Intelligent identification of fragmented non-magnetic materials for end-of-life refrigerator recycling
JOURNAL OF THE AIR & WASTE MANAGEMENT ASSOCIATION(2024)
摘要
E-waste is a valuable secondary resource containing numerous toxic substances and high-value components. If improperly handled, it will cause severe environmental pollution. Therefore, efficient recycling of this material can reduce environmental pollution. However, after crushing, fine crushing, and magnetic separation, a substantial quantity of fragmented non-magnetic materials with high value, such as copper andg aluminum, remain. Refrigerators, as typical e-waste, have a similar composition to fragmented non-magnetic materials. Consequently, this paper focuses on the issues of low efficiency, environmental pollution, and resource waste in sorting fragmented non-magnetic materials from waste refrigerators. This paper constructs a data set of fragmented non-magnetic materials of refrigerators, augments the data set, and identifies fragmented non-magnetic materials of refrigerators using a computer vision-based deep learning method. In this study, YOLOv5s is used as the benchmark model. The CBAM module is added to the backbone to enable intelligent identification and sorting of fragmented non-magnetic materials in refrigerators. The final identification efficiency of waste refrigerators meets the requirements of industrial applications, with an accuracy rate of 98.3%, a recall rate of 96.8%, and an average accuracy of 98%. Based on the similarity of the composition of e-waste fragmented materials, this model sorting method can be applied to sorting additional e-waste fragmented materials. Furthermore, it provides the theoretical foundation for promoting e-waste resourcefulness.Implications: This paper proposes a recognition model based on YOLOv5s to solve the problems of low sorting efficiency, environmental pollution, harm to health, and resource waste of non-magnetic crushed material from refrigerators. The recognition model principally addresses the following issues: a deep learning model is developed for recognition and sorting to improve e-waste recognition and sorting efficiency. Concerning the issue of environmental benefits in an ecological environment, a vision-based automatic identification method is proposed to sort harmful waste, such as foam, to preserve the ecological environment. In response to the problem of resource waste, this project improves the purity of precious metals, resulting in a recovery rate of 99.1% for copper and 96.44% for aluminum. In other words, the cost of recovering metals has increased. The identification model of non-magnetic crushed material in refrigerators satisfies production identification and sorting requirements. In addition, the method has application and promotion value, sorting a theoretical foundation and method for identifying and classifying e-waste.
更多查看译文
AI 理解论文
溯源树
样例
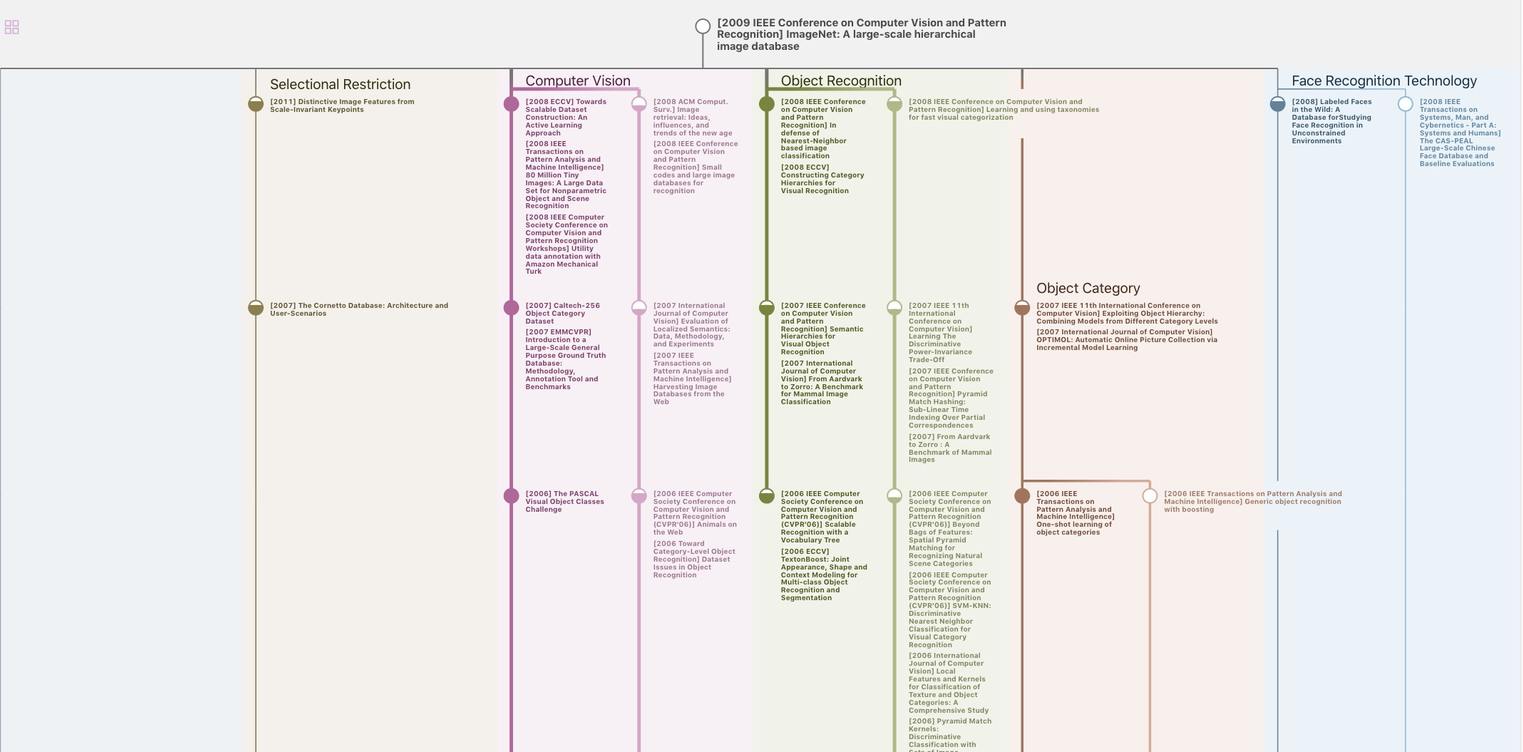
生成溯源树,研究论文发展脉络
Chat Paper
正在生成论文摘要