VeriDIP: Verifying Ownership of Deep Neural Networks through Privacy Leakage Fingerprints
CoRR(2023)
摘要
Deploying Machine Learning as a Service gives rise to model plagiarism, leading to copyright infringement. Ownership testing techniques are designed to identify model fingerprints for verifying plagiarism. However, previous works often rely on overfitting or robustness features as fingerprints, lacking theoretical guarantees and exhibiting under-performance on generalized models. In this paper, we propose a novel ownership testing method called VeriDIP, which verifies a DNN model's intellectual property. VeriDIP makes two major contributions. (1) It utilizes membership inference attacks to estimate the lower bound of privacy leakage, which reflects the fingerprint of a given model. The privacy leakage fingerprints highlight the unique patterns through which the models memorize sensitive training datasets. (2) We introduce a novel approach using less private samples to enhance the performance of ownership testing. Extensive experimental results confirm that VeriDIP is effective and efficient in validating the ownership of deep learning models trained on both image and tabular datasets. VeriDIP achieves comparable performance to state-of-the-art methods on image datasets while significantly reducing computation and communication costs. Enhanced VeriDIP demonstrates superior verification performance on generalized deep learning models, particularly on table-trained models. Additionally, VeriDIP exhibits similar effectiveness on utility-preserving differentially private models compared to non-differentially private baselines.
更多查看译文
关键词
Fingerprinting,neural networks,ownership protection,membership inference,differential privacy
AI 理解论文
溯源树
样例
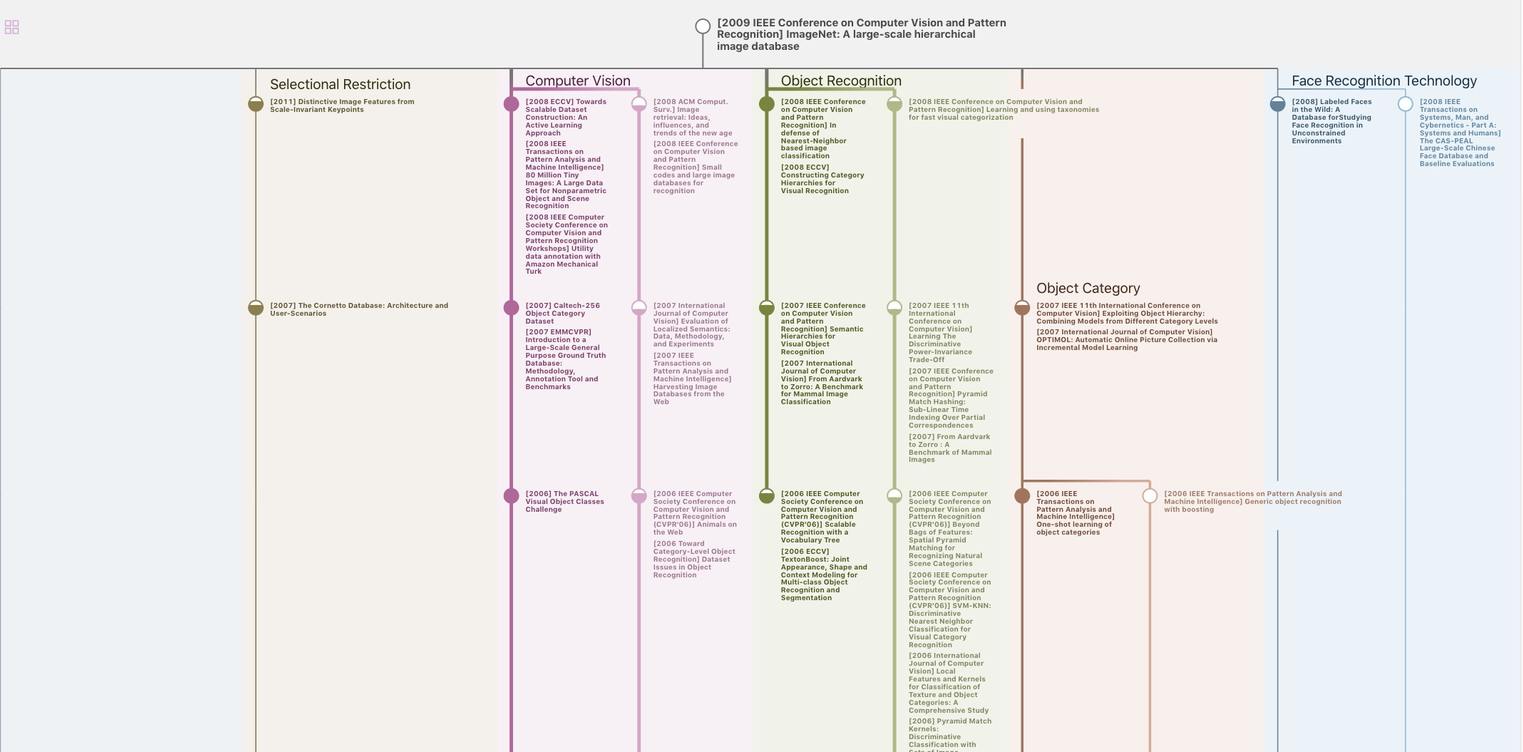
生成溯源树,研究论文发展脉络
Chat Paper
正在生成论文摘要