BayesDiff: Estimating Pixel-wise Uncertainty in Diffusion via Bayesian Inference
arXiv (Cornell University)(2023)
摘要
Diffusion models have impressive image generation capability, but low-quality
generations still exist, and their identification remains challenging due to
the lack of a proper sample-wise metric. To address this, we propose BayesDiff,
a pixel-wise uncertainty estimator for generations from diffusion models based
on Bayesian inference. In particular, we derive a novel uncertainty iteration
principle to characterize the uncertainty dynamics in diffusion, and leverage
the last-layer Laplace approximation for efficient Bayesian inference. The
estimated pixel-wise uncertainty can not only be aggregated into a sample-wise
metric to filter out low-fidelity images but also aids in augmenting successful
generations and rectifying artifacts in failed generations in text-to-image
tasks. Extensive experiments demonstrate the efficacy of BayesDiff and its
promise for practical applications.
更多查看译文
关键词
diffusion,uncertainty,bayesian,pixel-wise
AI 理解论文
溯源树
样例
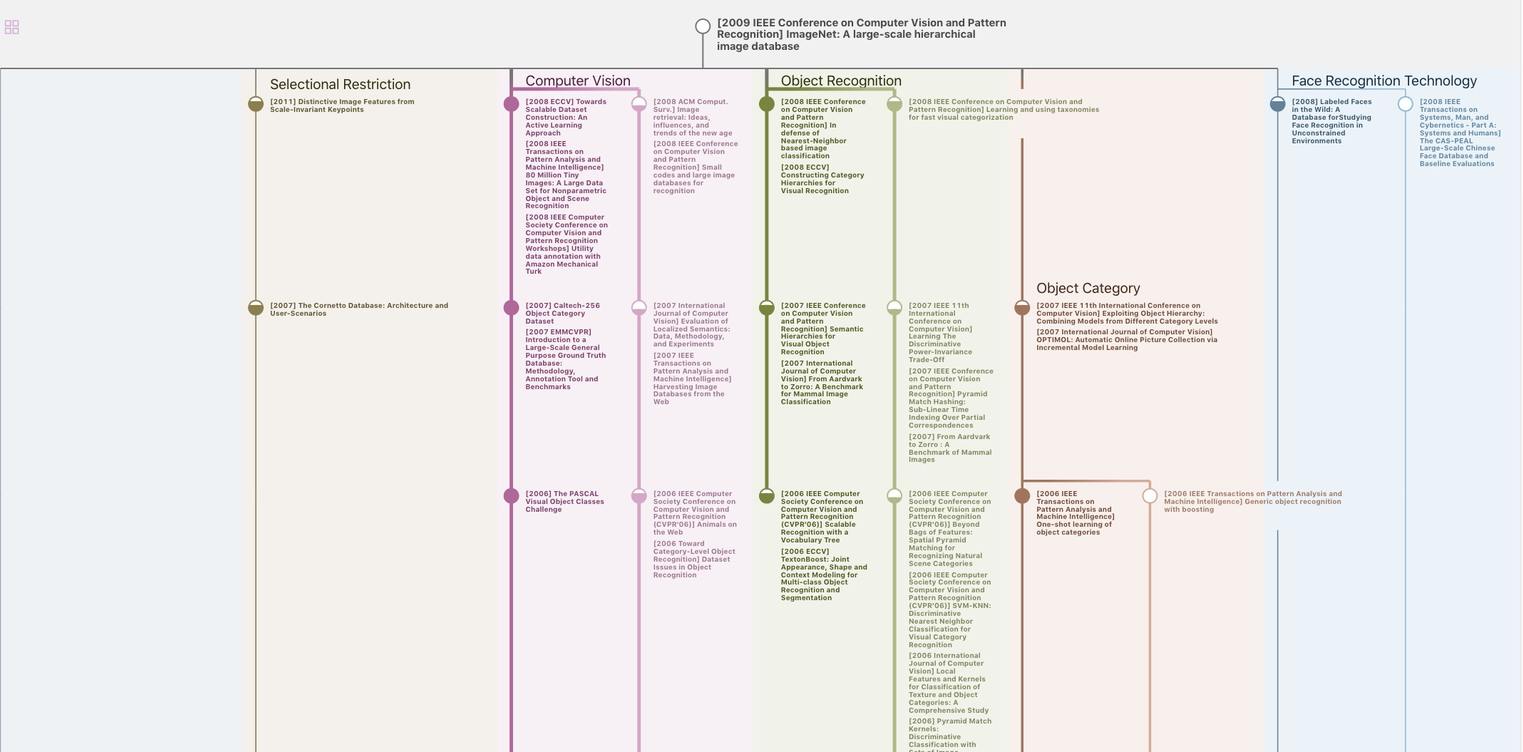
生成溯源树,研究论文发展脉络
Chat Paper
正在生成论文摘要