An early-stage diagnosis of diabetic retinopathy based on ensemble framework
Signal, Image and Video Processing(2023)
摘要
Diabetic retinopathy (DR) is one of the most common causes of vision loss worldwide. Early identification and detection of DR, aided by a retinal fundus scan, can prevent the disease’s onset and progression, lowering the risk of blindness in diabetic individuals. Over the years, ophthalmologists have relied on the time-consuming technique of manually screening for DR, which is also prone to erroneous results. Therefore, automated artificial intelligence approaches have become one of the most effective diagnostic technologies for validating ophthalmologists’ judgments. In this research, we present a binary classification of DR, which classifies healthy (not infected with DR) and DR eyes, based on ensemble deep learning approaches. The result is validated using an ensemble framework of five different models: VGG19, ResNet50, DenseNet121, InceptionV3, and EfficientNetB6. Eyepacs and Aptos fundus image datasets have been used to train, validate, and test the models. Additionally, the Messidor-2 fundus image dataset has also been used for external validation. Various preprocessing techniques, data augmentation, and hyperparameter tuning have been applied to improve the network performance of the models. The introduction of EfficientNetB6 within the ensemble framework performs better than the other models, with a train accuracy of 0.901, validation accuracy of 0.977, test accuracy of 0.984, and Cohen kappa score of 0.97.
更多查看译文
关键词
Diabetic retinopathy,Fundus image,Deep learning,Transfer learning,EfficientNet,Ensemble framework
AI 理解论文
溯源树
样例
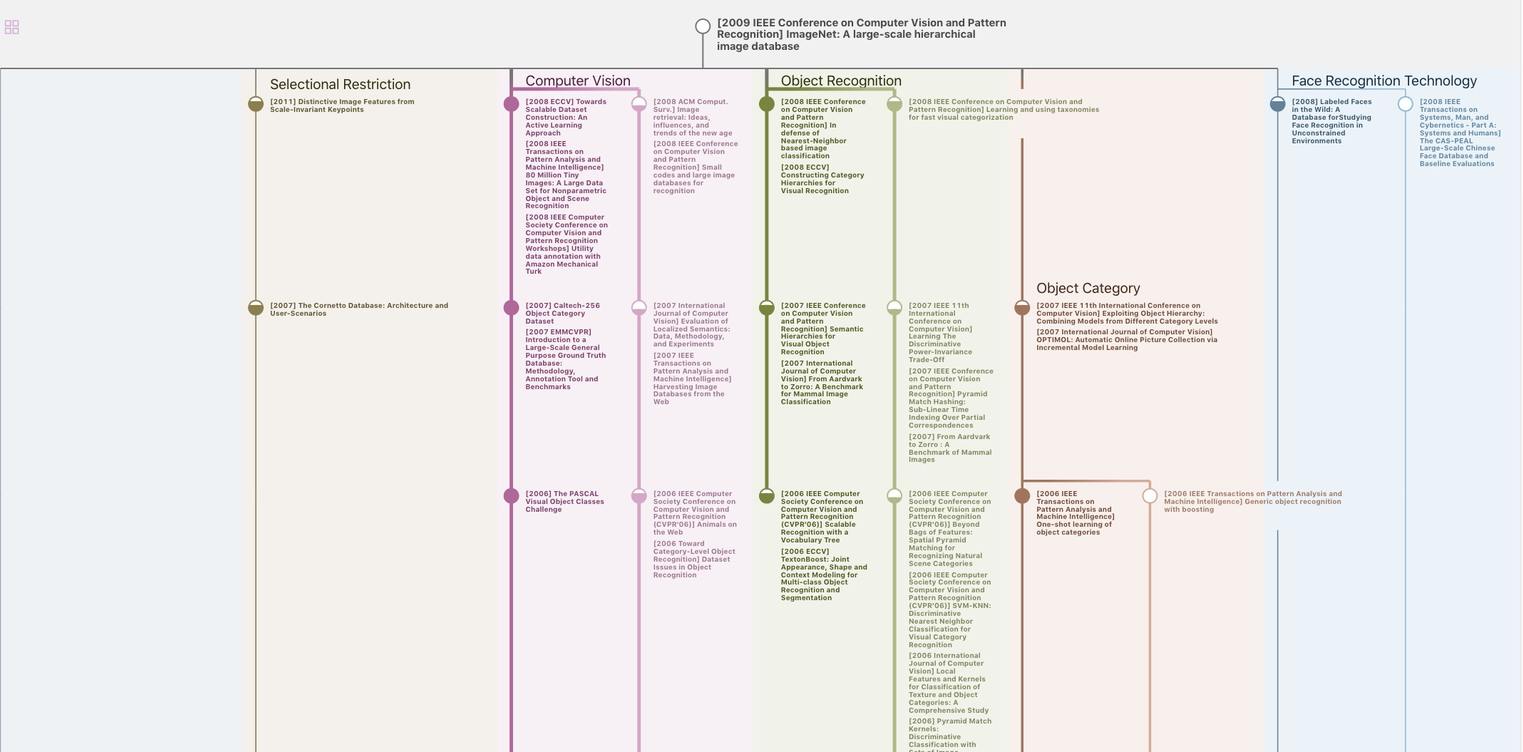
生成溯源树,研究论文发展脉络
Chat Paper
正在生成论文摘要