Detecting and Mitigating Algorithmic Bias in Binary Classification using Causal Modeling
CoRR(2023)
摘要
This paper proposes the use of causal modeling to detect and mitigate algorithmic bias. We provide a brief description of causal modeling and a general overview of our approach. We then use the Adult dataset, which is available for download from the UC Irvine Machine Learning Repository, to develop (1) a prediction model, which is treated as a black box, and (2) a causal model for bias mitigation. In this paper, we focus on gender bias and the problem of binary classification. We show that gender bias in the prediction model is statistically significant at the 0.05 level. We demonstrate the effectiveness of the causal model in mitigating gender bias by cross-validation. Furthermore, we show that the overall classification accuracy is improved slightly. Our novel approach is intuitive, easy-to-use, and can be implemented using existing statistical software tools such as "lavaan" in R. Hence, it enhances explainability and promotes trust.
更多查看译文
关键词
algorithmic bias,binary classification
AI 理解论文
溯源树
样例
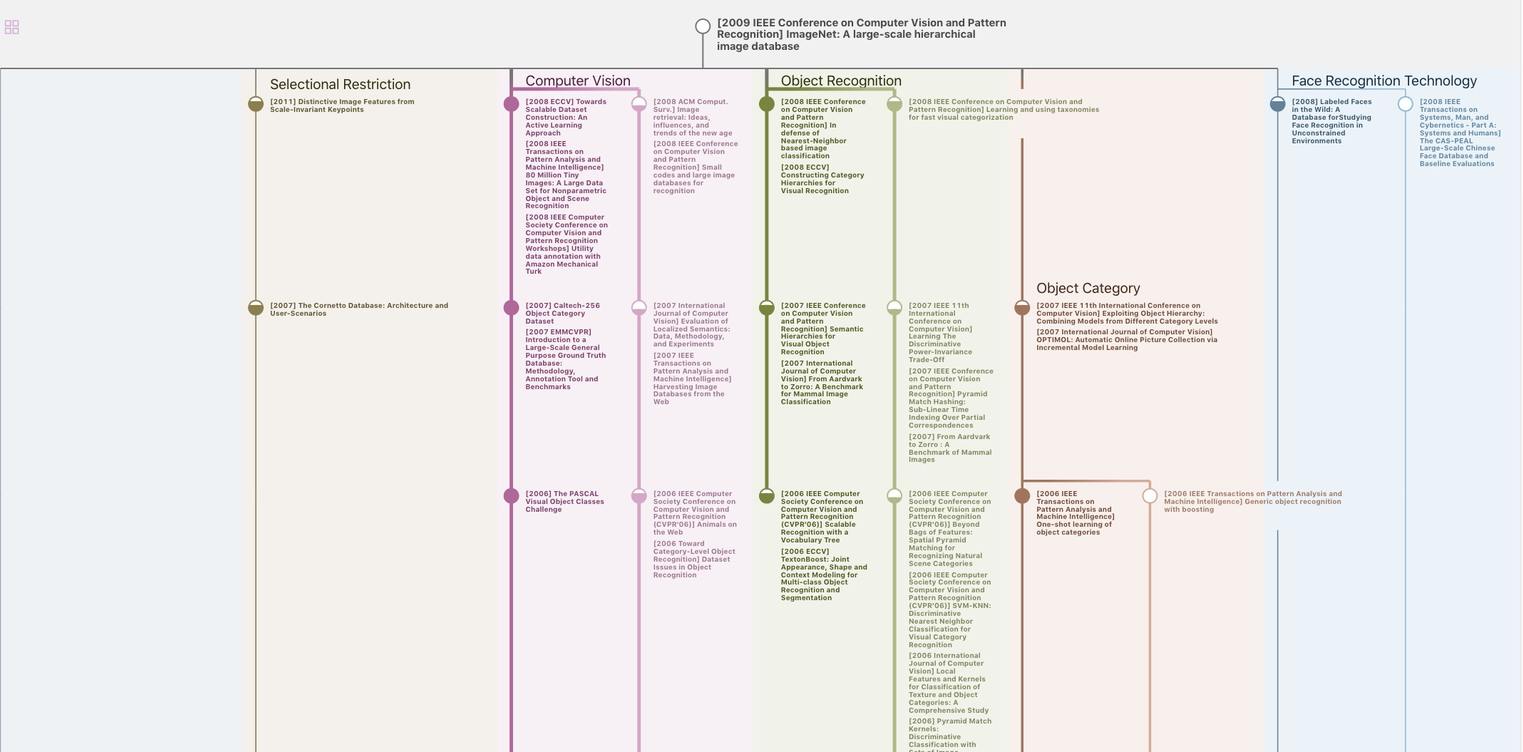
生成溯源树,研究论文发展脉络
Chat Paper
正在生成论文摘要