Beyond Motor Symptoms: Toward a Comprehensive Grading of Parkinson's Disease Severity
14TH ACM CONFERENCE ON BIOINFORMATICS, COMPUTATIONAL BIOLOGY, AND HEALTH INFORMATICS, BCB 2023(2023)
摘要
This study applies machine learning (ML) feature analysis to an array of multi-functional neurocognitive symptoms specific to individuals with Parkinson's Disease (PD). We provide a framework that can assist with modernizing and objectively individualizing the staging of PD. For that purpose, a hybrid feature score technique is proposed to compute a weighted vector for neurocognitive functions. The methodology is based on Principal Component Analysis and Random Forest for feature selection and extraction purposes. The study enrolled 37 participants who completed various tablet-based functional neurocognitive assessments for motor, memory, speech, executive function, and single versus multi-functional tasks. The study concludes that current assessment and staging schemes exhibit a significant bias toward fine-motor functionalities. Thus, the inclusion of other neurocognitive functions is essential for accurately identifying disease stages. This could be achieved through the integration of multiple functions into a unified score or by adopting function-specific staging. By incorporating ML into disease staging, a more comprehensive understanding of neurocognitive disorders can be obtained, revealing novel insights that affect the design and implementation of staging schemes.
更多查看译文
关键词
Machine Learning,Parkinson's Disease,Disease Staging,Digital Health,Neurocognitive Disorder,Mobile Device
AI 理解论文
溯源树
样例
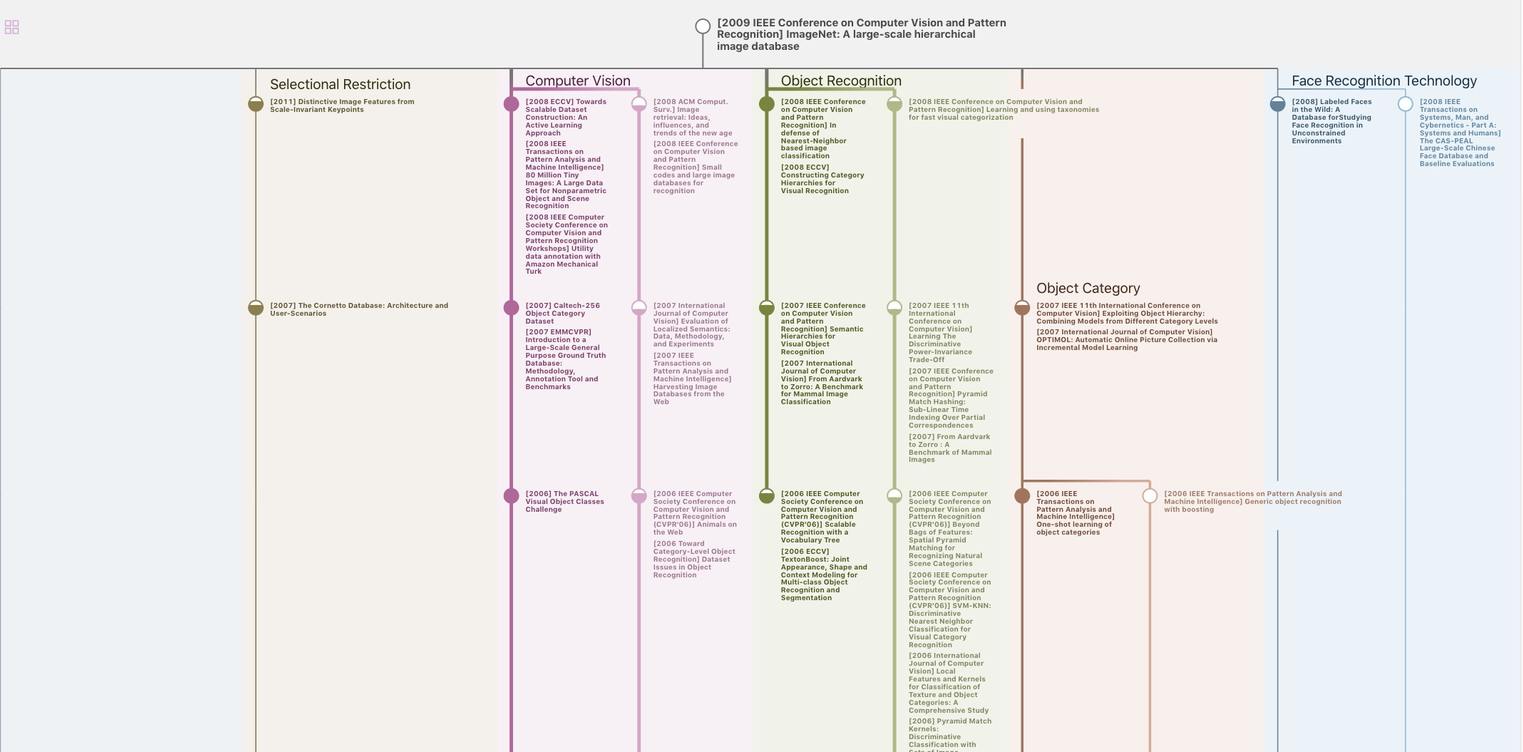
生成溯源树,研究论文发展脉络
Chat Paper
正在生成论文摘要