Towards prognostic generalization: a domain conditional invariance and specificity disentanglement network for remaining useful life prediction
Journal of Intelligent Manufacturing(2023)
摘要
Remaining useful life (RUL) prediction is an essential task in ensuring reliability in intelligent manufacturing. Recent advances in deep learning-based data-driven methods have shown promising results. However, one non-ignorable challenge is that distribution shift across various machine individuals often results in a performance decline. Domain adaptation approaches appear to be effective in tackling this issue, whereas they often require sufficient unlabeled target data, which is causally infeasible in prognostic tasks in practical scenarios. In this paper, we discuss the significance of prognostic generalization for RUL prediction, and a domain generalization-based scheme is proposed. A domain conditional invariance and specificity disentanglement network (DCISD) is proposed to learn domain conditional-invariant and domain-specific information simultaneously in a unified network. Domain conditional-invariant features are extracted through conditional domain adversarial learning and samples are conditioned by multiple RUL fuzzy sets. Domain-specific features correlated to individual degradation patterns are disentangled to promote sufficiency of degradation information. Moreover, a degradation dynamics-based augmentation method is proposed to mitigate domain imbalance following the degradation dynamics in the latent space. Two bearing run-to-failure datasets are utilized to evaluate the proposed method. Comparative and ablation studies validate the method effectiveness and superiority.
更多查看译文
关键词
Prognostic generalization,Domain generalization,RUL prediction,Feature disentanglement
AI 理解论文
溯源树
样例
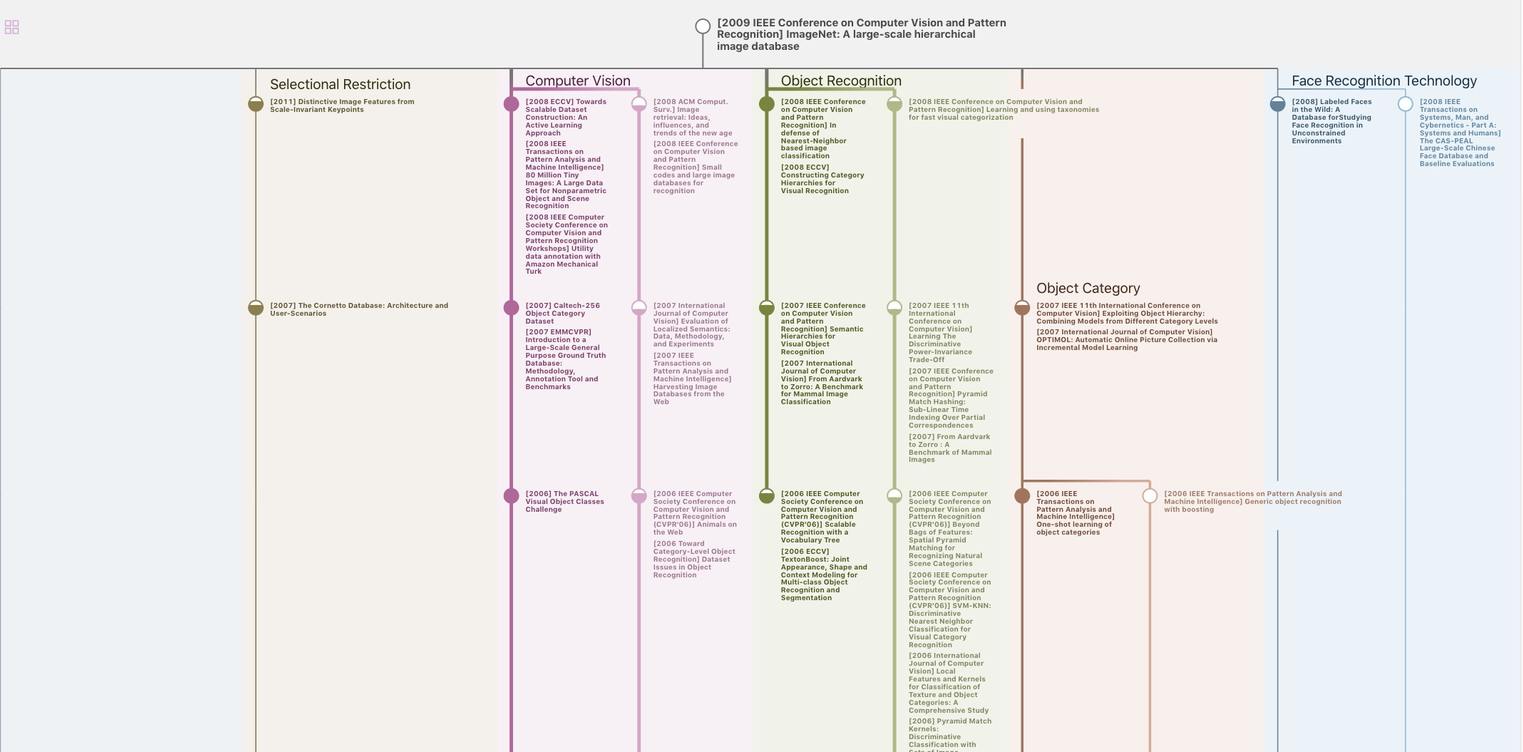
生成溯源树,研究论文发展脉络
Chat Paper
正在生成论文摘要