Metric Ensembles For Hallucination Detection
CoRR(2023)
摘要
Abstractive text summarization has garnered increased interest as of late, in part due to the proliferation of large language models (LLMs). One of the most pressing problems related to generation of abstractive summaries is the need to reduce "hallucinations," information that was not included in the document being summarized, and which may be wholly incorrect. Due to this need, a wide array of metrics estimating consistency with the text being summarized have been proposed. We examine in particular a suite of unsupervised metrics for summary consistency, and measure their correlations with each other and with human evaluation scores in the wiki_bio_gpt3_hallucination dataset. We then compare these evaluations to models made from a simple linear ensemble of these metrics. We find that LLM-based methods outperform other unsupervised metrics for hallucination detection. We also find that ensemble methods can improve these scores even further, provided that the metrics in the ensemble have sufficiently similar and uncorrelated error rates. Finally, we present an ensemble method for LLM-based evaluations that we show improves over this previous SOTA.
更多查看译文
关键词
metric ensembles,detection
AI 理解论文
溯源树
样例
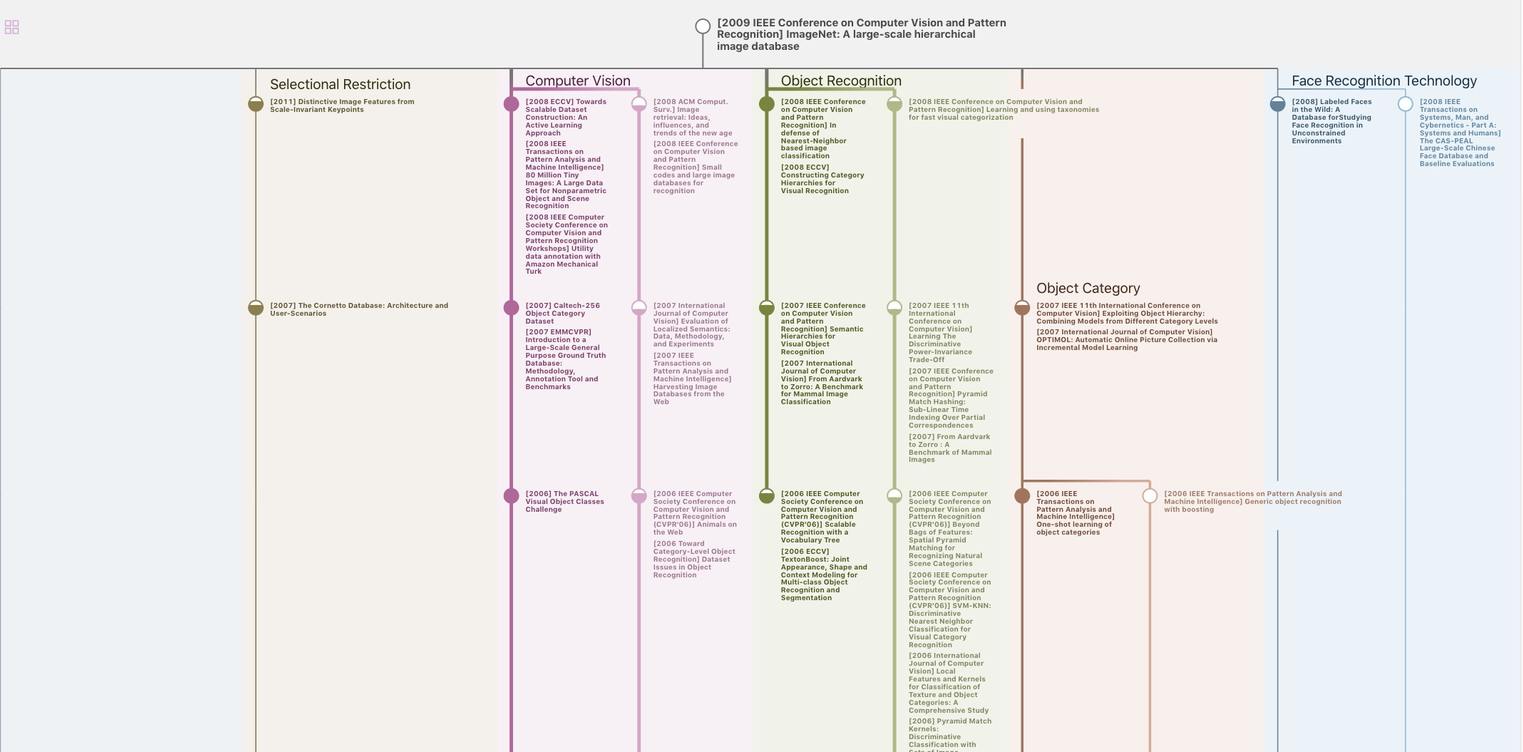
生成溯源树,研究论文发展脉络
Chat Paper
正在生成论文摘要