Quantifying Self-diagnostic Atomic Knowledge in Chinese Medical Foundation Model: A Computational Analysis
arxiv(2023)
摘要
Foundation Models (FMs) have the potential to revolutionize the way users
self-diagnose through search engines by offering direct and efficient
suggestions. Recent studies primarily focused on the quality of FMs evaluated
by GPT-4 or their ability to pass medical exams, no studies have quantified the
extent of self-diagnostic atomic knowledge stored in FMs' memory, which is the
basis of foundation models to provide factual and reliable suggestions. In this
paper, we first constructed a benchmark of Self-diagnostic Atomic Knowledge
(SdAK), including the most common types of atomic knowledge involved in
self-diagnostic queries, with 17 atomic types and a total of 14, 048 pieces of
atomic knowledge. Then, we evaluated both generic and open-source Chinese
medical FMs on the benchmark. The experimental results showcase that generic
FMs perform better than medical FMs in terms of self-diagnostic atomic
knowledge. Error analysis revealed that both generic and medical FMs are
sycophantic, e.g., always catering to users' claims when it comes to unknown
knowledge. We further explored different types of data commonly adopted for
fine-tuning medical FMs, i.e., real-world, semi-distilled, and distilled data,
and found that distilled data can benefit FMs most. The code and data are
available at https://github.com/FreedomIntelligence/SDAK.
更多查看译文
AI 理解论文
溯源树
样例
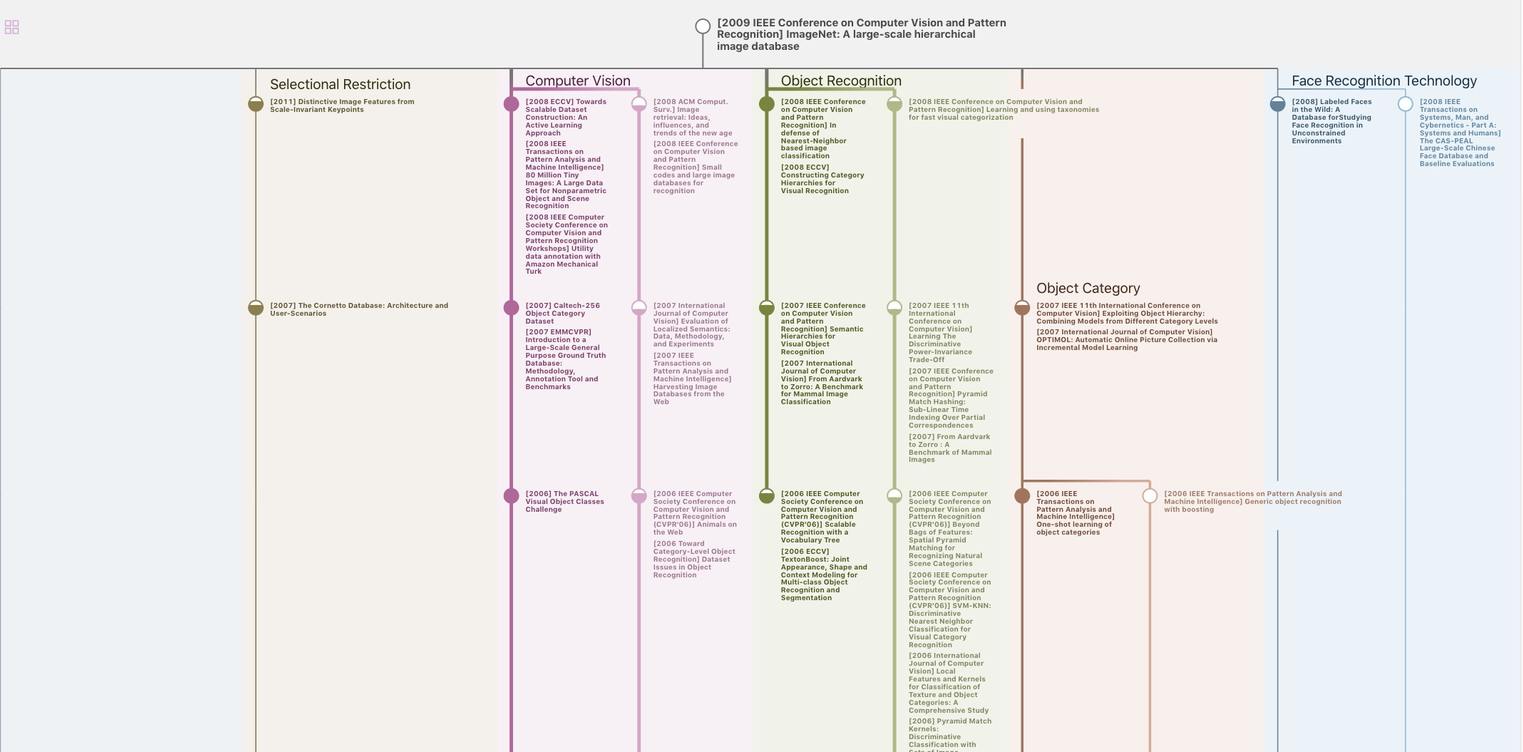
生成溯源树,研究论文发展脉络
Chat Paper
正在生成论文摘要