XRMDN: An Extended Recurrent Mixture Density Network for Short-Term Probabilistic Rider Demand Forecasting with High Volatility
arxiv(2023)
摘要
In the realm of Mobility-on-Demand (MoD) systems, the forecasting of rider
demand is a cornerstone for operational decision-making and system
optimization. Traditional forecasting methodologies primarily yield point
estimates, thereby neglecting the inherent uncertainty within demand
projections. Moreover, MoD demand levels are profoundly influenced by both
endogenous and exogenous factors, leading to high and dynamic volatility. This
volatility significantly undermines the efficacy of conventional time series
forecasting methods. In response, we propose an Extended Recurrent Mixture
Density Network (XRMDN), a novel deep learning framework engineered to address
these challenges. XRMDN leverages a sophisticated architecture to process
demand residuals and variance through correlated modules, allowing for the
flexible incorporation of endogenous and exogenous data. This architecture,
featuring recurrent connections within the weight, mean, and variance neural
networks, adeptly captures demand trends, thus significantly enhancing
forecasting precision, particularly in high-volatility scenarios. Our
comprehensive experimental analysis, utilizing real-world MoD datasets,
demonstrates that XRMDN surpasses the existing benchmark models across various
metrics, notably excelling in high-demand volatility contexts. This advancement
in probabilistic demand forecasting marks a significant contribution to the
field, offering a robust tool for enhancing operational efficiency and customer
satisfaction in MoD systems.
更多查看译文
关键词
probabilistic demand forecasting,networks-based,short-term,mobility-on-demand
AI 理解论文
溯源树
样例
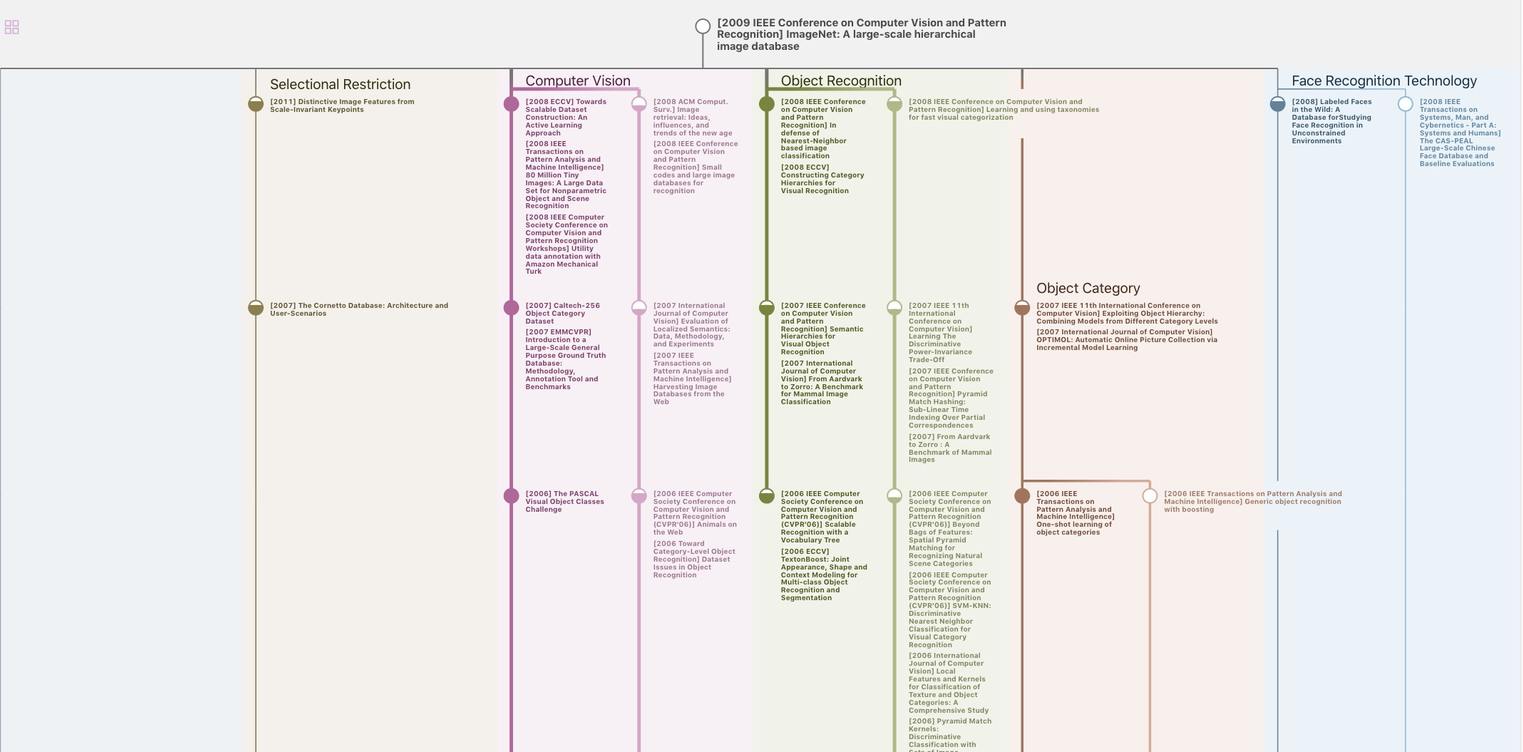
生成溯源树,研究论文发展脉络
Chat Paper
正在生成论文摘要