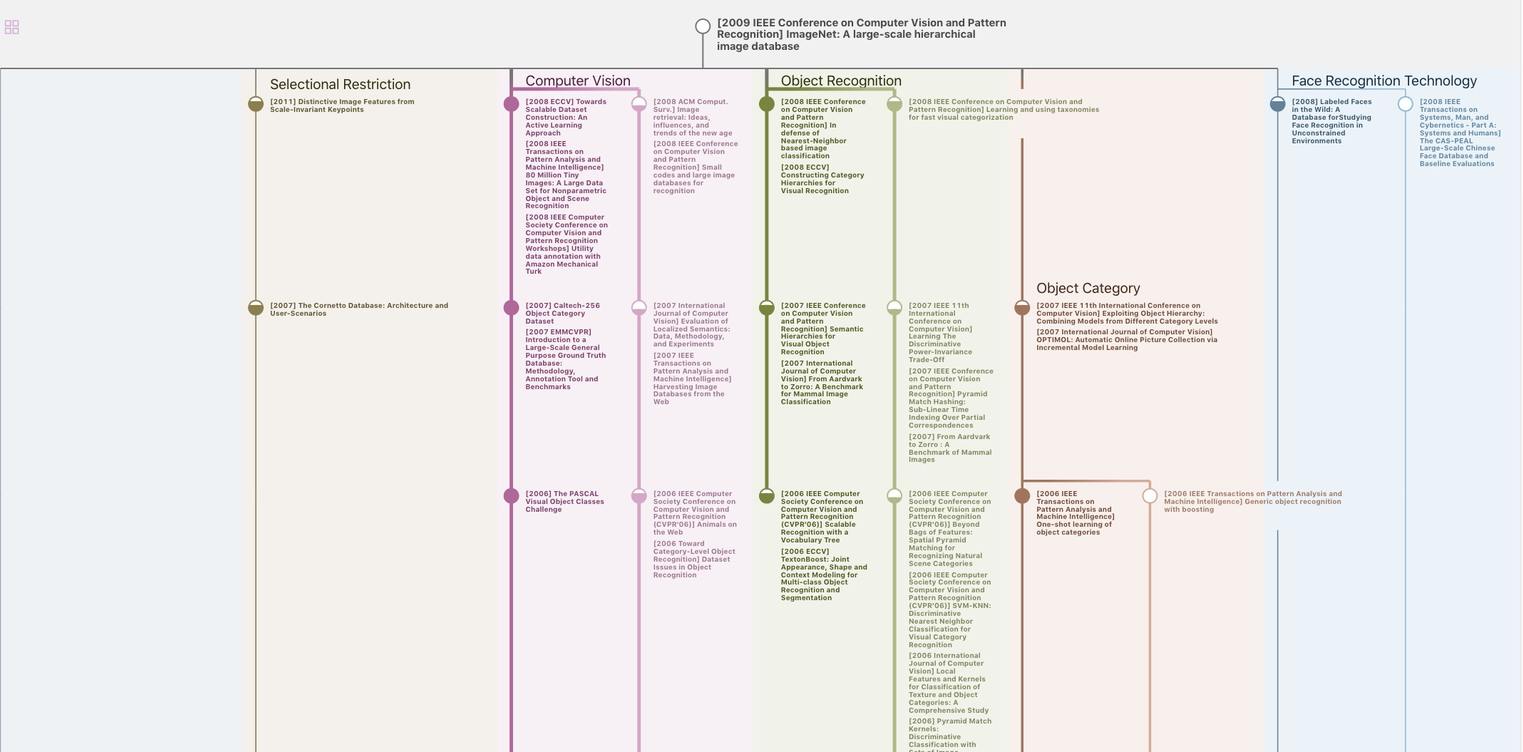
生成溯源树,研究论文发展脉络
Chat Paper
正在生成论文摘要