An interpretable ensemble learning model facilitates early risk stratification of ischemic stroke in intensive care unit: Development and external validation of ICU-ISPM.
Computers in biology and medicine(2023)
摘要
Ischemic stroke (IS) is a common and severe condition that requires intensive care unit (ICU) admission, with high mortality and variable prognosis. Accurate and reliable predictive tools that enable early risk stratification can facilitate interventions to improve patient outcomes; however, such tools are currently lacking. In this study, we developed and validated novel ensemble learning models based on soft voting and stacking methods to predict in-hospital mortality from IS in the ICU using two public databases: MIMIC-IV and eICU-CRD. Additionally, we identified the key predictors of mortality and developed a user-friendly online prediction tool for clinical use. The soft voting ensemble model, named ICU-ISPM, achieved an AUROC of 0.861 (95% CI: 0.829-0.892) and 0.844 (95% CI: 0.819-0.869) in the internal and external test cohorts, respectively. It significantly outperformed the APACHE scoring system and was more robust than individual models. ICU-ISPM obtained the highest performance compared to other models in similar studies. Using the SHAP method, the model was interpretable, revealing that GCS score, age, and intubation were the most important predictors of mortality. This model also provided a risk stratification system that can effectively distinguish between low-, medium-, and high-risk patients. Therefore, the ICU-ISPM is an accurate, reliable, interpretable, and clinically applicable tool, which is expected to assist clinicians in stratifying IS patients by the risk of mortality and rationally allocating medical resources. Based on ICU-ISPM, an online risk prediction tool was further developed, which was freely available at: http://ispm.idrblab.cn/.
更多查看译文
AI 理解论文
溯源树
样例
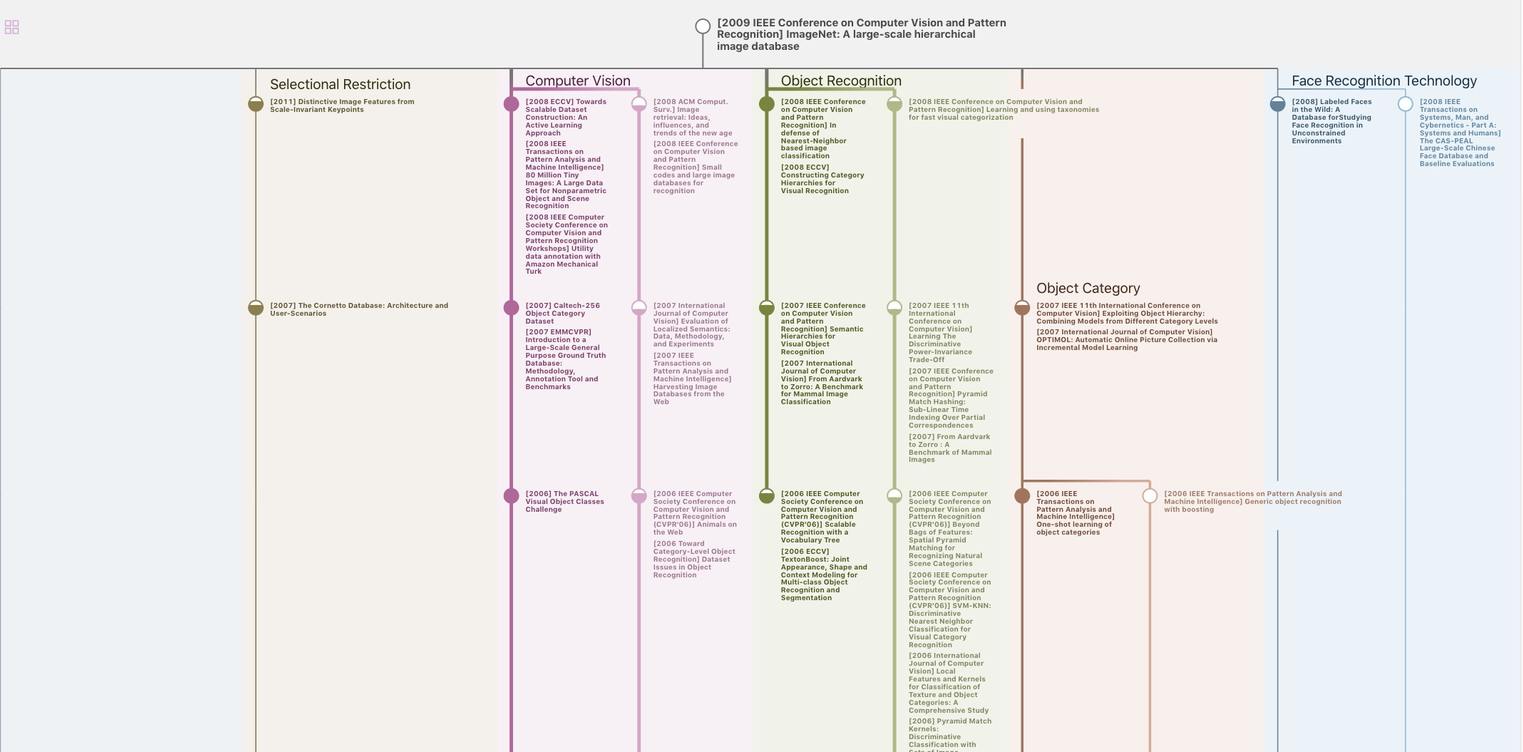
生成溯源树,研究论文发展脉络
Chat Paper
正在生成论文摘要