Auto-LfD: Towards Closing the Loop for Learning from Demonstrations
CoRR(2023)
摘要
Over the past few years, there have been numerous works towards advancing the generalization capability of robots, among which learning from demonstrations (LfD) has drawn much attention by virtue of its user-friendly and data-efficient nature. While many LfD solutions have been reported, a key question has not been properly addressed: how can we evaluate the generalization performance of LfD? For instance, when a robot draws a letter that needs to pass through new desired points, how does it ensure the new trajectory maintains a similar shape to the demonstration? This question becomes more relevant when a new task is significantly far from the demonstrated region. To tackle this issue, a user often resorts to manual tuning of the hyperparameters of an LfD approach until a satisfactory trajectory is attained. In this paper, we aim to provide closed-loop evaluative feedback for LfD and optimize LfD in an automatic fashion. Specifically, we consider dynamical movement primitives (DMP) and kernelized movement primitives (KMP) as examples and develop a generic optimization framework capable of measuring the generalization performance of DMP and KMP and auto-optimizing their hyperparameters without any human inputs. Evaluations including a peg-in-hole task and a pushing task on a real robot evidence the applicability of our framework.
更多查看译文
关键词
demonstrations,learning,loop,auto-lfd
AI 理解论文
溯源树
样例
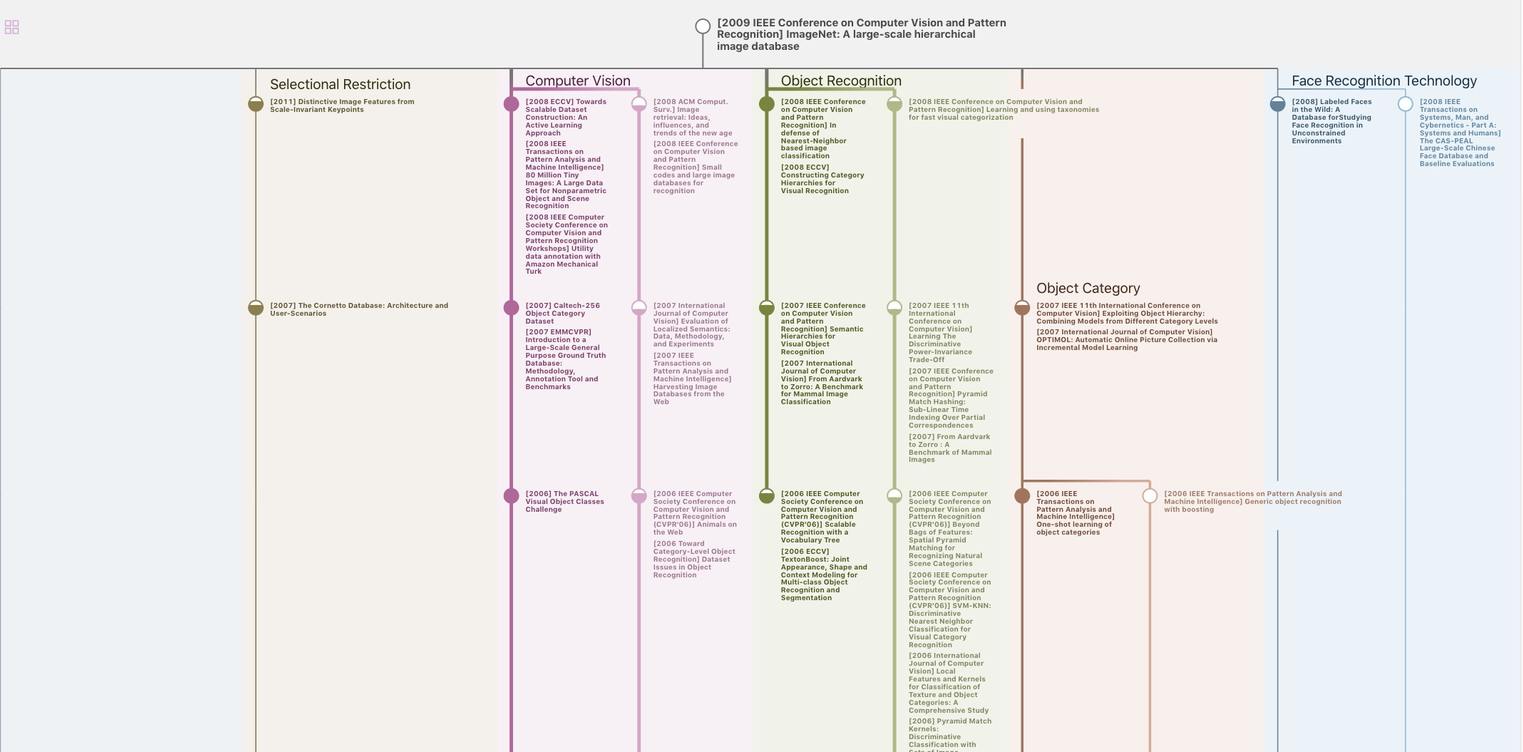
生成溯源树,研究论文发展脉络
Chat Paper
正在生成论文摘要