Accelerated feasible screening of flame-retardant polymeric composites using data-driven multi-objective optimization
Computational Materials Science(2023)
摘要
The design of flame-retardant polymeric composites (FRPC) with simultaneously satisfied requirements of multiple performances was traditionally costly in both time and economy. In this work, we performed data-driven multi-objective optimization (MOO) of the FRPC to accelerate the discoveries of high-performance composites. Consistent experimental datasets for each of the four target performances: limiting oxygen index, tensile strength, maximum specific optical density, and the total smoke release within the first four minutes, were created on 153 FRPC. Then, decision rules and approximate equations were found by using machine learning to predict the performances, and MOO was achieved by identifying the optimal composites that simultaneously satisfying the requirements by all these models. Considering a huge composition space (nearly 44 thousands of composites), MOO led to the discovery of 149 promising candidates. 10 of them were selected for experimental validation, and most of which were confirmed with excellent overall performances, demonstrating the efficiency of the approach in designing the FRPC for industrial applications.
更多查看译文
关键词
Multi-objective optimization, Flame-retardant polymeric composites, Machine learning, Data-driven science
AI 理解论文
溯源树
样例
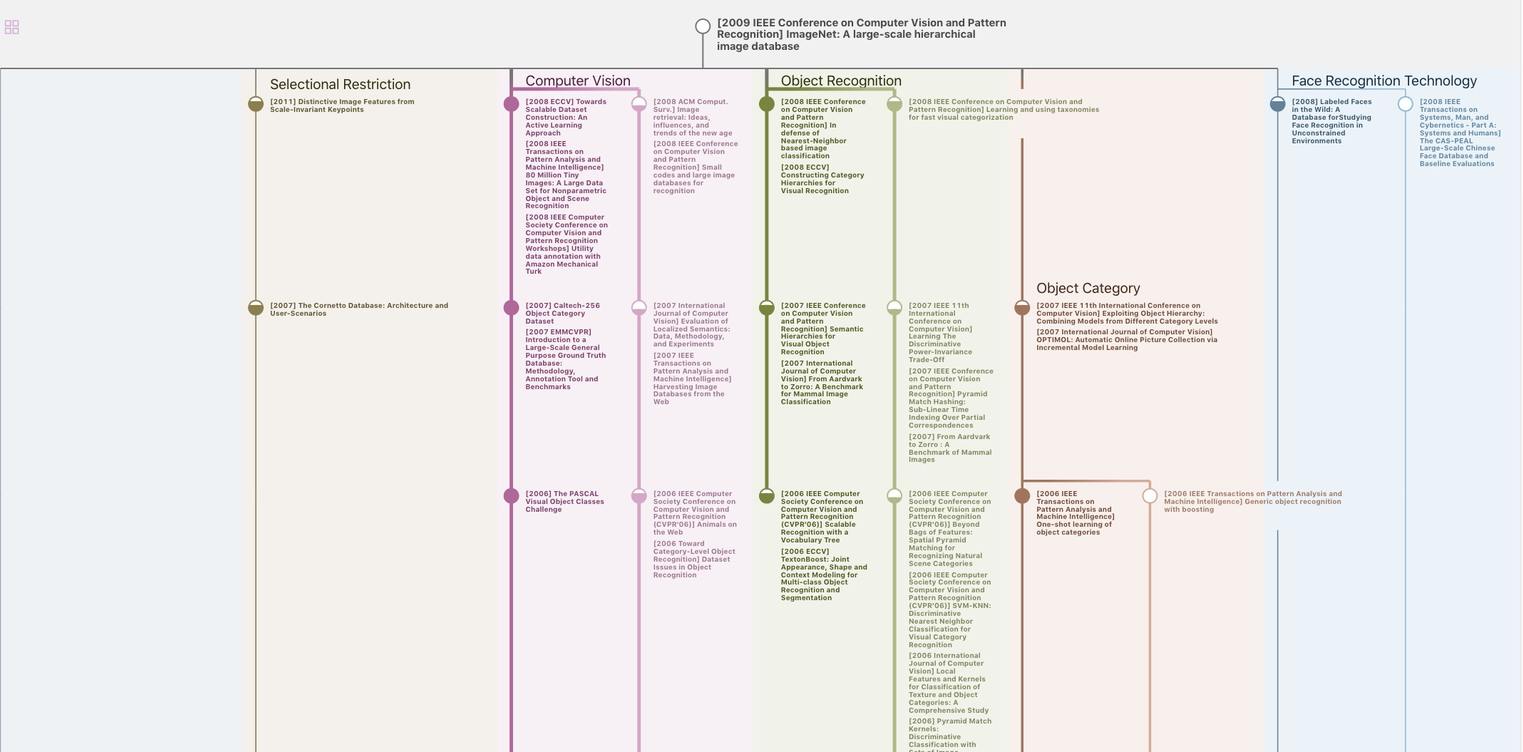
生成溯源树,研究论文发展脉络
Chat Paper
正在生成论文摘要