An incremental clustering method based on multiple objectives for dynamic data analysis
Multimedia Tools and Applications(2023)
摘要
Due to the advancement in big data and bioinformatics, the quantity and quality of raw data have exploded during the past two decades. Multiple sources contributed to the generation of very complex, diverse, and vast raw data. The generated data may conceal crucial patterns that need to be identified for data analysis. In the past few decades, a variety of clustering methods have been developed and have proven useful for data analysis. However, these methods are inappropriate for dynamic applications and only function with static data. To address this issue, we present a multi-objective incremental clustering method for processing dynamic data that generates and updates clusters in real-time. To improve the dynamic clustering process, the proposed method employs Euclidean distance to calculate the similarity between data points and constructs a fitness function with three primary clustering objective functions: inter-cluster distance, intra-cluster distance, and cluster density. The proposed method employs the concept of objective weighting, which allocates a weight to each objective in order to generate a single Pareto-optimal solution for the constructed fitness function. The proposed method outperforms other state-of-the-art methods on five benchmarks and three real-life plant genomics data sets.
更多查看译文
关键词
Multi-objective optimization,Incremental clustering,Intra-cluster distance,Inter-cluster distance,Cluster density
AI 理解论文
溯源树
样例
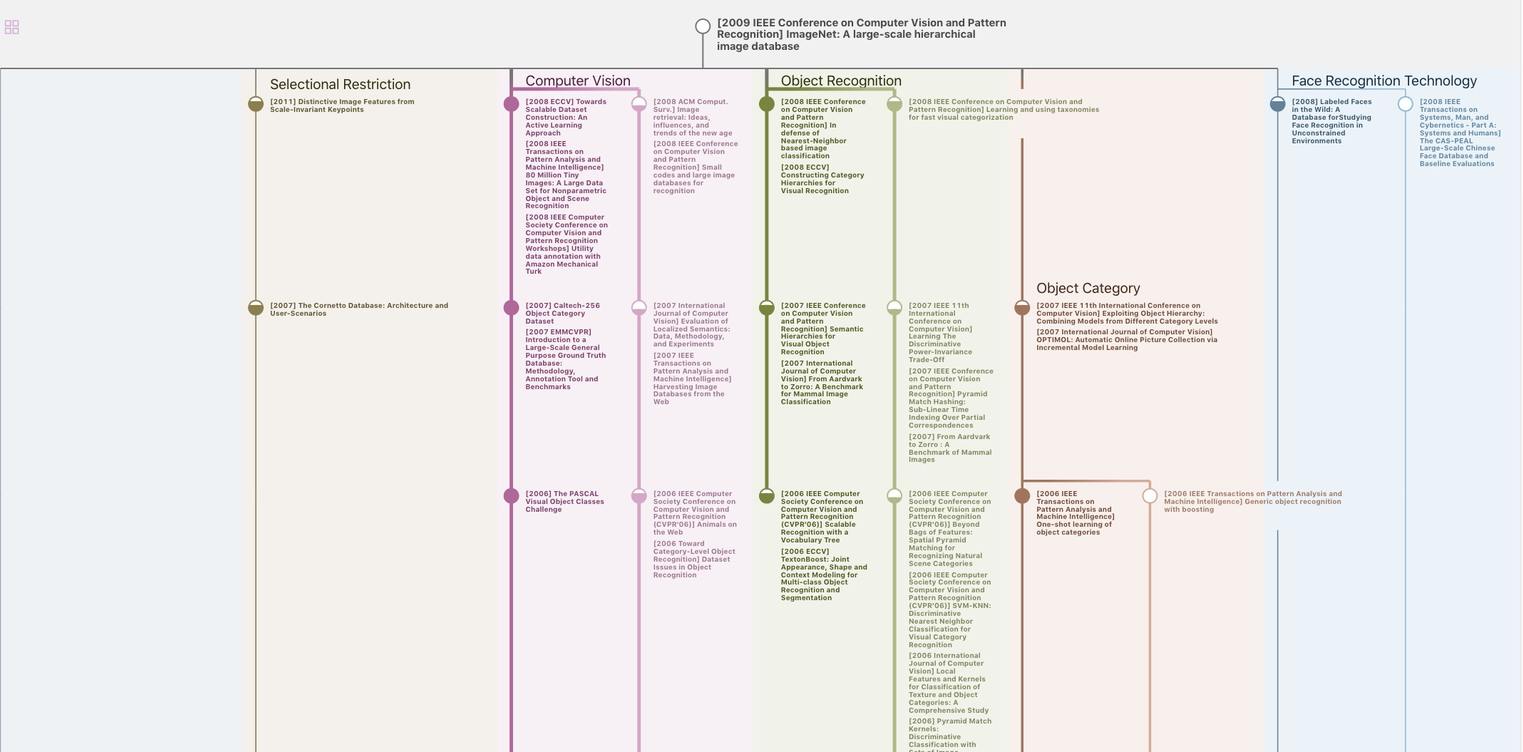
生成溯源树,研究论文发展脉络
Chat Paper
正在生成论文摘要