Evaluation of sleep stage classification using feature importance of EEG signal for big data healthcare
IJAIN (International Journal of Advances in Intelligent Informatics)(2023)
摘要
Sleep analysis is widely and experimentally considered due to its importance to body health care. Since its sufficiency is essential for a healthy life, people often spend almost a third of their lives sleeping. In this case, a similar sleep pattern is not practiced by every individual, regarding pure healthiness or disorders such as insomnia, apnea, bruxism, epilepsy, and narcolepsy. Therefore, this study aims to determine the classification patterns of sleep stages, using big data for health care. This used a high-dimensional FFT extraction algorithm, as well as a feature importance and tuning classifier, to develop accurate classification. The results showed that the proposed method led to more accurate classification than previous techniques. This was because the previous experiments had been conducted with the feature selection model, with accuracy implemented as a performance evaluation. Meanwhile, the EEG Sleep Stages classification model in this present report was composed of the feature selection and importance of the extraction stage. The previous and present experiments also reached the highest values of accuracy, with the Random Forest and SVM models using 2000 and 3000 features (87.19% and 89.19%, respectively. In this article, we proposed an analysis that the feature importance subsequently influenced the model's accuracy. This was because the proposed method was easily fine-tuned and optimized for each subject to improve sensitivity and reduce false negative occurrences.
更多查看译文
关键词
sleep stage classification,eeg signal,big data
AI 理解论文
溯源树
样例
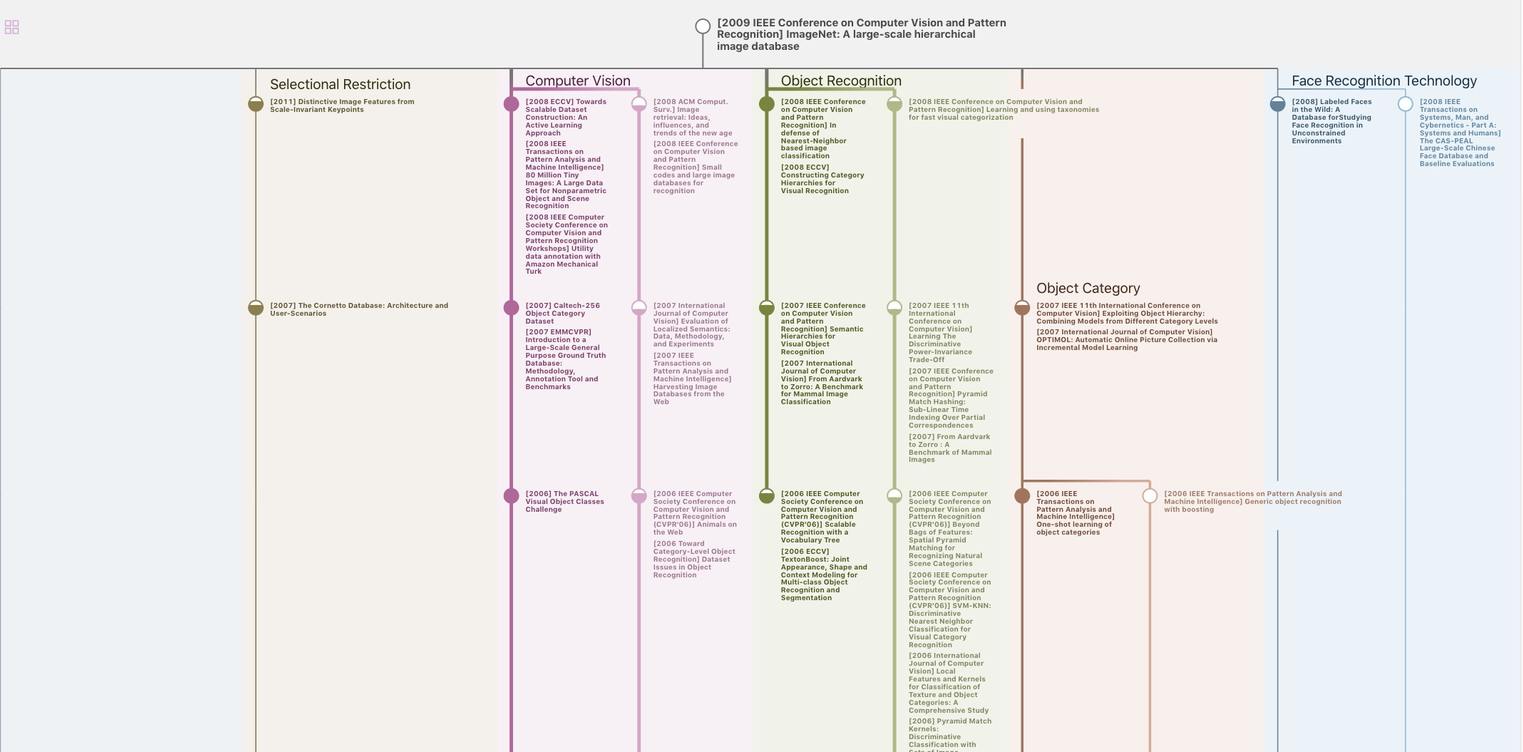
生成溯源树,研究论文发展脉络
Chat Paper
正在生成论文摘要