Towards Unified and Effective Domain Generalization
CoRR(2023)
摘要
We propose $\textbf{UniDG}$, a novel and $\textbf{Uni}$fied framework for $\textbf{D}$omain $\textbf{G}$eneralization that is capable of significantly enhancing the out-of-distribution generalization performance of foundation models regardless of their architectures. The core idea of UniDG is to finetune models during the inference stage, which saves the cost of iterative training. Specifically, we encourage models to learn the distribution of test data in an unsupervised manner and impose a penalty regarding the updating step of model parameters. The penalty term can effectively reduce the catastrophic forgetting issue as we would like to maximally preserve the valuable knowledge in the original model. Empirically, across 12 visual backbones, including CNN-, MLP-, and Transformer-based models, ranging from 1.89M to 303M parameters, UniDG shows an average accuracy improvement of +5.4% on DomainBed. These performance results demonstrate the superiority and versatility of UniDG. The code is publicly available at https://github.com/invictus717/UniDG
更多查看译文
关键词
effective domain generalization,unified
AI 理解论文
溯源树
样例
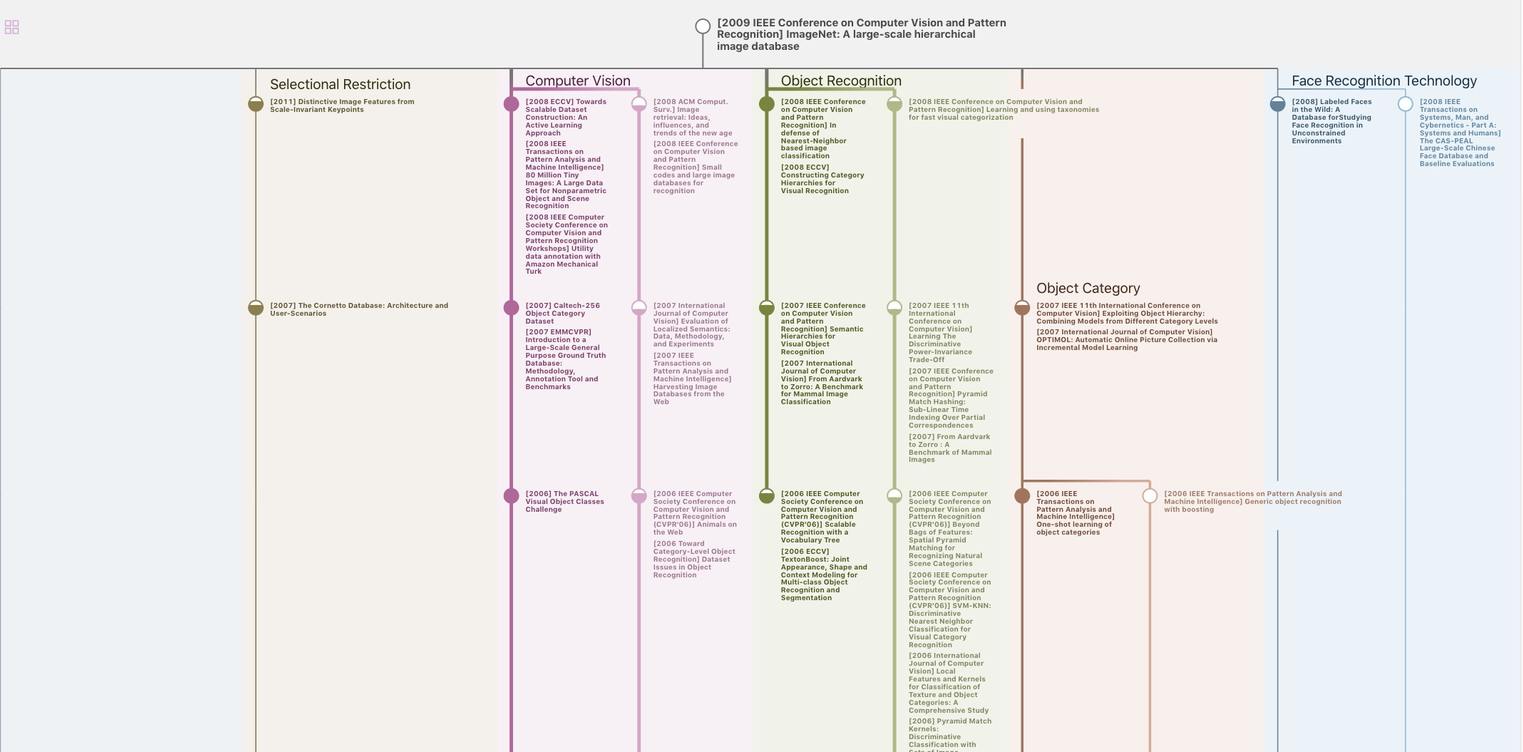
生成溯源树,研究论文发展脉络
Chat Paper
正在生成论文摘要