Assessing Urdu Language Processing Tools via Statistical and Outlier Detection Methods on Urdu Tweets
ACM Transactions on Asian and Low-Resource Language Information Processing(2023)
摘要
Text pre-processing is a crucial step in Natural Language Processing (NLP) applications, particularly for handling informal and noisy content on social media. Word-level tokenization plays a vital role in text preprocessing by removing stop words, filtering irrelevant characters, and retaining relevant tokens. These tokens are essential for constructing meaningful n-grams within advanced NLP frameworks used for data modeling. However, tokenization in low-resource languages like Urdu presents challenges due to language complexity and limited resources. Conventional space-based methods and direct application of language-specific tools often result in erroneous tokens in Urdu Language Processing (ULP). This hinders language models from effectively learning language-specific and domain-specific tokens, leading to sub-optimal results for downstream tasks such as aspect mining, topic modeling, and Named Entity Recognition (NER). To address this issue for Urdu, we have proposed a data pre-processing technique that detects outliers using the Inter-Quartile-Range (IQR) method and proposed normalization algorithms for creating useful lexicons in conjunction with existing technologies. We have collected approximately 50 million Urdu tweets using the Twitter API and conducted the performance analysis of existing language-specific tokenizers (Urduhack and Space-based tokenizer). Dataset variants were created based on the language-specific tokenizers, and we performed statistical analysis tests and visualization techniques to compare tokenization results before and after applying the proposed outlier detection and normalization method. Our findings highlighted the noticeable improvement in token size distributions, handling of informal language tokens, and misspelled and lengthy tokens. The Urduhack tokenizer combined with the proposed outlier detection and normalization yielded tokens with the best-fitted distribution in ULP. Its effectiveness has been evaluated through the task of topic modeling using Non-negative Matrix Factorization (NMF) and Latent Dirichlet allocation (LDA). The results demonstrated new and distinct topics using unigram features while achieving highly coherent topics when utilizing bigram features. For the traditional space-based method, the results consistently demonstrated improved coherence and precision scores. However, the NMF topic modeling with bigram features outperformed LDA topic modeling with bigram features.
更多查看译文
关键词
urdu language processing tools,outlier detection methods
AI 理解论文
溯源树
样例
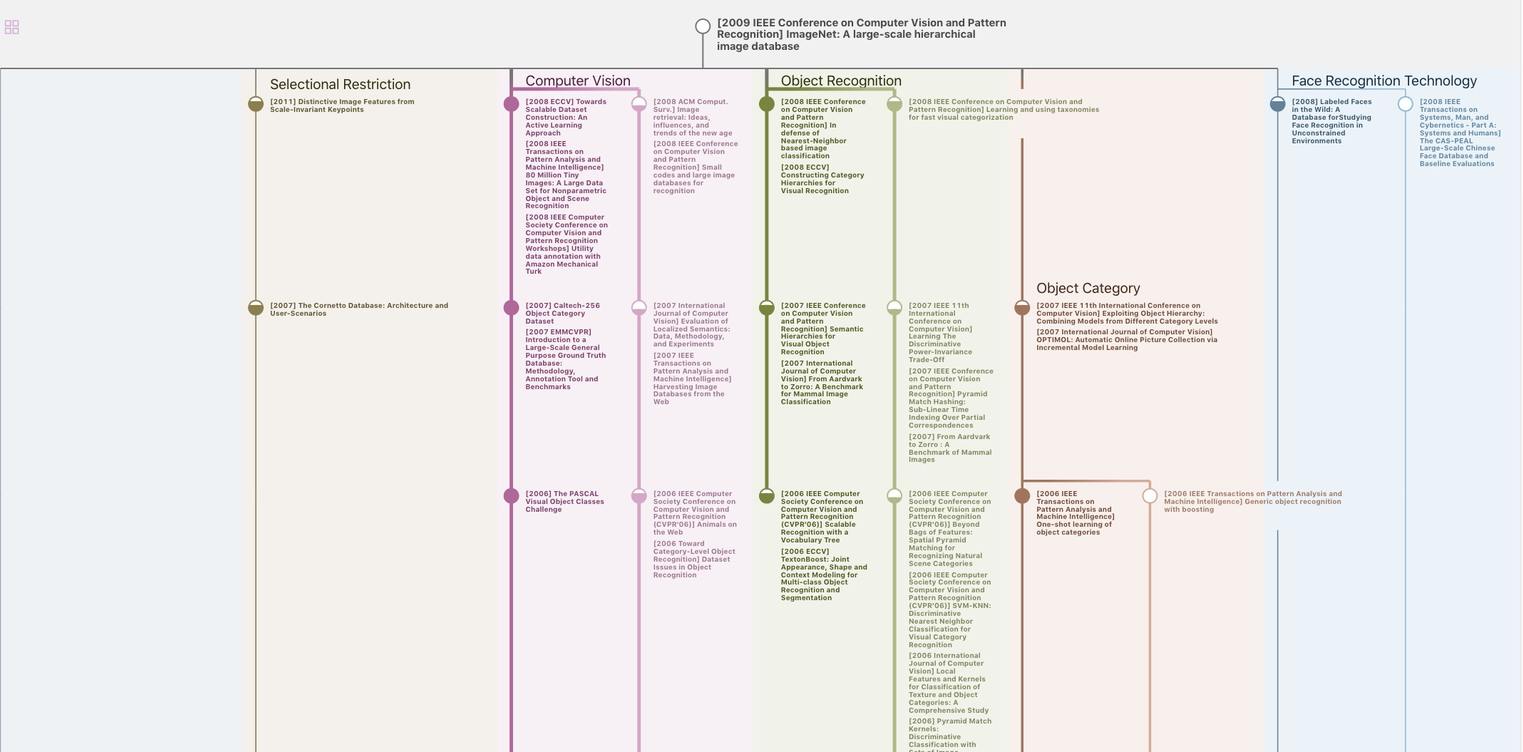
生成溯源树,研究论文发展脉络
Chat Paper
正在生成论文摘要