TK-KNN: A Balanced Distance-Based Pseudo Labeling Approach for Semi-Supervised Intent Classification.
CoRR(2023)
摘要
The ability to detect intent in dialogue systems has become increasingly important in modern technology. These systems often generate a large amount of unlabeled data, and manually labeling this data requires substantial human effort. Semi-supervised methods attempt to remedy this cost by using a model trained on a few labeled examples and then by assigning pseudo-labels to further a subset of unlabeled examples that has a model prediction confidence higher than a certain threshold. However, one particularly perilous consequence of these methods is the risk of picking an imbalanced set of examples across classes, which could lead to poor labels. In the present work, we describe Top-K K-Nearest Neighbor (TK-KNN), which uses a more robust pseudo-labeling approach based on distance in the embedding space while maintaining a balanced set of pseudo-labeled examples across classes through a ranking-based approach. Experiments on several datasets show that TK-KNN outperforms existing models, particularly when labeled data is scarce on popular datasets such as CLINC150 and Banking77. Code is available at https://github.com/ServiceNow/tk-knn
更多查看译文
关键词
pseudo labeling approach,classification,tk-knn,distance-based,semi-supervised
AI 理解论文
溯源树
样例
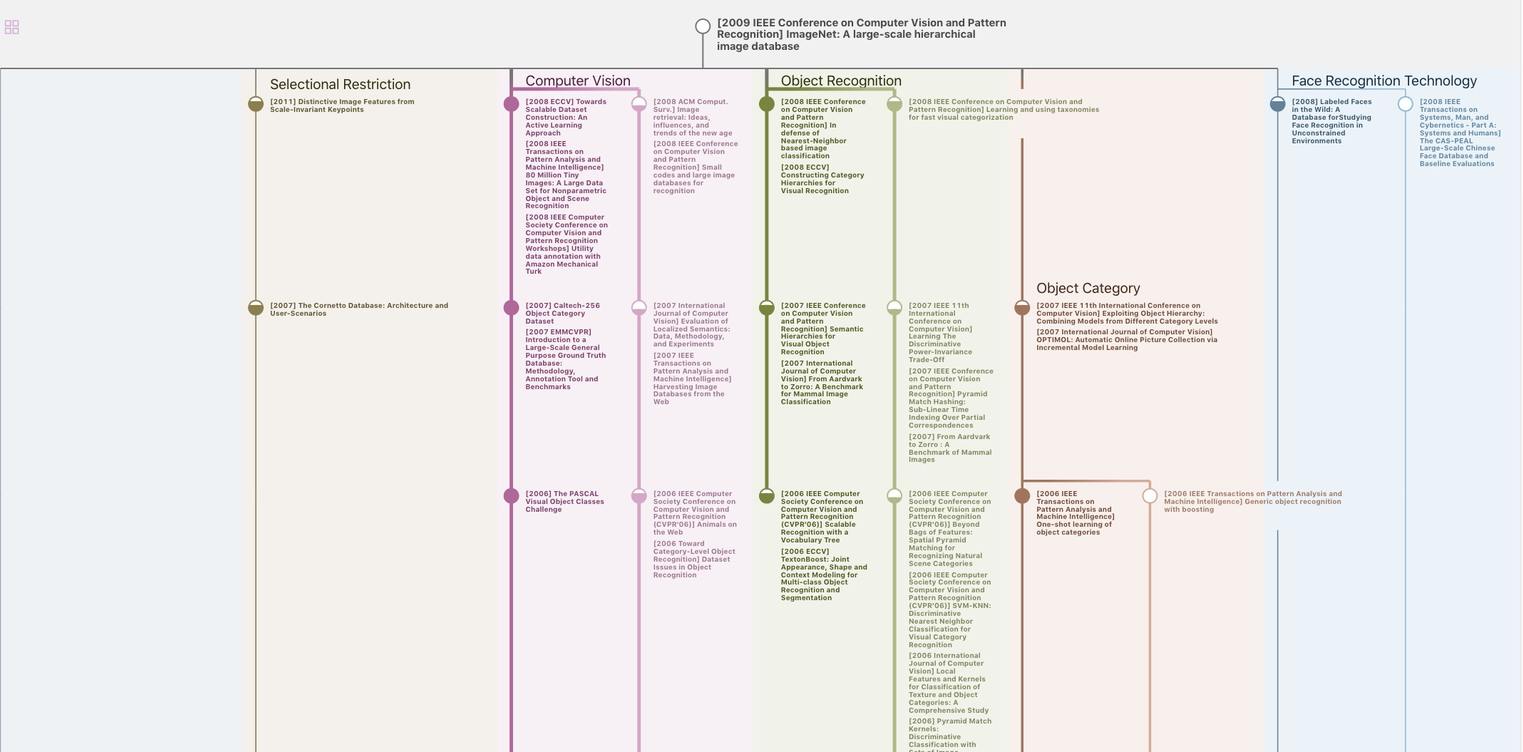
生成溯源树,研究论文发展脉络
Chat Paper
正在生成论文摘要