A Comparison of Machine Learning Models with Data Augmentation Techniques for Skeleton-based Human Action Recognition
14TH ACM CONFERENCE ON BIOINFORMATICS, COMPUTATIONAL BIOLOGY, AND HEALTH INFORMATICS, BCB 2023(2023)
摘要
3D skeleton motion recognition plays a crucial role in the field of human action recognition (HAR) due to its efficiency and reliability. This paper introduces data augmentation techniques applied to skeleton data with the aim of improving the accuracy of machine learning models and examining the effectiveness of different augmentation methods for different activities. Spatial transformations are applied to generate augmented samples from the original 3D skeleton sequences, while temporal augmentation techniques are employed to capture the temporal differences in motion. We evaluated the effects of spatial and temporal data augmentation at different levels on the MSR Daily Activity 3D dataset using SVM, CNN, LSTM, and CNNLSTM models. The results show that temporal augmentation significantly improves model performance, while spatial augmentation has only a limited effect on model performance. Simultaneously applying both spatial and temporal augmentation further improved model performance, highlighting the importance of temporal information in action sequence data.
更多查看译文
关键词
Data augmentation,Skeleton-based Human Action Recognition,SVM,CNN,LSTM,CNNLSTM
AI 理解论文
溯源树
样例
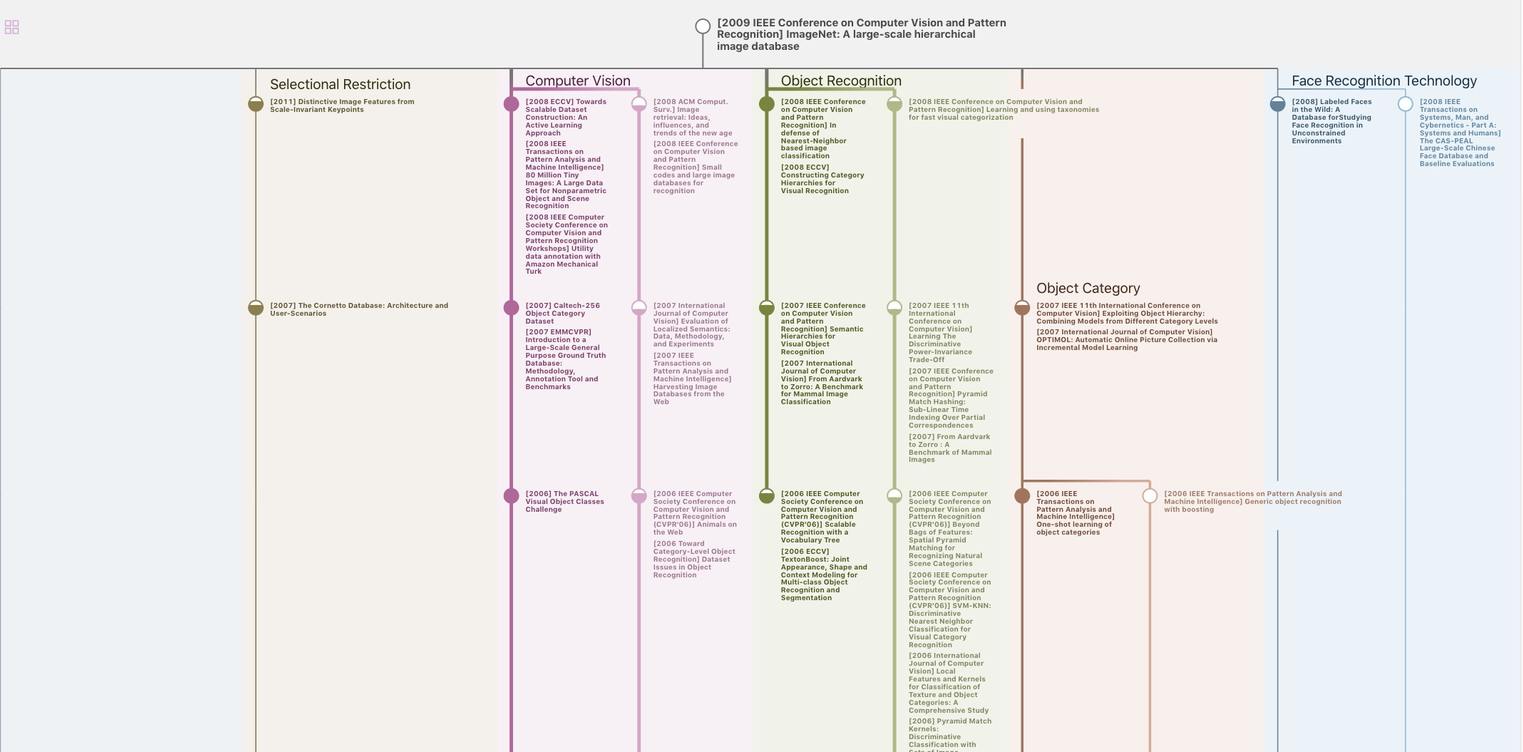
生成溯源树,研究论文发展脉络
Chat Paper
正在生成论文摘要