Using Experience Classification for Training Non-Markovian Tasks
CoRR(2023)
摘要
Unlike the standard Reinforcement Learning (RL) model, many real-world tasks are non-Markovian, whose rewards are predicated on state history rather than solely on the current state. Solving a non-Markovian task, frequently applied in practical applications such as autonomous driving, financial trading, and medical diagnosis, can be quite challenging. We propose a novel RL approach to achieve non-Markovian rewards expressed in temporal logic LTL$_f$ (Linear Temporal Logic over Finite Traces). To this end, an encoding of linear complexity from LTL$_f$ into MDPs (Markov Decision Processes) is introduced to take advantage of advanced RL algorithms. Then, a prioritized experience replay technique based on the automata structure (semantics equivalent to LTL$_f$ specification) is utilized to improve the training process. We empirically evaluate several benchmark problems augmented with non-Markovian tasks to demonstrate the feasibility and effectiveness of our approach.
更多查看译文
关键词
experience classification,training,non-markovian
AI 理解论文
溯源树
样例
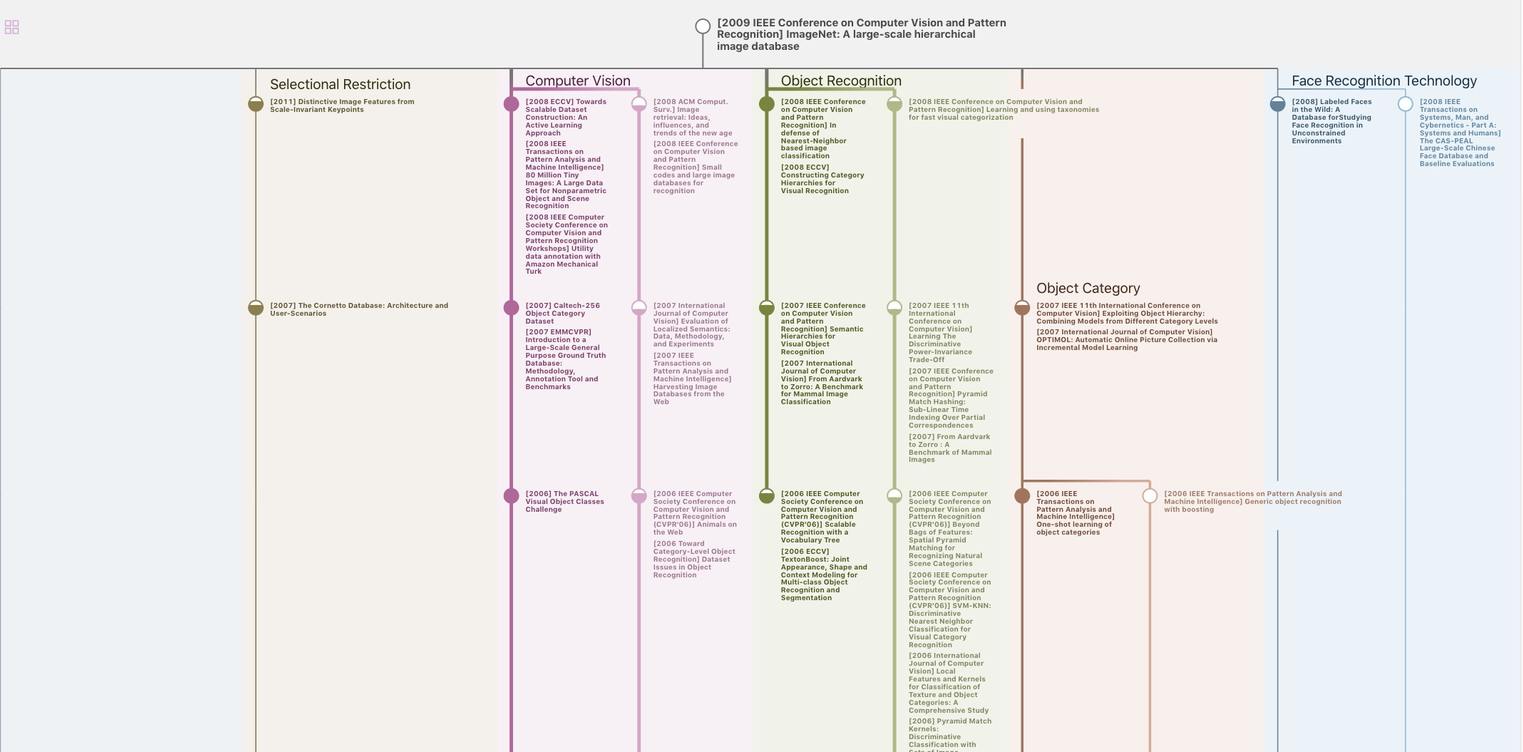
生成溯源树,研究论文发展脉络
Chat Paper
正在生成论文摘要