Post-clustering Inference under Dependency
arXiv (Cornell University)(2023)
摘要
Recent work by Gao et al. has laid the foundations for post-clustering inference. For the first time, the authors established a theoretical framework allowing to test for differences between means of estimated clusters. Additionally, they studied the estimation of unknown parameters while controlling the selective type I error. However, their theory was developed for independent observations identically distributed as $p$-dimensional Gaussian variables with a spherical covariance matrix. Here, we aim at extending this framework to a more convenient scenario for practical applications, where arbitrary dependence structures between observations and features are allowed. We show that a $p$-value for post-clustering inference under general dependency can be defined, and we assess the theoretical conditions allowing the compatible estimation of a covariance matrix. The theory is developed for hierarchical agglomerative clustering algorithms with several types of linkages, and for the $k$-means algorithm. We illustrate our method with synthetic data and real data of protein structures.
更多查看译文
关键词
inference,dependency,post-clustering
AI 理解论文
溯源树
样例
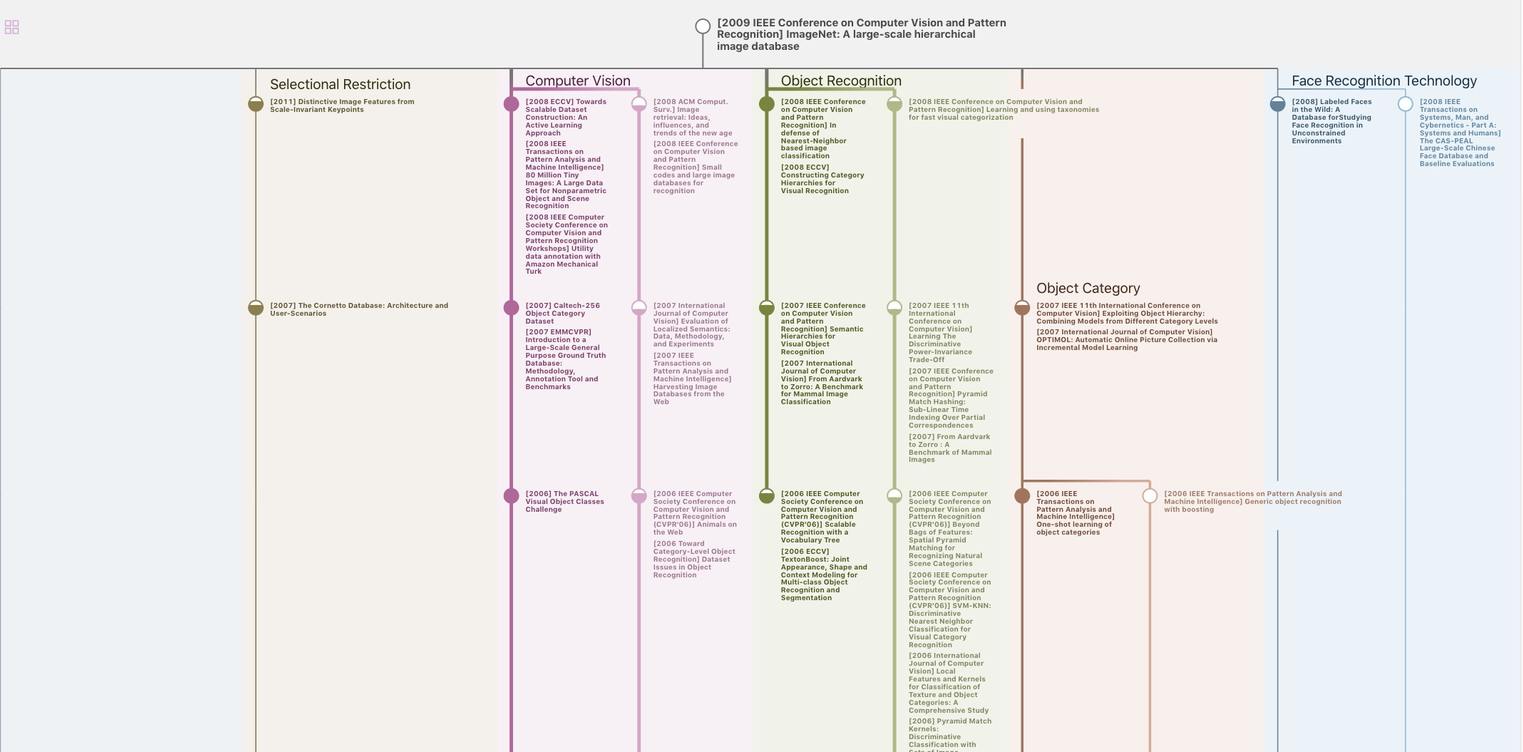
生成溯源树,研究论文发展脉络
Chat Paper
正在生成论文摘要