A Novel Benchmarking Paradigm and a Scale- and Motion-Aware Model for Egocentric Pedestrian Trajectory Prediction
CoRR(2023)
摘要
Predicting pedestrian behavior is one of the main challenges for intelligent driving systems. In this paper, we present a new paradigm for evaluating egocentric pedestrian trajectory prediction algorithms. Based on various contextual information, we extract driving scenarios for a meaningful and systematic approach to identifying challenges for prediction models. In this regard, we also propose a new metric for more effective ranking within the scenario-based evaluation. We conduct extensive empirical studies of existing models on these scenarios to expose shortcomings and strengths of different approaches. The scenario-based analysis highlights the importance of using multimodal sources of information and challenges caused by inadequate modeling of ego-motion and scale of pedestrians. To this end, we propose a novel egocentric trajectory prediction model that benefits from multimodal sources of data fused in an effective and efficient step-wise hierarchical fashion and two auxiliary tasks designed to learn more robust representation of scene dynamics. We show that our approach achieves significant improvement by up to 40% in challenging scenarios compared to the past arts via empirical evaluation on common benchmark datasets.
更多查看译文
关键词
novel benchmarking paradigm,prediction,motion-aware
AI 理解论文
溯源树
样例
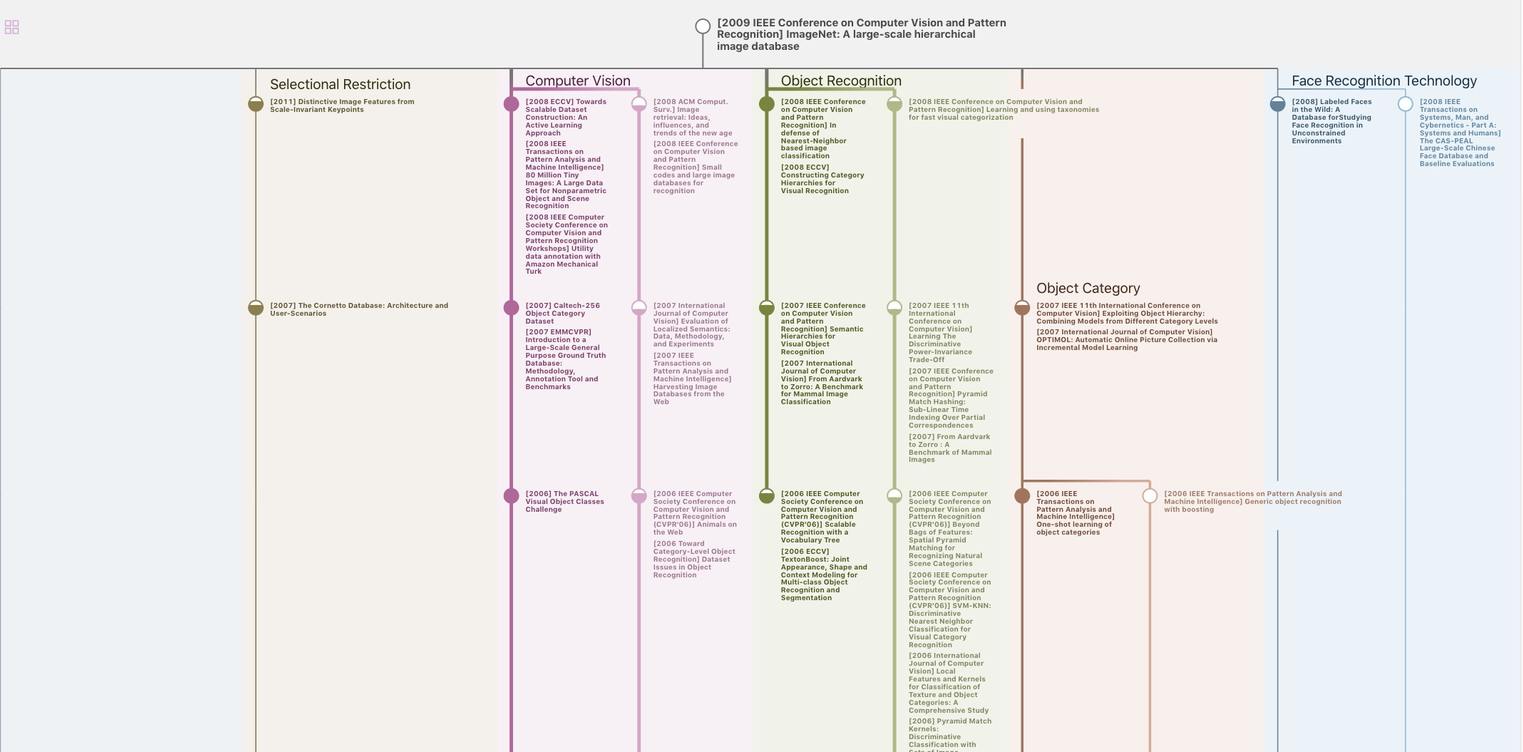
生成溯源树,研究论文发展脉络
Chat Paper
正在生成论文摘要