Online Estimation with Rolling Validation: Adaptive Nonparametric Estimation with Streaming Data
arxiv(2023)
摘要
Online nonparametric estimators are gaining popularity due to their efficient
computation and competitive generalization abilities. An important example
includes variants of stochastic gradient descent. These algorithms often take
one sample point at a time and instantly update the parameter estimate of
interest. In this work we consider model selection and hyperparameter tuning
for such online algorithms. We propose a weighted rolling-validation procedure,
an online variant of leave-one-out cross-validation, that costs minimal extra
computation for many typical stochastic gradient descent estimators. Similar to
batch cross-validation, it can boost base estimators to achieve a better,
adaptive convergence rate. Our theoretical analysis is straightforward, relying
mainly on some general statistical stability assumptions. The simulation study
underscores the significance of diverging weights in rolling validation in
practice and demonstrates its sensitivity even when there is only a slim
difference between candidate estimators.
更多查看译文
AI 理解论文
溯源树
样例
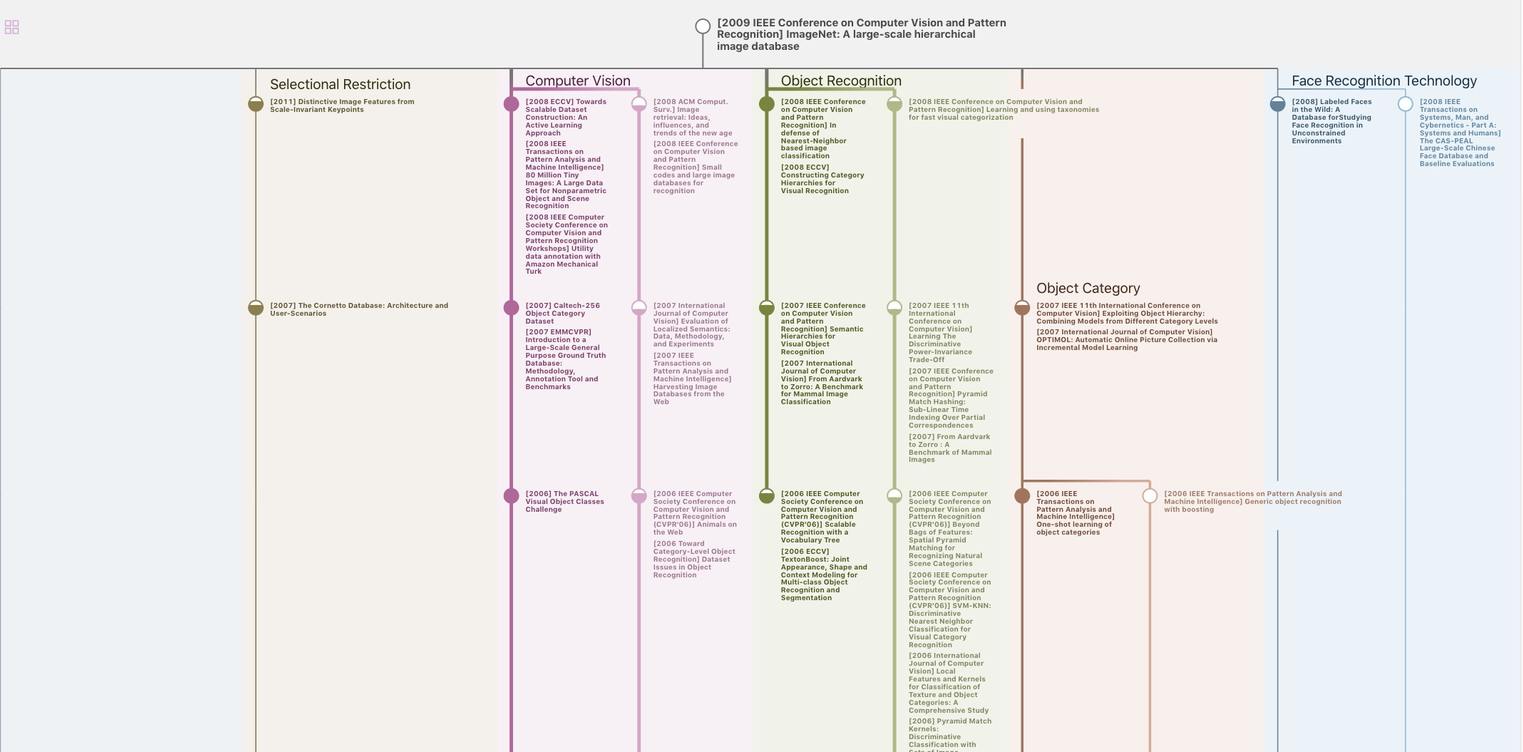
生成溯源树,研究论文发展脉络
Chat Paper
正在生成论文摘要