Comparative Performance Analysis of Machine Learning Algorithms for Arm and Shoulder Exercises Using Wrist-Worn Band
IEEE Access(2023)
摘要
The COVID-19 pandemic forcing many individuals to exercise at home without the supervision of a professional trainer, and maintaining proper posture during arm and shoulder exercises has become a concern. In this study, the dataset is collected from various individuals performing different exercises with different variations in the range of motion. The wrist-worn band captures the motion data using the MPU6050 sensor, which measures acceleration data. The collected data is pre-processed, and features are extracted from the raw data. Machine learning-based classification algorithms are employed to classify the collected data. An App prototype has been developed, which is integrated with a WIFI module and Cloud for real-time data collection. The collected data is then used to train the selected machine learning models, which can accurately analyze an individual’s workout session and provide feedback on their performance through the App. Overall, this methodology provides a solution for individuals to continue their regular exercises at home without the need for a professional gym trainer or environment while minimizing the risk of injury due to poor posture. The results of this study will provide insight into the effectiveness of different machine learning algorithms for arm and shoulder exercises using a wrist-worn band, which can help in designing personalized exercise programs for individuals. We applied four machine learning algorithms to the dataset which are weighted KNN, Bagged Trees, Decision Trees, and Fine Gaussian SVM. The experimental results show that the Weighted KNN Model gives the best accuracy of 92%.
更多查看译文
关键词
machine learning algorithms,shoulder exercises,machine learning,wrist-worn
AI 理解论文
溯源树
样例
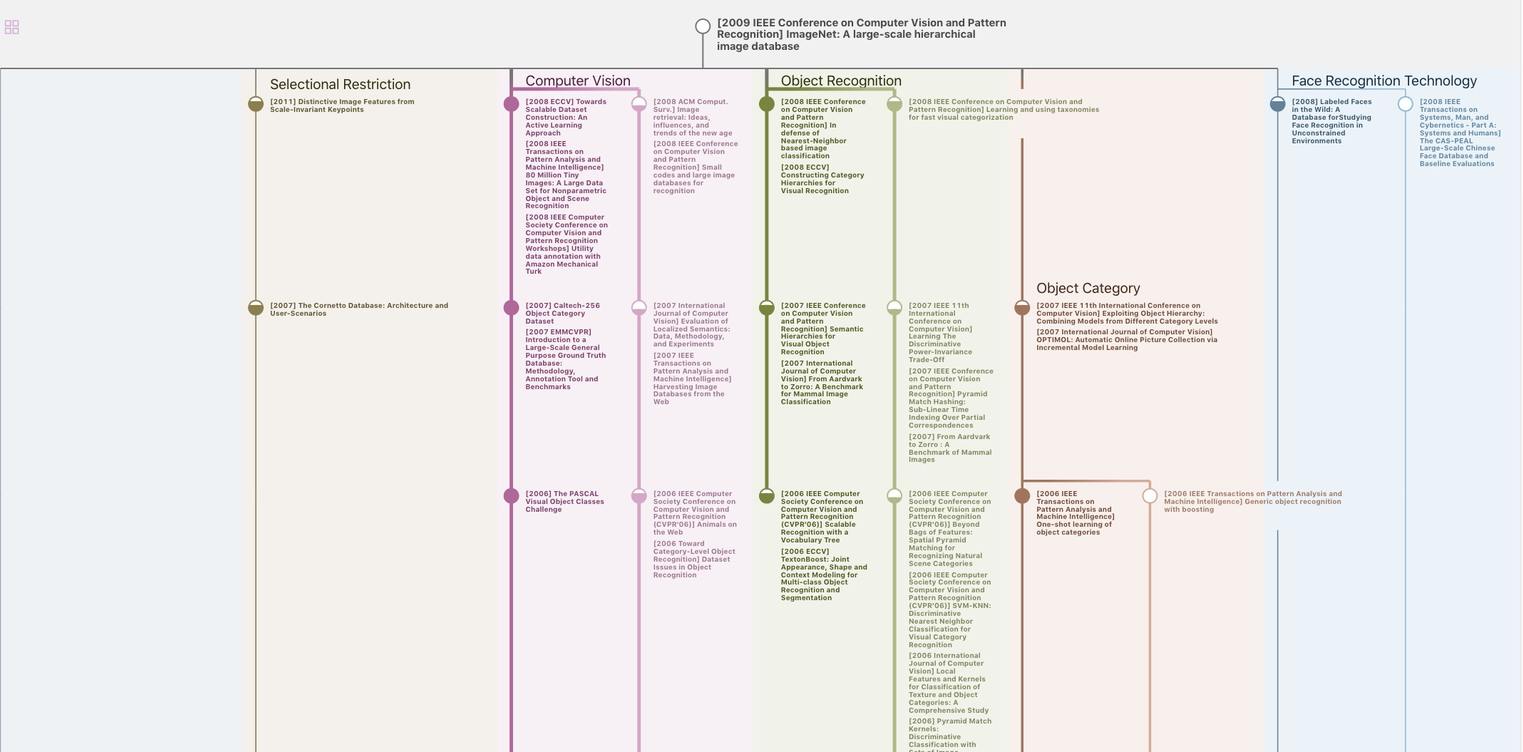
生成溯源树,研究论文发展脉络
Chat Paper
正在生成论文摘要