Ensemble Forecast Sensitivity to Observations Impact (EFSOI) applied to a regional data assimilation system over south-eastern South America
ATMOSPHERIC RESEARCH(2023)
摘要
Observations that are assimilated into numerical weather prediction systems are conformed by numerous data sets and their impact should be objectively evaluated. This can be efficiently achieved by the Forecast Sensitivity to Observation Impact (FSOI) methodology. In this study we explore the application of the ensemble formulation of FSOI (EFSOI) in a regional data assimilation system over south-eastern South America and evaluate the ob-servations that result beneficial or detrimental to the forecast. In this paper we focus on the impact of two types of surface weather stations: human-operated conventional surface weather station networks from the National Meteorological Service and non-conventional automatic surface weather stations from different public and private networks (CSWS and NSWS respectively). To achieve this, the Weather Research and Forecasting model coupled with the Local Ensemble Transform Kalman Filter is used with 20 members. The experiment was carried out during 30 days of the intensive observing period of the RELAMPAGO-CACTI (Remote sensing of Electrifi-cation, Lightning, And Mesoscale/microscale Processes with Adaptive Ground Observations-Clouds, Aerosols, and Complex Terrain Interaction) field campaign that was conducted during the 2018-2019 austral warm season in the center of Argentina. 20 km resolution analyses were obtained every 6-h, assimilating data from soundings, aircrafts, GOES derived motion winds, AIRS retrievals, CSWS and NSWS. It is shown that, considering the entire period, all the observation sources had a positive impact on the 6-h forecasts. However, when each variable is analyzed individually, surface pressure observations from both CSWS and NSWS show on average a negative impact. Two data denial experiments were carried out in order to support EFSOI results. This paper is the first approximation to quantify the impact of different observation sources and observed variables at a regional scale over south-eastern South America, a data sparse region where unconventional networks can help close large geographical data gaps. The results of this work help identify observation data sources detrimental for the data assimilation system, suggesting data selection criteria to improve the regional forecast accuracy.
更多查看译文
关键词
Data assimilation,Ensemble,Observation impact,Numerical weather prediction systems
AI 理解论文
溯源树
样例
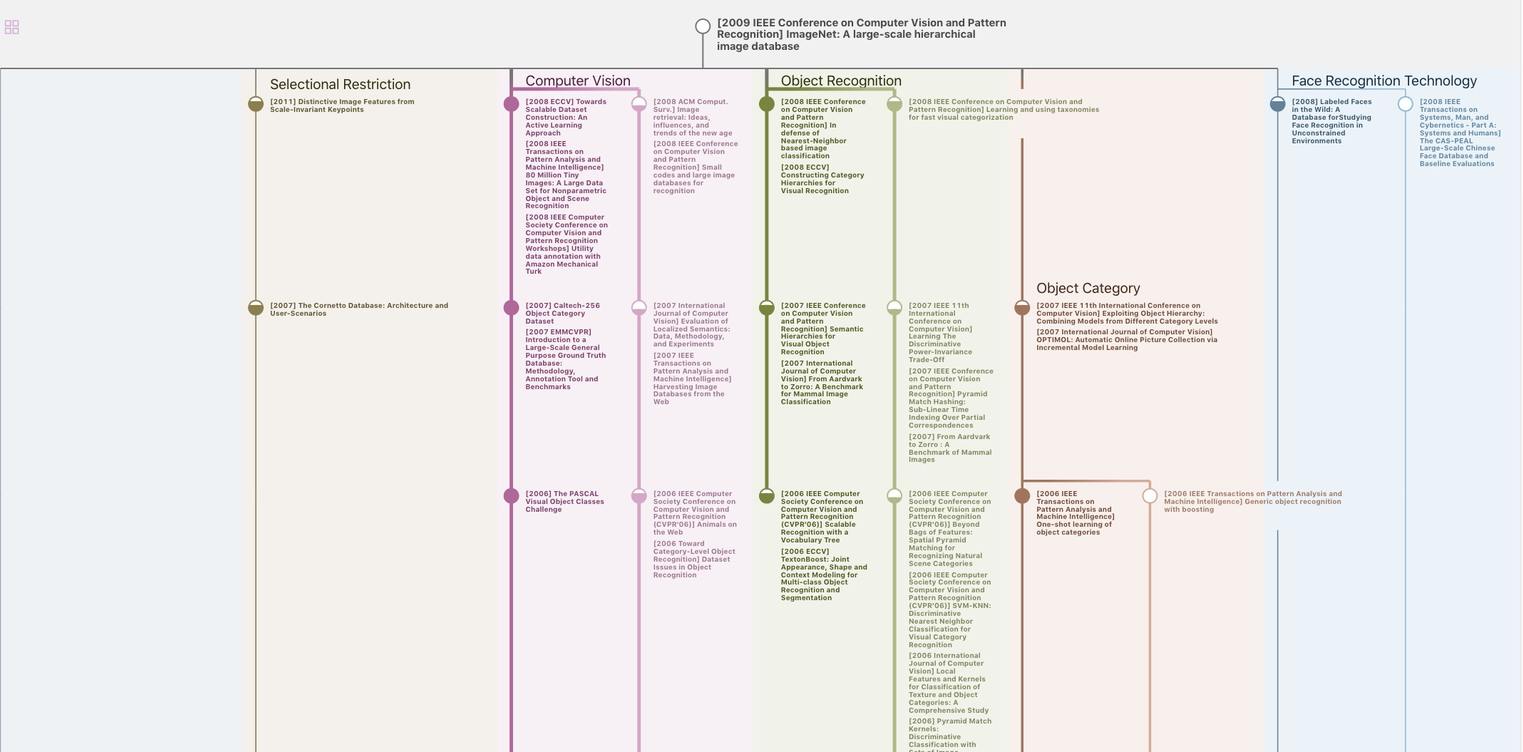
生成溯源树,研究论文发展脉络
Chat Paper
正在生成论文摘要