Machine learning-assisted extreme value statistics of anomalies in AlSi10Mg manufactured by L-PBF for robust fatigue strength predictions
MATERIALS & DESIGN(2023)
摘要
Traditional Extreme Value Statistics (EVS) applied to block maxima sampled anomalies of components produced by Laser-Powder Bed Fusion may produce important inaccuracies in the estimated characteristic defects. In fact, the typical presence of multiple defect types may significantly affect the fitted maxima distributions obtained from different sampling volumes. In this work, we show how the limitations of traditional EVS can be overcome by applying supervised machine learning (ML) algorithms to classify the defects before estimating the maxima distributions for each defect type. The ML-assisted EVS provided maxima distributions unaffected by the different sampling volumes. The obtained maxima distribution lead to robust estimates of exceedance curves and finally, by employing the Shiozawa curve, to robust fatigue strength predictions.
更多查看译文
关键词
Laser-powder bed fusion,Extreme value statistics,Machine learning,X-ray computed tomography,Fatigue
AI 理解论文
溯源树
样例
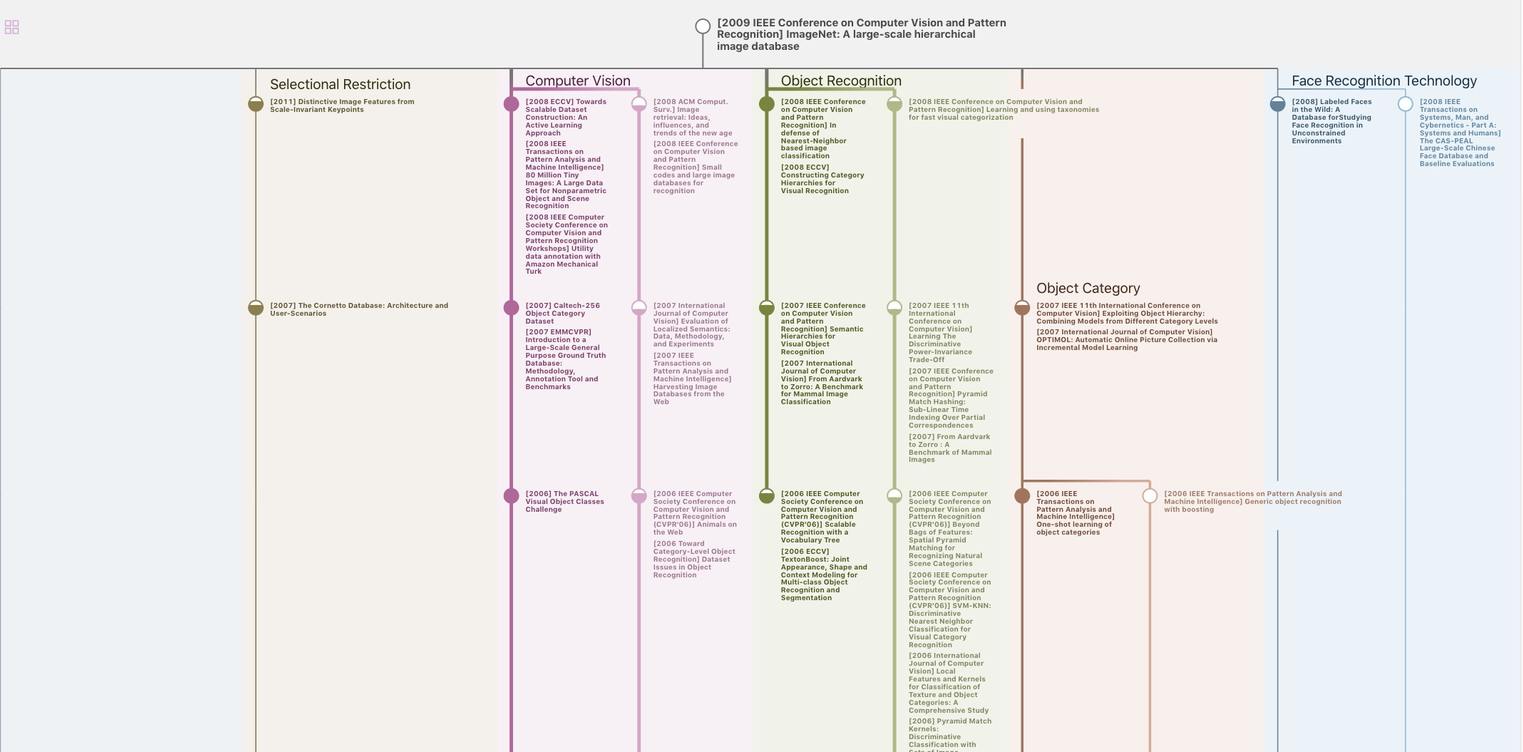
生成溯源树,研究论文发展脉络
Chat Paper
正在生成论文摘要