Uncertainty Quantification For Turbulent Flows with Machine Learning
arXiv (Cornell University)(2023)
摘要
Turbulent flows are of central importance across applications in science and engineering problems. For design and analysis, scientists and engineers use Computational Fluid Dynamics (CFD) simulations using turbulence models. Turbulent models are limited approximations, introducing epistemic uncertainty in CFD results. For reliable design and analysis, we require quantification of these uncertainties. The Eigenspace Perturbation Method (EPM) is the preeminent physics based approach for turbulence model UQ, but often leads to overly conservative uncertainty bounds. In this study, we use Machine Learning (ML) models to moderate the EPM perturbations and introduce our physics constrained machine learning framework for turbulence model UQ. We test this framework in multiple problems to show that it leads to improved calibration of the uncertainty estimates.
更多查看译文
关键词
turbulent flows,uncertainty,machine learning,quantification
AI 理解论文
溯源树
样例
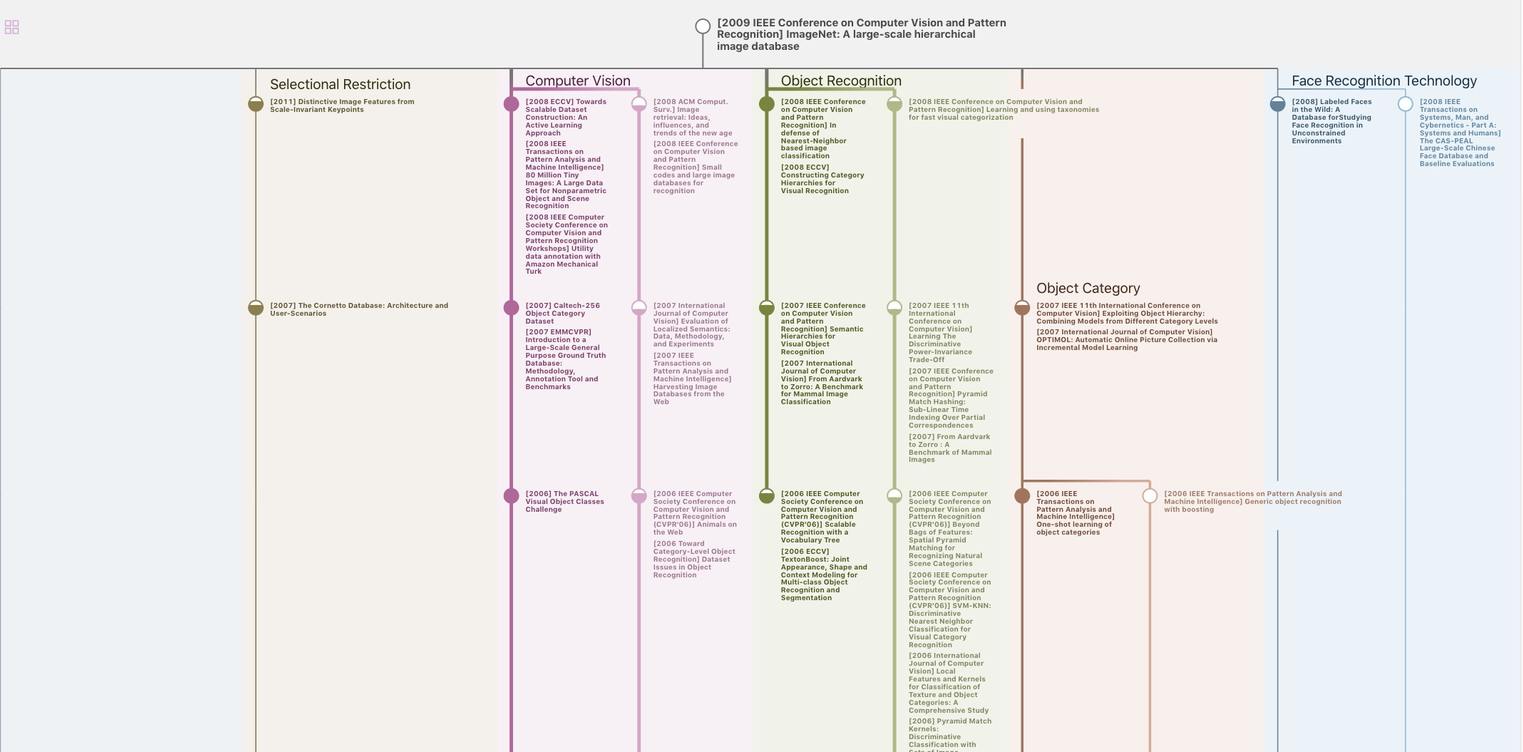
生成溯源树,研究论文发展脉络
Chat Paper
正在生成论文摘要